Open Access
ARTICLE
Secure Digital Image Watermarking Technique Based on ResNet-50 Architecture
1 Department of Computer Science and Engineering, GIET University, Gunupur, Odisha, 765022, India
2 Department of Computer Science and Applications, Utkal University, Bhubaneswar, Odisha, 751004, India
* Corresponding Authors: Satya Narayan Das. Email: ; Mrutyunjaya Panda. Email:
Intelligent Automation & Soft Computing 2024, 39(6), 1073-1100. https://doi.org/10.32604/iasc.2024.057013
Received 05 August 2024; Accepted 18 October 2024; Issue published 30 December 2024
Abstract
In today’s world of massive data and interconnected networks, it’s crucial to burgeon a secure and efficient digital watermarking method to protect the copyrights of digital content. Existing research primarily focuses on deep learning-based approaches to improve the quality of watermarked images, but they have some flaws. To overcome this, the deep learning digital image watermarking model with highly secure algorithms is proposed to secure the digital image. Recently, quantum logistic maps, which combine the concept of quantum computing with traditional techniques, have been considered a niche and promising area of research that has attracted researchers’ attention to further research in digital watermarking. This research uses the chaotic behaviour of the quantum logistic map with Rivest–Shamir–Adleman (RSA) and Secure Hash (SHA-3) algorithms for a robust watermark embedding process, where a watermark is embedded into the host image. This way, the quantum chaos method not only helps limit the chance of tampering with the image content through reverse engineering but also assists in maintaining a high level of imperceptibility and strong robustness with efficient extraction or detection of watermark images. Lifting Wavelet Transformation (LWT) is a potential and computationally efficient version of traditional Discrete Wavelet Transform (DWT) where the host image is divided into four sub-bands to offer a multi-resolution view of an image with greater flexibility in watermarking methodologies. Furthermore, considering the robustness against attacks, a pre-trained Residual Neural Network (ResNet-50), a convolutional neural network with 50 layers deep, is used to better learn the complex features and efficiently extract the watermark from the image. By integrating RSA and SHA-3 algorithms, the proposed model demonstrates improved imperceptibility, robustness, and accuracy in watermark extraction compared to traditional methods. It achieves a Peak Signal-to-Noise Ratio (PSNR) of 49.83%, a Structural Similarity Index Measure (SSIM) of 0.98, and a Number of Pixels Change Rate (NPCR) of 99.79%, respectively. These results reflect the model’s effectiveness in delivering superior quality and security. Consequently, our proposed approach offers accurate results, exceptional invisibility, and enhanced robustness compared to the existing digital image watermarking techniques.Keywords
Cite This Article
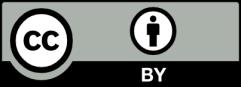
This work is licensed under a Creative Commons Attribution 4.0 International License , which permits unrestricted use, distribution, and reproduction in any medium, provided the original work is properly cited.