Open Access
ARTICLE
Optimizing Two-Phase Flow Heat Transfer: DCS Hybrid Modeling and Automation in Coal-Fired Power Plant Boilers
1 Technical Supervision Center, Huadian Electric Power Research Institute Co., Ltd., Hangzhou, 310030, China
2 School of Mechanical Engineering, Southwest Jiaotong University, Chengdu, 611756, China
3 School of Management Science & Real Estate, Chongqing University, Chongqing, 400044, China
* Corresponding Authors: Caijiang Lu. Email: ; Pan Shi. Email:
(This article belongs to the Special Issue: Two-phase flow heat and mass transfer in advanced energy systems)
Frontiers in Heat and Mass Transfer 2024, 22(2), 615-631. https://doi.org/10.32604/fhmt.2024.048333
Received 05 December 2023; Accepted 18 January 2024; Issue published 20 May 2024
Abstract
In response to escalating challenges in energy conservation and emission reduction, this study delves into the complexities of heat transfer in two-phase flows and adjustments to combustion processes within coal-fired boilers. Utilizing a fusion of hybrid modeling and automation technologies, we develop soft measurement models for key combustion parameters, such as the net calorific value of coal, flue gas oxygen content, and fly ash carbon content, within the Distributed Control System (DCS). Validated with performance test data, these models exhibit controlled root mean square error (RMSE) and maximum absolute error (MAXE) values, both within the range of 0.203. Integrated into their respective automatic control systems, these models optimize two-phase flow heat transfer, fine-tune combustion conditions, and mitigate incomplete combustion. Furthermore, this paper conducts an in-depth exploration of the generation mechanism of nitrogen oxides (NO) and low oxygen emission reduction technology in coal-fired boilers, demonstrating a substantial reduction in furnace exit NO generation by 30% to 40% and the power supply coal consumption decreased by 1.62 g/(kW h). The research outcomes highlight the model’s rapid responsiveness, enabling prompt reflection of transient variations in various economic indicator parameters. This provides a more effective means for real-time monitoring of crucial variables in coal-fired boilers and facilitates timely combustion adjustments, underscoring notable achievements in boiler combustion. The research not only provides valuable and practical insights into the intricacies of two-phase flow heat transfer and heat exchange but also establishes a pioneering methodology for tackling industry challenges.Graphic Abstract
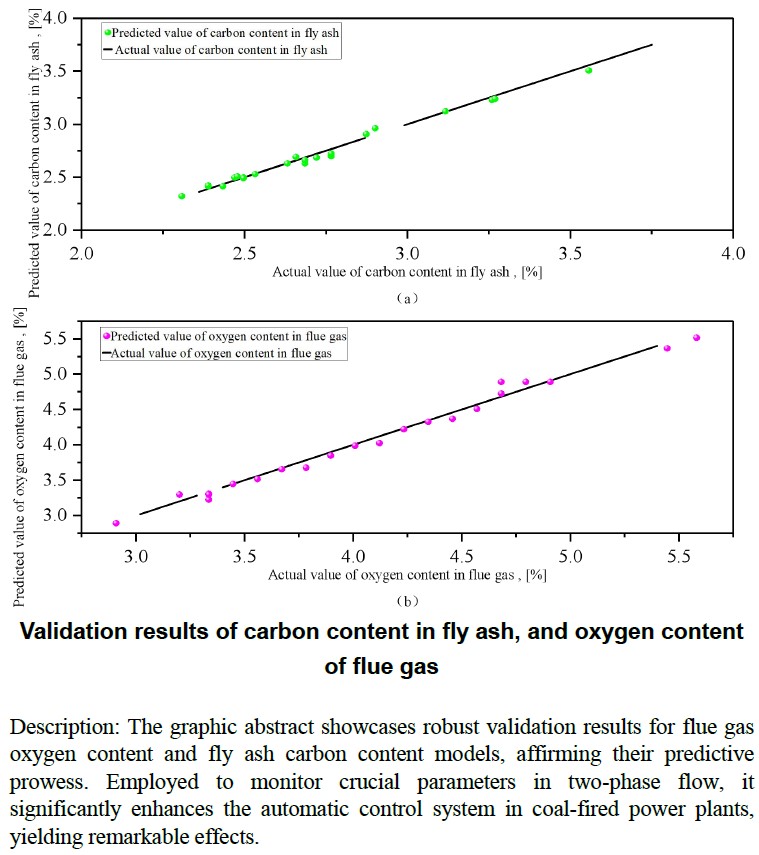
Keywords
With escalating global energy demand and a thrust towards green energy policies, coal-fired power plants face the dual challenges of cost reduction and emission reduction. Of particular concern is the optimization of high-efficiency, low-pollution combustion technologies. The reduction of nitrogen oxides (NOx) is especially critical, as its generation is closely related to furnace temperature [1] and excess air, which is interconnected with two-phase flow heat transfer. Precise measurements of flue gas oxygen content, automatic regulation of air flow and oxygen content, and the use of staged combustion [2,3] can effectively reduce NOx emissions. To achieve the energy efficiency optimization of coal-fired power plants, an in-depth understanding of the fluid phase transition processes inside the boiler and their interrelations with key combustion parameters is crucial. For example, the work of Stupar et al. [4] defined radiating reactive two-phase turbulent flow in a steam boiler and analyzed the impact of parameters on the steam boiler operation with an accepted concept of primary measures.
