Open Access
ARTICLE
ABMRF: An Ensemble Model for Author Profiling Based on Stylistic Features Using Roman Urdu
1 Department of Computer Science, City University of Science and Information Technology, Peshawar, Pakistan
2 Department of Computer Science, School of Engineering and Digital Sciences, Nazarbayev University, Astana, Kazakhstan
3 Department of Computer Science, College of Computer, Qassim University, Buraydah, Saudi Arabia
4 Department of Information Technology, College of Computer, Qassim University, Buraydah, Saudi Arabia
* Corresponding Author: Ali Mustafa Qamar. Email:
Intelligent Automation & Soft Computing 2024, 39(2), 301-317. https://doi.org/10.32604/iasc.2024.045402
Received 25 August 2023; Accepted 27 December 2023; Issue published 21 May 2024
Abstract
This study explores the area of Author Profiling (AP) and its importance in several industries, including forensics, security, marketing, and education. A key component of AP is the extraction of useful information from text, with an emphasis on the writers’ ages and genders. To improve the accuracy of AP tasks, the study develops an ensemble model dubbed ABMRF that combines AdaBoostM1 (ABM1) and Random Forest (RF). The work uses an extensive technique that involves text message dataset pretreatment, model training, and assessment. To evaluate the effectiveness of several machine learning (ML) algorithms in classifying age and gender, including Composite Hypercube on Random Projection (CHIRP), Decision Trees (J48), Naïve Bayes (NB), K Nearest Neighbor, AdaboostM1, NB-Updatable, RF, and ABMRF, they are compared. The findings demonstrate that ABMRF regularly beats the competition, with a gender classification accuracy of 71.14% and an age classification accuracy of 54.29%, respectively. Additional metrics like precision, recall, F-measure, Matthews Correlation Coefficient (MCC), and accuracy support ABMRF’s outstanding performance in age and gender profiling tasks. This study demonstrates the usefulness of ABMRF as an ensemble model for author profiling and highlights its possible uses in marketing, law enforcement, and education. The results emphasize the effectiveness of ensemble approaches in enhancing author profiling task accuracy, particularly when it comes to age and gender identification.Keywords
Cite This Article
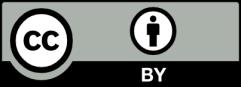
This work is licensed under a Creative Commons Attribution 4.0 International License , which permits unrestricted use, distribution, and reproduction in any medium, provided the original work is properly cited.