Open Access
ARTICLE
Hyperspectral Reflectance Imaging for Detecting Typical Defects of Durum Kernel Surface
a College of Life Information Science and Instrument Engineering, Hangzhou Dianzi University, Hangzhou, People’s Republic of China;
b School of Technology and Health, KTH Royal Institute of Technology, Stockholm, Sweden;
c Department of Economic Management, Cancer Affiliated Hospital of Xinjiang Medical University, Urumqi, China;
d College of Biosystems Engineering and Food Science, Zhejiang University, Hangzhou, People’s Republic of China
# Feng-Nong Chen and Pulan Chen are contributed equally to this study and share first authorship
* Corresponding Authors:Fang Cheng, ..cn; Feng-Nong, Chen
Intelligent Automation & Soft Computing 2018, 24(2), 351-358. https://doi.org/10.1080/10798587.2017.1293927
Abstract
In recent years, foodstuff quality has triggered tremendous interest and attention in our society as a series of food safety problems. The hyperspectral imaging techniques have been widely applied for foodstuff quality. In this study, we were undertaken to explore the possibility of unsound kernel detecting (Triticum durum Desf), which were defined as black germ kernels, moldy kernels and broken kernels, by selecting the best band in hyperspectral imaging system. The system possessed a wavelength in the range of 400 to 1,000 nm with neighboring bands 2.73 nm apart, acquiring images of bulk wheat samples from different wheat varieties. A series of technologies of hyperspectral imaging processing and spectral analysis were used to separate unsound kernels from sound kernels, including the Principal Component Analysis (PCA), the band ratio, the band difference and the best band. According to the selected bands, the best accuracy was 95.6, 96.7 and 98.5% for 710 black germ kernels, 627 break kernels and 1,169 healthy kernels,respectively. The result shows that the method based on the band selection was feasible.Abbreviations: CCD: Charge-coupled Device; PC: Personal Computer; PCA: Principal Component Analysis; PLSDA: Partial Least Lquares Discriminant Analysis; ANN: Artificial Neural Networks; SVM: Support Vector Machine
Keywords
Cite This Article
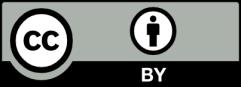