The net calorific value of coal significantly influences combustion heat release and transfer efficiency. High-calorific coal demands meticulous two-phase flow control for complete combustion, while low-calorific coal necessitates adjustments for extended coal powder residence time, augmenting combustion efficiency and reducing fly ash carbon content. The oxygen content of flue gas is pivotal for coal combustion, affecting heat transfer efficiency, and its poor control may lead to incomplete combustion. In the two-phase flow heat transfer process, fluid phase change may influence the flow and mixing of flue gas, altering the oxygen demand during combustion. By optimizing two-phase flow heat transfer, combustion conditions can be adjusted to reduce incomplete combustion, thereby affecting the concentration of oxygen in the flue gas. Zhang et al. [5] conducted a numerical simulation, revealing the impact of direct coal smoke in hot flue gas furnaces on temperature, velocity, pressure, and the CH4, CO, O2 and CO2 content distribution. Furnace exit NOx concentration is a key environmental indicator, influencing denitrification equipment and heat transfer efficiency. Fluid phase change alters NOx generation paths, as demonstrated by Zhan et al.’s research on pulverized-coal nozzle flow deflection angles [6]. Carbon content in fly ash reflects combustion’s economic impact. Two-phase flow heat transfer affects coal powder combustion paths and residence time, influencing fly ash formation.
The aforementioned studies highlight the multidimensional and highly nonlinear correlation between two-phase flow heat transfer processes and boiler operational parameters [7]. It is difficult to establish a comprehensive theoretical model to calculate key combustion parameters and efficiency at this stage of development. Therefore, traditional methods for optimizing boiler combustion primarily rely on experiments and plant trails, e.g., direct measurement of parameters like excess air to assess the impact on heat loss and emissions [8], conducting cold airflow experiment [9], and retrofitting the boiler [10], or empirical knowledge facilitated with a type of control system mainly aimed at improving the load-following capability [11], stability of the steam pressure [12] and load adaptation of boiler-turbines [13]. While these methods are useful to varying degrees for industrial process operations, they exhibit limitations across various types. For instance, the experiments, either lab scale or plan tests, can’t cover all the possible operating conditions and the restrictions of the control system, leading to difficulties in optimizing boiler combustion. Additionally, many power plants still rely on trial adjustments and personal experience for boiler operation. Strict adherence to conventional operating procedures for combustion optimization introduces errors [14], lacking real-time monitoring and automatic control, hindering operational efficiency improvements.
To address the complexity of actual boiler combustion and heat transfer processes, various feasible simplified models have been proposed, focusing on exploring the impact of one or a few variables [15–18]. Bing et al. used range analysis to determine the quantitative influence of five variables, unit load, namely coal property, the oxygen concentration, the second layer separated over-fire air (SOFA) opening degree and the 3/4 layer SOFA opening degree, on boiler efficiency and furnace exit NOx concentration [19]. However, in practical boiler operation, factors involved in combustion dynamically change and interact, making it challenging for the above models to monitor the two-phase flow heat transfer process in real time, thus achieving the desired optimization effect. More critically, in actual boilers, real-time and accurate measurement of key combustion parameters is extremely difficult. For example, due to the immaturity of equipment for real-time measurement of the net calorific value of coal, economic combustion adaptability to coal quality changes. The conflict between efficient and low-nitrogen combustion technologies is evident in flue gas oxygen content control [20]. Precise control can reduce NOx emissions and enhance economic combustion but encounters challenges due to unrepresentative measurements and the costly nature of zirconia sensors. Lee et al. [21] used dynamic matrix control (DMC) for supplementary control of air-fuel ratio in power plant emissions, but inaccurate oxygen measurements prevented the widespread application of this control method. Measurement devices for fly ash carbon content face accuracy issues, high maintenance costs, and high failure rates, hindering real-time optimization.
With the rapid development of computer technology, despite some achievements in modeling boiler combustion characteristics using machine learning algorithms such as neural networks and support vector machines [22–26], there are currently three challenges. First, seamless integration with the Distributed Control System (DCS) of power plants remains an ongoing challenge for these algorithms, hindering the automatic control of parameters and the achievement of optimal combustion results. Second, these algorithms heavily depend on hardware measurements, such as flue gas oxygen content and fly ash carbon content, as input variables. The accuracy of these initial input variables is insufficient, limiting the accuracy of predictions. Third, these algorithms, such as support vector machines, are known to have limitations in large-sample predictions and struggle to make accurate online predictions based on extensive power plant data. This paper concentrates on two 600 MW supercritical, front-rear wall tangentially fired pulverized coal system units as the research objects. DCS computational technology is harnessed for hybrid modeling, integrating boiler combustion mechanisms, mathematical statistics, factor analysis, and experimental modeling. In comparison to other methods with exorbitant costs and complexity [27,28], this approach operates without algorithmic constraints, requiring no new measurement points, additional equipment, or investments. In comparison to existing combustion optimization technologies, such as the retrofitting of low nitrogen combustion burners conducted by Li et al. [19], which incurred comprehensive costs reaching several million RMB through field test and optimization adjustment, resulting in a reduction of the power supply coal consumption by 1.4 g/(kW h), the technology proposed in this paper foregoes substantial investments in equipment and model algorithms. The cost is nearly zero, contributing to real-time optimization of key combustion parameters through computational soft measurements. The overarching goal of this technology is to enhance two-phase flow heat transfer efficiency, realizing efficient and environmentally friendly combustion processes. As a result, the power supply coal consumption ultimately decreased by 1.62 g/(kW h) and reduced furnace exit NOx generation by 30% to 40%. This study makes a significant contribution by effectively addressing challenges related to challenging-to-measure parameters through the integration of hybrid modeling and automation technologies within the DCS. Therefore, this research not only provides valuable and practical insights into the nuances of two-phase flow heat transfer and heat exchange but also lays the foundation for the intelligent advancement of future power plants, positioning itself as a crucial resource that can serve as initial parameters for a series of artificial intelligence algorithm models.
