Open Access
ARTICLE
Air-Side Heat Transfer Performance Prediction for Microchannel Heat Exchangers Using Data-Driven Models with Dimensionless Numbers
1 Institute of Process Equipment, College of Energy Engineering, Zhejiang University, Hangzhou, 310027, China
2 Institute of Wenzhou, Zhejiang University, Wenzhou, 310027, China
3 School of Intelligent Manufacturing Ecosystem, Xi’an Jiaotong-Liverpool University, Suzhou, 215123, China
* Corresponding Author: Long Huang. Email:
(This article belongs to the Special Issue: Machine Learning and Data-Driven Techniques for Mass and Thermal Transport)
Frontiers in Heat and Mass Transfer 2024, 22(6), 1613-1643. https://doi.org/10.32604/fhmt.2024.058231
Received 07 September 2024; Accepted 16 October 2024; Issue published 19 December 2024
Abstract
This study explores the effectiveness of machine learning models in predicting the air-side performance of microchannel heat exchangers. The data were generated by experimentally validated Computational Fluid Dynamics (CFD) simulations of air-to-water microchannel heat exchangers. A distinctive aspect of this research is the comparative analysis of four diverse machine learning algorithms: Artificial Neural Networks (ANN), Support Vector Machines (SVM), Random Forest (RF), and Gaussian Process Regression (GPR). These models are adeptly applied to predict air-side heat transfer performance with high precision, with ANN and GPR exhibiting notably superior accuracy. Additionally, this research further delves into the influence of both geometric and operational parameters—including louvered angle, fin height, fin spacing, air inlet temperature, velocity, and tube temperature—on model performance. Moreover, it innovatively incorporates dimensionless numbers such as aspect ratio, fin height-to-spacing ratio, Reynolds number, Nusselt number, normalized air inlet temperature, temperature difference, and louvered angle into the input variables. This strategic inclusion significantly refines the predictive capabilities of the models by establishing a robust analytical framework supported by the CFD-generated database. The results show the enhanced prediction accuracy achieved by integrating dimensionless numbers, highlighting the effectiveness of data-driven approaches in precisely forecasting heat exchanger performance. This advancement is pivotal for the geometric optimization of heat exchangers, illustrating the considerable potential of integrating sophisticated modeling techniques with traditional engineering metrics.Keywords
Cite This Article
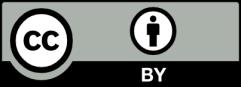
This work is licensed under a Creative Commons Attribution 4.0 International License , which permits unrestricted use, distribution, and reproduction in any medium, provided the original work is properly cited.