Open Access
ARTICLE
Artificial Intelligence-Driven FVM-ANN Model for Entropy Analysis of MHD Natural Bioconvection in Nanofluid-Filled Porous Cavities
1 Department of Mathematical Sciences, College of Science, Princess Nourah bint Abdulrahman University, Riyadh, 11671, Saudi Arabia
2 Mathematics Department, Faculty of Sciences, Assiut University, Assiut, 71515, Egypt
3 Department of Mathematics, College of Science, King Khalid University, Abha, 62529, Saudi Arabia
4 Basic Science, Faculty of Engineering, The British University in Egypt, Al-Shorouk City, Cairo, 11837, Egypt
5 Instituto de Ciencias Matemáticas ICMAT, CSIC, UAM, UCM, UC3M, Madrid, 28049, Spain
* Corresponding Author: Sara I. Abdelsalam. Email:
(This article belongs to the Special Issue: Advances in Computational Thermo-Fluids and Nanofluids)
Frontiers in Heat and Mass Transfer 2024, 22(5), 1277-1307. https://doi.org/10.32604/fhmt.2024.056087
Received 14 July 2024; Accepted 25 September 2024; Issue published 30 October 2024
Abstract
The research examines fluid behavior in a porous box-shaped enclosure. The fluid contains nanoscale particles and swimming microbes and is subject to magnetic forces at an angle. Natural circulation driven by biological factors is investigated. The analysis combines a traditional numerical approach with machine learning techniques. Mathematical equations describing the system are transformed into a dimensionless form and then solved using computational methods. The artificial neural network (ANN) model, trained with the Levenberg-Marquardt method, accurately predicts values, showing high correlation (R = 1), low mean squared error (MSE), and minimal error clustering. Parametric analysis reveals significant effects of parameters, length and location of source (B), (D), heat generation/absorption coefficient (Q), and porosity parameter (ε). Increasing the cooling area length (B) reduces streamline intensity and local Nusselt and Sherwood numbers, while decreasing isotherms, iso-concentrations, and micro-rotation. The Bejan number (Be+) decreases with increasing (B), whereas (Be+++), and global entropy (e+++) increase. Variations in (Q) slightly affect streamlines but reduce isotherm intensity and average Nusselt numbers. Higher (D) significantly impacts isotherms, iso-concentrations, and micro-rotation, altering streamline contours and local Bejan number distribution. Increased (ε) enhances streamline strength and local Nusselt number profiles but has mixed effects on average Nusselt numbers. These findings highlight the complex interactions between cooling area length, fluid flow, and heat transfer properties. By combining finite volume method (FVM) with machine learning technique, this study provides valuable insights into the complex interactions between key parameters and heat transfer, contributing to the development of more efficient designs in applications such as cooling systems, energy storage, and bioengineering.Keywords
Cite This Article
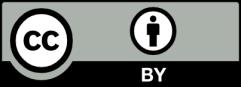
This work is licensed under a Creative Commons Attribution 4.0 International License , which permits unrestricted use, distribution, and reproduction in any medium, provided the original work is properly cited.