2.1 Soft Measurement Calculation Models for the Net Calorific Value of Coal and Oxygen Content of Flue Gas
The calculation of flue gas oxygen content needs to take into account the net calorific value of coal [29]. For subcritical drum boilers, the direct energy balance (DEB) method is used to estimate the net calorific value of coal. In contrast, for supercritical boilers, we use a specific coal consumption coefficient method [30]. This coefficient, denoted as K, incorporates the principle of energy conservation, as expressed in formula (1), which enables us to estimate the net calorific value of coal:
where:
K: K is usually confined to a range of 0.7 to 1.3.
B: Represents the actual total fuel consumption entering the furnace (primarily the total coal consumption, including oil consumption converted to coal consumption during oil gun use).
P: Represents actual power generation load.
P0: Corresponds to various load points under the design coal type.
B0: Corresponds to the fuel quantity at each load point under the design coal type.
Special cases like Run Back (RB) and high-pressure heater exit handling are also considered.
For direct-fired system, an approach simulating several minutes of coal feeding is used to calculate the actual total fuel consumption B and the actual load P. The total coal feed signal undergoes transmission through a series of third-order and first-order inertia links to obtain the corresponding boiler steam generation capacity. This method provides greater accuracy compared to algorithms utilizing averaged actual loads and fuel consumption values over a few minutes, as it better reflects the characteristics of the controlled object. The time constant t of the inertia link is obtained through on-site simulation experiments, and its transfer function is shown as formula (2):
Using the transfer function above, the net calorific value of coal are given by the following expression:
where:
Q: Represents the net calorific value of coal.
Qs: Represents the net calorific value of the design coal.
K(t): Signifies the coal consumption ratio coefficient value after a time constant t.
As shown in Fig. 1, the coal consumption ratio coefficient is established in DCS, and the calculation result is crucial for accurately calculating flue gas oxygen content.
Figure 1: Trend of the net calorific value soft measurement of coal
Subsequently, guided by the basic principles of boiler combustion, the coal combustion inside the furnace occurs at high temperatures. Under these conditions, the combustion of combustible substances and oxygen from the air takes place via high-temperature exothermic chemical reactions. The formula for calculating the oxygen concentration is given [31]:
where:
Qv: Represents the air flow into the furnace (adjusted using the DCS-calculated value of secondary air density and considering leakage coefficient, corrected using the air preheater’s front and rear differential pressure values under corresponding conditions).
B: Represents the total fuel consumption into the furnace.
Qr, QFT: Respectively represent the theoretical air required for complete combustion of 1 kg of entering coal and the theoretical flue gas volume (adjusted using the DCS-calculated flue gas density), with units of m3/kg.
The formula for calculating air density is shown as formula (5):
where:
ρ, p, T: Respectively represent the density, pressure, and thermodynamic temperature of dry air under other states.
ρ0, p0, T0: Represent the density, pressure, and thermodynamic temperature of dry air under standard conditions. They are measured in units of kg/m3, kPa, and K.
For standard conditions (T0 = 273 K and P0 = 101.3 kPa), ρ0 represents the density of dry air under normal composition (ρ0 = 1.293 kg/m3).
p: Represents atmospheric pressure, which is transmitted to DCS via transmitters installed on-site to measure atmospheric pressure.
T: Represents the average air temperature at the inlet of forced draft fans A and B, i.e., tif.
The calculation formulas for Qr and QFT are given by:
Through formulas (6) and (7), it is evident that despite the relatively simple combustion mechanism of the boiler, real-time calculations are challenging due to the necessity of promptly knowing the reference content of various elements. However, as ultimate analysis of coal is intricate and entails lag, coal-fired power plants generally perform proximate analysis, and DCS lacks real-time measurement data for various elements. Through extensive analysis of coal properties, we discern that coal primarily consists of combustible elements such as carbon, hydrogen, and sulfur. Under complete combustion conditions, these elements generate CO2, SO2, and H2O. According to the relevant literature, the air required for the combustion of 1 kg of coal per unit mass shows an approximately linear relationship with the net calorific value of coal. Therefore, in the absence of fuel element analysis data, the net calorific value of coal can be used as an approximation to calculate the theoretical air quantity [32]:
where:
Q: Represents the net calorific value of coal, measured in units of kJ/kg.
Finally, leveraging the real-time net calorific value of coal obtained through formula (3) in DCS, combined with the results of formulas (8) and (9), we calculate the theoretical air quantity Qr and the theoretical flue gas volume QFT required for the complete combustion of 1 kg of entering coal and subsequently obtain the oxygen concentration through formula (4).
