Guest Editors
Dr. Anas Bilal
Email: 910288@hainnu.edu.cn
Affiliation: College of Information Science and Technology, Hainan Normal University, Haikou 571158, China
Homepage:
Research Interests: AI, Computer vision, Image processing, medical imaging, Remote Sensing, and Pattern Recognition
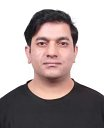
Dr. Muhammad Shafiq
Email: shafiqk786@hotmail.com
Affiliation: School of Information Engineering, Qujing Normal University, Qujing 655011, China
Homepage:
Research Interests: IoT, AI, ML, DL, Information Systems, Software Engineering, Requirements Engineering, Software Security, Offshore Software Development, Software Outsourcing, DevOps Security, Information Science & Technology
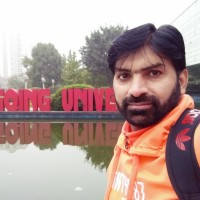
Dr. Zohaib Ahmed
Email: ahmedzohaib03@gmail.com
Affiliation: Department of Criminology & Forensic Sciences Technology, Lahore Garrison University, Lahore, Pakistan
Homepage:
Research Interests: understanding human behavior and the practical applications of psychological principles
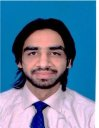
Summary
The field of generative artificial intelligence is transforming due to methods in soft computing and adaptive machine learning. Soft computing and adaptive machine learning are vital because they allow for continuing learning and adaptation to new data, boost performance progressively, provide flexibility in addressing uncertainty via methods like fuzzy logic and evolutionary algorithms, and stimulate generative algorithms' creative potential to produce more realistic and various results. These technologies can be integrated by using genetic algorithms for iterative model evolution, fuzzy logic can be used to handle uncertainty and approximate reasoning, reinforcement learning to improve models based on feedback from their environment, and adaptive neural networks that modify their parameters based on new data.
A number of methods and tools are used, including Generative Adversarial Networks (GANs) and Variational Autoencoders (VAEs) for producing high-quality images and data, Genetic Programming to evolve programs and optimize performance, and TensorFlow and PyTorch frameworks for developing adaptive and soft computing models. In this field, current trends include AI art and music produced by generative models, displaying high levels of creativity, models that customize in real time to new data for applications such as personalized recommendations and autonomous driving, and cross-disciplinary applications in healthcare for diagnosis and drug discovery. Future developments include hyper-personalization that will improve user experiences by providing customized features and content, autonomous systems that will learn and adapt continuously to make better decisions, and improved human-AI collaboration that leads to more logical and effective partnerships.
Despite its potential, it faces several obstacles like the demand for high-quality and various information to develop adaptive models, high computational complexity during training and adaptation, and ethical issues over AI-generated content and decision-making. The primary goal of efforts to address these issues consists of enhancing algorithm performance to reduce computational demands, guaranteeing that data management procedures have integrity to preserve data quality, and creating moral frameworks and standards to guide the ethical advancement and application of generative AI technologies. However, dynamic learning, flexibility, and increased creativity are being made possible by adaptive machine learning and soft computing techniques, which are transforming generative AI.
The topics of the issue include but not limited to the following:
• Integration of Soft Computing and Adaptive Machine Learning in Generative AI.
• Enhancing Model Learning and Adaptation in Generative AI through Reinforcement Learning.
• Ethical Considerations in the Development of Adaptive Generative AI Models.
• Applications of Adaptive Techniques in Cross-disciplinary Generative AI.
• Role of GANs and VAEs in Image and Data Generation.
• Employing Generative AI in Entertainment for Enhancing User Engagement and Experience.
• Analysis of Legal and Regulatory Challenges in Generative AI Deployment.
• Future of Generative AI in Space Exploration and Science.
• Ensuring Data Integrity in Generative AI for Robust Data Management Practices.
• Strategies for Overcoming Obstacles in Generative AI.
Keywords
Generative AI., Soft Computing, Adaptive Machine Learning, Reinforcement Learning, Generative AI Model