Open Access
ARTICLE
Extended Deep Learning Algorithm for Improved Brain Tumor Diagnosis System
1 Department of Computer Engineering, Government Polytechnic College, Sevvapet, Tiruvallur, Tamil Nadu, 602025, India
2 Department of Computer Science and Engineering, KG Reddy College of Engineering and Technology, Hyderabad, Telangana, 500075, India
3 Department of Computer Science and Engineering, Sri Venkateswara College of Engineering, Sriperumbudur, Chennai, 602117, India
4 Department of Computer Science and Engineering, Annamalai University, Chidambaram, Tamil Nadu, 608002, India
5 Department of Computer Science and Engineering, Aarupadai Veedu Institute of Technology, Vinayaka Mission Research Foundation, Paiyanur, Tamil Nadu, 603104, India
6 Department of Electrical and Electronics Engineering, Sri Venkateswara College of Engineering, Sriperumbudur, 602117, India
7 Department of Computer Science, College of Computer and Information Sciences, Majmaah University, Al-Majmaah, 11952, Saudi Arabia
8 Department of Mathematics, College of Science Al-Zulfi, Majmaah University, Al-Majmaah, 11952, Saudi Arabia
9 Electrical Engineering Department, Faculty of Engineering & Technology, Future University in Egypt, New Cairo, 11835, Egypt
10 Department of Computer Science, College of Computing, Khon Kaen University, Khon Kaen, 40002, Thailand
* Corresponding Author: Mohd Anul Haq. Email:
Intelligent Automation & Soft Computing 2024, 39(1), 33-55. https://doi.org/10.32604/iasc.2024.039009
Received 07 January 2023; Accepted 10 March 2023; Issue published 29 March 2024
Abstract
At present, the prediction of brain tumors is performed using Machine Learning (ML) and Deep Learning (DL) algorithms. Although various ML and DL algorithms are adapted to predict brain tumors to some range, some concerns still need enhancement, particularly accuracy, sensitivity, false positive and false negative, to improve the brain tumor prediction system symmetrically. Therefore, this work proposed an Extended Deep Learning Algorithm (EDLA) to measure performance parameters such as accuracy, sensitivity, and false positive and false negative rates. In addition, these iterated measures were analyzed by comparing the EDLA method with the Convolutional Neural Network (CNN) way further using the SPSS tool, and respective graphical illustrations were shown. The results were that the mean performance measures for the proposed EDLA algorithm were calculated, and those measured were accuracy (97.665%), sensitivity (97.939%), false positive (3.012%), and false negative (3.182%) for ten iterations. Whereas in the case of the CNN, the algorithm means accuracy gained was 94.287%, mean sensitivity 95.612%, mean false positive 5.328%, and mean false negative 4.756%. These results show that the proposed EDLA method has outperformed existing algorithms, including CNN, and ensures symmetrically improved parameters. Thus EDLA algorithm introduces novelty concerning its performance and particular activation function. This proposed method will be utilized effectively in brain tumor detection in a precise and accurate manner. This algorithm would apply to brain tumor diagnosis and be involved in various medical diagnoses after modification. If the quantity of dataset records is enormous, then the method’s computation power has to be updated.Keywords
Cite This Article
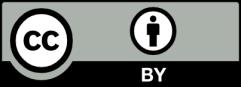
This work is licensed under a Creative Commons Attribution 4.0 International License , which permits unrestricted use, distribution, and reproduction in any medium, provided the original work is properly cited.