In order to verify the soft-sensing of flue gas oxygen content, we utilized actual operational data for verification, Fig. 2 shows three aspects were examined. The calculated results from the established soft-sensing model were highly accurate and could replace physical sensors. The soft-sensing model shows a remarkable improvement in the accuracy of measuring oxygen content, especially at low loads, proving more reliable than the measurements from physical sensors. The soft-sensing results provided effective redundant information for physical sensors in cases of hardware failures or malfunctions, thus improving measurement reliability across various operational conditions.
Figure 2: Trend of soft-sensing values of flue gas oxygen content
2.2 Soft Measurement Calculation Model for Carbon Content in Fly Ash
Carbon content in fly ash is affected by various factors, making it challenging to model through mechanism analysis. As a result, factor analysis is employed in this paper to select key indicators or factors from numerous variables, forming the basis of the fly ash carbon content model. Then, the model is refined using experimental data.
Firstly, factor analysis is applied to obtain the maximum variance of the factor component matrix by orthogonal rotation, as per formula (10), and the factor model for carbon content in fly ash Cfh is established:
In formula (10), F1 represents the factor of hot air temperature, F2 stands for the factor of burnout, F3 corresponds to the factor of primary air pressure, and F4 represents the coal quality factor. Therefore, the main influencing factors on fly ash carbon content can be divided into: the hot air temperature factor (primary air temperature T1 and secondary air temperature T2), the burnout factor (excess air coefficient and coal fineness R90), the primary air pressure factor, and the coal quality factor. In this paper, T1 represents the outlet temperature of pulverizers, T2 signifies the average temperature at the outlet of air preheaters A and B, the excess air coefficient can be obtained from the calculation formula of the oxygen content Φ(O2) in the flue gas of furnace exit (soft-sensing values) and the oxygen content Φ’(O2) at the inlet of the air preheater, and R90 represents the particle size distribution of coal particles, which can be modeled by soft-sensing using primary air pressure PA and pulverizers separator frequency FE.
Subsequently, the coal quality factor is primarily determined by the contents of the coal’s total moisture M, inherent ash A, volatile matter V, and total sulfur S. The influence weights of these parameters on fly ash carbon content, as well as the variations in fly ash carbon content due to changes in coal quality under specific conditions, can be calculated using the boiler design residence time [33]. This important data requires online data to ensure its real-time availability. For instance, A can be derived through a linear relationship function corresponding to the net calorific value of coal calculated earlier (Q); S can be derived through a linear relationship function corresponding to the furnace exit SO2 concentration measurement value; M can be obtained by considering energy balance principles and soft-sensing modeling based on variables such as pulverizers air flow rate Vm, pulverizers load Bm, pulverizers inlet temperature Tin, pulverizers outlet temperature Tout, and coal fineness R90 [34]. The above data can also be set as default values based on manual assay values. However, if there are no new manual assay values input within 4 h, the system will automatically switch back to online values. V can be input manually based on the coal type or proximate analysis.
Finally, in order to refine the factor model, individual variable experiments were conducted, and actual test data were collected (including offline assay data for fly ash carbon content). From these data, curves depicting the numerical variations of fly ash carbon content due to individual variable influences were derived. These curves were then corrected using the least squares method. Through several rounds of experimental data correction, a relatively accurate factor model for fly ash carbon content was established. The model output results are rapid and highly responsive to instantaneous variations in various economic indicator parameters, exhibiting strong correlations with other boiler parameters.
3 Experimental Methods and Model Validation
3.1 Data Collection and Preprocessing
In this paper, data were collected for parameters such as oxygen content of flue gas and carbon content in fly ash using soft measurement methods on Boiler 1. After identifying steady state operating conditions using system discrimination, data were collected at 10-min intervals.
In addition, in order to ensure data quality, the 3σ principle was used to eliminate outliers. Data normalization was performed using formula (11), which limited input and output values within the range of [−1, 1].
where:
x, x′: Represent the original and normalized values, respectively.
3.2 Models Testing and Analysis
To validate the flue gas oxygen content soft measurement model, we employed an oxygen grid method. In this method, three high-precision zirconia sensors were strategically positioned on each side of furnace exit, denoted as A1, A2, and A3 for the A side, and B1, B2, and B3 for the B side. By collecting data from these sensors at different positions and varying insertion depths, followed by computing the average of all recorded values, we obtained the precise actual oxygen content within the flue gas.
Subsequently, the model was trained and tested under different loads, and the predicted values were compared with actual measurements, as shown in Table 1.
To validate the fly ash carbon content soft measurement model, we conducted model training using sample data collected at different loads, specifically focusing on the data points surrounding offline assay sampling moments.
Fig. 3 displays the validation results for oxygen content in flue gas and carbon content in fly ash, while Table 2 presents accuracy calculations, indicating high level of predictive capability for both models.
Figure 3: Validation results of carbon content in fly ash, and oxygen content in flue gas
4.1 Application of Soft Measurement Models in the Automatic Control System
4.1.1 Application of the Net Calorific Value of Coal in the Unit Coordinated Control System
Considering the entire unit as a state observer, the real-time value of the coal consumption ratio coefficient K(t) is securely integrated into the “Boiler-Follow-Turbine Coordinated Control System” within DCS, used for fuel control system to the calibration of measured fuel values, referred to as “feedback” correction. This helps compensate for external disturbances due to variations in coal quality. Furthermore, this value can be sent to the boiler’s main control pressure regulator to balance pressure regulation.
When the net calorific value of coal matches the design coal type, K is equal to 1. As actual coal consumption increases, K exceeds 1, and the total coal feed rate command will be divided by K to obtain the corrected total coal feed rate, which serves as the measured value for fuel control system. In this case, the measured value decreases, indicating lower the net calorific value of coal entering the furnace. Through the PID control action of fuel control system more coal is added, and vice versa. Ultimately, the model can be used to calculate RB target coal amounts, fuel-to-water ratios, and more, esolving issues caused by frequent and significant fluctuations in the net calorific value of coal, which can lead to heat transfer deterioration (HTD) in two phase gas-liquid flow, thereby reducing fluctuations in control parameters like main steam temperature and main steam pressure.
4.1.2 Optimization of Oxygen Control System
Boiler combustion adjustments involve multiple tasks such as coal supply control, air supply control, and furnace negative pressure control. This intricate process encompasses various variables that mutually influence and constrain each other. Maintaining stable excess air in the furnace is crucial for ensuring economical combustion. Accurate oxygen measurement helps determine whether fuel is burning completely or if there are air leakage issues in the boiler.
Increasing oxygen requires boosting the boiler’s air supply, enhancing flue gas heat exchange and lowering exhaust gas temperature. However, increasing oxygen levels also leads to higher flue gas flow through the heating surface, causing elevated exhaust gas temperature. These two effects collectively raise exhaust gas temperature. As shown in Fig. 4, if operating the boiler with too little oxygen compromises combustion, increasing heat loss due to incomplete combustion and reducing boiler efficiency. Determining the optimal oxygen value is crucial for improving gas-solid two-phase heat transfer and the overall economic operation of the unit.
Figure 4: Comparison of boiler combustion economics
To ascertain the current optimal oxygen value, we utilize methods such as design values provided by the manufacturer and boiler combustion adjustment experiments. However, these methods usually offer static optimal values and cannot adapt to changes in the boiler’s actual operational state. Our oxygen control optimization method, after boiler combustion adjustment experiments, acquires stable gas-solid two-phase heat transfer schemes, and optimal oxygen control curves under different load conditions are shown in Table 3. During actual boiler operation, these are adjusted based on varying coal’s net calorific values to globally seek the optimal solution for oxygen parameters.
Soft measurement of flue gas oxygen content, with advantages like leading data, high accuracy, and sensitivity, ensures timely, accurate, and rapid effects in boiler combustion adjustments. Soft measurement values completely replace actual measurements, serving as automatic control inputs for oxygen. Employing various strategies, such as reducing PID output speed during load changes, lowering oxygen setpoint during NOx increases, and modifying the oxygen control curve based on the coal consumption ratio coefficient K(t), PID control ensures the excess air coefficient stays near the optimal value.
As shown in Fig. 5, boiler 2’s oxygen level was continuously adjusted under variable loads ranging from 310 to 600 MW, with a maximum static deviation of 0.3% and a maximum dynamic deviation of 1%. This complies with the requirements of China’s DL/T 657-2015 standard for “Steady state Quality Index: ±1%.”
Figure 5: Effects of automatic oxygen control activation
4.1.3 Application of Carbon Content in Fly Ash in Pulverizer Separator Frequency Control
Operators can timely adjust boiler combustion based on real-time data from the soft measurement of fly ash carbon content, controlling it at a lower level while maintaining other crucial boiler parameters in optimal states. Once the stability and accuracy of the fly ash carbon content soft measurement model are validated, it can be applied to the automatic control system of the pulverizer separator frequency as a controlled variable, further enhancing combustion control to achieve energy savings and emissions reduction goals.
4.1.4 Switch Design of Automatic Control System
In the context of the aforementioned automatic control system design, should any anomalies occur in the relevant soft measurement data, the system will automatically maintain the current values, issue an alarm, and switch the corresponding automatic control system to manual mode.
4.2 Mechanisms of NOx Formation in Coal-Fired Boilers and Low Oxygen Emission
The primary principle behind air-staged combustion technology is to segment the fuel combustion process, reduce the air volume in the coal powder combustion zone, increase the coal powder concentration in the combustion zone, delay the mixing time of primary and secondary air, and form a rich fuel zone with an excess air coefficient around 0.75 when coal powder enters the furnace [35]. This facilitates sub-oxidation combustion in the rich fuel zone, reducing combustion speed and temperature, and consequently minimizing NOx generation. The flue gas generated by sub-oxidation combustion is then mixed with secondary air, ensuring complete fuel combustion. Ultimately, as shown in Fig. 6, air-staged combustion can reduce NOx generation by 30% to 40% at furnace exit.
Figure 6: Comparison of flue gas oxygen content and NOx generation trends
Hence, air-staged combustion technology should be combined with oxygen control techniques to achieve the desired goal of lowering NOx emissions. To investigate the impact of reducing oxygen levels within the boiler, experiments were conducted. The results indicate that under stable operating conditions, there exists a peak point in the furnace temperature as oxygen levels change. The influence of reducing oxygen levels on combustion and boiler operating parameters varies on either side of this peak point. There is also a peak point in NOx concentration near the furnace temperature peak, and a valley point when oxygen levels are significantly reduced. Only when oxygen levels are within the peak-valley range can reducing oxygen levels lead to a decrease in NOx concentration.
This reduction in oxygen levels increases the swirl index during steady-state conditions and fluctuates significantly during load reduction, leading to suboptimal gas-solid two-phase heat transfer and inadequate gas-liquid two-phase heat exchange effects, resulting in issues such as large steam temperature deviations and over-temperature. However, if lowering oxygen levels do not significantly increase the heat loss due to incomplete combustion, it will increase the fluctuation amplitudes of furnace temperature and NOx concentration during load regulation. Therefore, we have adopted a simplified strategy: When the SCR reaction zone’s inlet NOx concentration remains continuously above 390 mg/Nm3 for 10 s, oxygen setpoints are moderately reduced. This strategy has notably reduced the difficulty in controlling the ammonia injection quantity and NOx emission concentration in the SCR reaction zone, achieving a significant overall emission reduction. It also improved the swirl index during steady-state conditions and load regulation, providing strong support for gas-solid two-phase heat transfer and gas-liquid two-phase heat exchange effects.
In comparison with existing combustion optimization technologies, the proposed methods exhibit an exceptionally high investment-return ratio, outlined as follows:
The modeling approach presented in this paper, distinct from other costly and intricate methods, operates without algorithmic constraints. It exclusively relies on DCS, necessitating no new measurement points, additional equipment, or investments. With virtually zero cost and no alteration to the existing system structure, it addresses the industry challenge of inaccurately measuring crucial parameters in boiler combustion systems. Furthermore, when compared to traditional thermal power plants, significant fixed investment savings ranging from 3 to 3.5 million RMB are achieved. This encompasses expenses linked to procuring hardware measurement devices for newly constructed coal-fired power plants or technological upgrades. Additionally, a notable decrease in failure rates and a substantial reduction in maintenance requirements result in an annual maintenance cost saving of approximately 200,000–300,000 RMB.
The soft measurement of coal’s net calorific value effectively augments the unit’s rapid load response capability. Real-time guidance from soft measurement values of fly ash carbon content aids operators in economically adjusting combustion. After integrating soft measurement values of oxygen content into automatic control, the adjustment quality is improved. Coordinated control of oxygen content and NOx is achieved, reducing the difficulty in controlling NOx emission concentration and overall emissions. The comprehensive reduction in the power supply coal consumption is estimated at approximately 1.62 g/(kW h). Specifically, on the day when operators strictly adjust boiler combustion based on the fly ash soft measurement model, the fly ash carbon content decreases, averaging from 2.94% to 2.14%, resulting in a 0.8% decrease. Considering the average reduction of 1% in fly ash carbon content leading to a decrease of 1.019 g/(kW h) in standard coal consumption rate, this translates to a reduction of 0.82 g/(kW h). In the month before the deployment of the flue gas oxygen content soft measurement, the plant’s flue gas heat loss was 5.35%. After the soft measurement system’s implementation, this heat loss decreased to 5.11%, contributing to a calculated reduction of 0.8 g/(kW h) in the standard coal consumption rate.
In summary, this study has achieved noteworthy milestones in soft measuring the net calorific value of coal, flue gas oxygen content, and fly ash carbon content using hybrid modeling technology. The robustness of the findings is confirmed through performance test data, showcasing controlled RMSE and MAXE values, both consistently within the range of 0.203. The investigation into oxygen control, coal consumption ratio coefficient correction, and related aspects has yielded successful outcomes, optimizing boiler combustion processes. Meticulous real-time analysis of oxygen values and SCR reaction zone entrance NOx concentrations, coupled with the precise implementation of coordinated control of oxygen content and NOx, not only led to a substantial 30% to 40% reduction in NOx generation but also brought about significant optimization of boiler operating parameters. This optimization is estimated to result in a comprehensive reduction in the power supply coal consumption, reaching approximately 1.62 g/(kW h).
The proposed methods not only ensure energy savings and emissions reduction but also maintain the safe operation of the unit. Furthermore, the positive outcomes of this research contribute invaluable, practical, and replicable experiences in the domains of gas-solid two-phase heat transfer and gas-liquid two-phase heat exchange. Looking ahead, the continuous development of thermal parameter soft measurement technology and automatic control technology for coal-fired power plants holds paramount guiding significance for future advancements in the field.
Acknowledgement: None.
Funding Statement: The authors received no specific funding for this study.
Author Contributions: The authors confirm contribution to the paper as follows: study conception and design: Ming Yan and Pan Shi; data collection: Ming Yan and Meiling Zhang; analysis and interpretation of results: Jiawei Zhang and Liang Wang; draft manuscript preparation: Ming Yan and Liang Wang. All authors reviewed the results and approved the final version of the manuscript.
Availability of Data and Materials: The datasets utilized in this paper are available upon request.
Conflicts of Interest: The authors declare that they have no conflicts of interest to report regarding the present study.
References
1. Jing, X. H., Li, W., Li, Z. Y., Wang, Z. Y. (2023). Numerical simulation of combustion in 300 MW tangentially fired pulverized high-alkali coal boiler on underload operation. Frontiers in Heat and Mass Transfer, 20(1), 1–4. https://doi.org/10.5098/hmt.20.19 [Google Scholar] [CrossRef]
2. Wang, H. P., Ti, S. G., Jin, H. Z., Wang, H., Gao, K. et al. (2022). Influence of overfire air rate of the two-channel overfire air on flow and combustion characteristics in a 600-MWe boiler. Asia-Pacific Journal of Chemical Engineering, 17(1), e2726. https://doi.org/10.1002/apj.v17.1 [Google Scholar] [CrossRef]
3. Liu, C. L., Li, Z. Q., Zeng, L. Y., Zhang, Q. H., Hu, R. C. et al. (2016). Gas/particle two-phase flow characteristics of a down-fired 350 MWe supercritical utility boiler at different tertiary air ratios. Energy, 102, 54–64. [Google Scholar]
4. Stupar, G., Tucakovi, D., Zivanovi, T., Belo, S. (2015). Assessing the impact of primary measures for NOx reduction on the thermal power plant steam boiler. Applied Thermal Engineering, 78(5), 397–409. [Google Scholar]
5. Zhang, Z. W., Wang, P. (2019). Numerical simulation of internal flow in direct burning coal-fired hot flue gas furnace. Journal of Physics Conference Series,1187, 022025. https://doi.org/10.1088/1742-6596/1187/2/022025 [Google Scholar] [CrossRef]
6. Zhan, Z. G., Liu, Y. M., Fang, Q. Y., Du, L., Zhang, C. et al. (2014). Effect of horizontal staging of secondary air on NOx emissions in a 600 MW tangentially coal-fired utility boiler. International Conference on Materials for Renewable Energy and Environment (ICMREE), pp. 745–749, Chengdu, China. [Google Scholar]
7. Zheng, L. G., Zhou, H., Cen, K. F., Wang, C. L. (2009). A comparative study of optimization algorithms for low NOx combustion modification at a coal-fired utility boiler. Expert Systems with Applications, 36(2), 2780–2793. https://doi.org/10.1016/j.eswa.2008.01.088 [Google Scholar] [CrossRef]
8. Kouprianov, V. I., Tanetsakunvatana, V. (2003). Optimization of excess air for the improvement of environmental performance of a 150 MW boiler fired with Thai lignite. Applied Energy, 74(3–4), 445–453. [Google Scholar]
9. Kuang, M., Yang, G. H., Zhu, Q. Y., Ti, S. G., Wang, Z. F. (2017). Effect of burner location on flow-field deflection and asymmetric combustion in a 600 MWe supercritical down-fired boiler. Applied Energy, 206, 1393–1405. https://doi.org/10.1016/j.apenergy.2017.09.121 [Google Scholar] [CrossRef]
10. Yao, G. J., Han, X.J, Liu, Z. D., Tang, H., Zhou, Y. Q. et al. (2023). Low-NOx study of a 600 MW tangentially fired boiler based on pulverized coal preheating method. Case Studies in Thermal Engineering, 48, 103156. https://doi.org/10.1016/j.csite.2023.103156 [Google Scholar] [CrossRef]
11. Hultgren, M., Ikonen, E., Kovács, J. (2019). Integrated control and process design for improved load changes in fluidized bed boiler steam path. Chemical Engineering Science, 199, 164–178. https://doi.org/10.1016/j.ces.2019.01.025 [Google Scholar] [CrossRef]
12. Wang, F., Huang, Q. X., Liu, D., Yan, J. H., Cen, K. F. (2008). Improvement of load-following capacity based on the flame radiation intensity signal in a power plant. Energy & Fuels, 22(3), 1731–1738. https://doi.org/10.1021/ef700620n [Google Scholar] [CrossRef]
13. Fang, F., Wei, L. (2011). Backstepping-based nonlinear adaptive control for coal-fired utility boiler-turbine units. Applied Energy, 88(3), 814–824. https://doi.org/10.1016/j.apenergy.2010.09.003 [Google Scholar] [CrossRef]
14. Xu, W. T., Huang, Y. J., Song, S. H., Chen, B., Qi, X. M. (2023). A novel online combustion optimization method for boiler combining dynamic modeling, multi-objective optimization and improved case-based reasoning. Fuel, 337, 126854. https://doi.org/10.1016/j.fuel.2022.126854 [Google Scholar] [CrossRef]
15. Cao, H. L., Sun, S. Z., Liu, Y. H., Wall, T. F. (2010). Computational fluid dynamics modeling of NOx reduction mechanism in oxyfuel combustion. Energy & Fuels, 24, 131–135. https://doi.org/10.1021/ef900524b [Google Scholar] [CrossRef]
16. Qin, N., Li, R. J. (2020). Online simplified model and experimental comparison of CFB boiler thermal efficiency. Applied Thermal Engineering, 171, 115021. https://doi.org/10.1016/j.applthermaleng.2020.115021 [Google Scholar] [CrossRef]
17. Park, H. Y., Baek, S. H., Kim, Y. J., Kim, T. H., Kang, D. S. et al. (2013). Numerical and experimental investigations on the gas temperature deviation in a large scale, advanced low NOx, tangentially fired pulverized coal boiler. Fuel, 104, 641–646. https://doi.org/10.1016/j.fuel.2012.06.091 [Google Scholar] [CrossRef]
18. Tang, J. R., Li, Q. B., Wang, S. K., Yu, H. S. (2023). Thermo-economic optimization and comparative analysis of different organic flash cycles for the supercritical CO2 recompression Brayton cycle waste heat recovery. Energy, 278, 128002. https://doi.org/10.1016/j.energy.2023.128002 [Google Scholar] [CrossRef]
19. Li, B., Wang, K., Zhu, B. Y. (2020). Study on energy-saving operation technology of environmental protection facilities of ultra-low emission coal-fired unit. Energy Reports, 6, 1356–1364. https://doi.org/10.1016/j.egyr.2020.11.021 [Google Scholar] [CrossRef]
20. Wang, C. L., Liu, Y., Zheng, S., Jiang, A. P. (2018). Optimizing combustion of coal fired boilers for reducing NOx emission using gaussian process. Energy, 153, 149–158. https://doi.org/10.1016/j.energy.2018.01.003 [Google Scholar] [CrossRef]
21. Lee, T., Han, E., Moon, U. C., Lee, K. Y. (2020). Supplementary control of air-fuel ratio using dynamic matrix control for thermal power plant emission. Energies, 13(1), 226. https://doi.org/10.3390/en13010226 [Google Scholar] [CrossRef]
22. Hu, X. Y., Niu, B., P., F., Wang, J. M., Zhang, X. X. (2020). Multi-objective prediction of coal-fired boiler with a deep hybrid neural networks. Atmospheric Pollution Research, 11(7), 1084–1090. https://doi.org/10.1016/j.apr.2020.04.001 [Google Scholar] [CrossRef]
23. Xu, X., Chen, Q., Ren, M. F., Cheng, L., Xie, J. (2019). Combustion optimization for coal fired power plant boilers based on improved distributed ELM and distributed PSO. Energies, 12(6), 1036. https://doi.org/10.3390/en12061036 [Google Scholar] [CrossRef]
24. Wang, P. L., Li, Q. B., Wang, S. K., He, C., Wu, C. (2024). Off-design performance evaluation of thermally integrated pumped thermal electricity storage systems with solar energy. Energy Conversion and Management, 301, 118001. https://doi.org/10.1016/j.enconman.2023.118001 [Google Scholar] [CrossRef]
25. Shi, Y., Zhang, W. Q., Chen, X., Yu, A. B., Li, J. (2019). Combustion optimization of ultra supercritical boiler based on artificial intelligence. Energy, 170, 804–817. https://doi.org/10.1016/j.energy.2018.12.172 [Google Scholar] [CrossRef]
26. Ren, T., Modest, M. F., Fateev, A., Sutton, G., Zhao, W. J. et al. (2019). Machine learning applied to retrieval of temperature and concentration distributions from infrared emission measurements. Applied Energy, 252, 113448. https://doi.org/10.1016/j.apenergy.2019.113448 [Google Scholar] [CrossRef]
27. Mohamed, O., Khalil, A., Wang, J. H. (2020). Modeling and control of supercritical and ultra-supercritical power plants: A review. Energies, 13(11), 2935. https://doi.org/10.3390/en13112935 [Google Scholar] [CrossRef]
28. Cui, J. H., Chai, T. Y., Liu, X. J. (2020). Deep-neural-network-based economic model predictive control for ultra-supercritical power plant. IEEE Transactions on Industrial Informatics, 16(9), 5905–5913. https://doi.org/10.1109/TII.9424 [Google Scholar] [CrossRef]
29. Mi, C. L., Guo, J. L., Li, X. G., Wei, G., An, G. Y. (2015). Flue gas determination based soft-sensing model for coal quality monitoring for utility boilers. Thermal Power Generation, 44(7), 62–65 (In Chinese). [Google Scholar]
30. Li, H. Y., Yang, P. Y. (2013). Research and application of real-time measurement for lower calorific value of burning coal in coal-fired power plants. Electric Power Construction, 34(10), 60–64 (In Chinese). [Google Scholar]
31. Zhao, Z., Zeng, D. L., Tian, L., Liu, J. Z. (2005). Research on soft-sensing of oxygen content based on datafusion. Proceedings of the CSEE, 25(7), 7–12 (In Chinese). [Google Scholar]
32. Cai, J. J., Ma, X. Q., Liao, Y. F. (2006). Study on boiler’s operation performance and optimization of oxygen content in flue gas. Thermal Power Generation, 7, 28–30 (In Chinese). [Google Scholar]
33. Fu, J. F., Feng, J. K. (2005). Resident time of combustion products and its effect on carbon content in fly ash in pulverized coal fired boilers. Boiler Technology, 36(6), 21–24 (In Chinese). [Google Scholar]
34. Zhao, Z., Chen, X. H., Wang, Y. (2009). Research on soft-sensing of coal moisture. Electric Power Science and Engineering, 25(11), 67–69 (In Chinese). [Google Scholar]
35. Zhou, C. Y., Wang, Y. Q., Jin, Q. Y., Chen, Q. J., Zhou, Y. G. (2019). Mechanism analysis on the pulverized coal combustion flame stability and NOx emission in a swirl burner with deep air staging. Journal of the Energy Institute, 92(2), 298–310 (In Chinese). https://doi.org/10.1016/j.joei.2018.01.006 [Google Scholar] [CrossRef]
Cite This Article
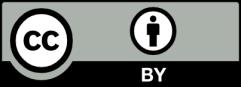
This work is licensed under a Creative Commons Attribution 4.0 International License , which permits unrestricted use, distribution, and reproduction in any medium, provided the original work is properly cited.