Open Access
ARTICLE
Artificial Neural Network Modeling for Predicting Thermal Conductivity of EG/Water-Based CNC Nanofluid for Engine Cooling Using Different Activation Functions
1 Faculty of Computing, Universiti Malaysia Pahang Al-Sultan Abdullah, Pekan, Pahang, 26600, Malaysia
2 Faculty of Mechanical and Automotive Engineering Technology, Universiti Malaysia Pahang Al-Sultan Abdullah, Pekan, Pahang, 26600, Malaysia
3 Department of Management and Information Technology, St. Francis College, 179 Livingston St, Brooklyn, NY, 11201, USA
4 Dell Technologies, Data Analytics, and Automation, Bukit Tengah, Bukit Mertajam, 14000, Malaysia
5 Department of Computer Science, Adrian College, Adrian, MI, USA
6 Department of Computer Science & Engineering, United International University Bangladesh, United City, Madani Avenue, Badda, Dhaka, 1212, Bangladesh
* Corresponding Author: Md Mustafizur Rahman. Email:
(This article belongs to the Special Issue: Machine Learning and Data-Driven Techniques for Mass and Thermal Transport)
Frontiers in Heat and Mass Transfer 2024, 22(2), 537-556. https://doi.org/10.32604/fhmt.2024.047428
Received 05 November 2023; Accepted 19 February 2024; Issue published 20 May 2024
Abstract
A vehicle engine cooling system is of utmost importance to ensure that the engine operates in a safe temperature range. In most radiators that are used to cool an engine, water serves as a cooling fluid. The performance of a radiator in terms of heat transmission is significantly influenced by the incorporation of nanoparticles into the cooling water. Concentration and uniformity of nanoparticle distribution are the two major factors for the practical use of nanofluids. The shape and size of nanoparticles also have a great impact on the performance of heat transfer. Many researchers are investigating the impact of nanoparticles on heat transfer. This study aims to develop an artificial neural network (ANN) model for predicting the thermal conductivity of an ethylene glycol (EG)/water-based crystalline nanocellulose (CNC) nanofluid for cooling internal combustion engine. The implementation of an artificial neural network considering different activation functions in the hidden layer is made to find the best model for the cooling of an engine using the nanofluid. Accuracies of the model with different activation functions in artificial neural networks are analyzed for different nanofluid concentrations and temperatures. In artificial neural networks, Levenberg–Marquardt is an optimization approach used with activation functions, including Tansig and Logsig functions in the training phase. The findings of each training, testing, and validation phase are presented to demonstrate the network that provides the highest level of accuracy. The best result was obtained with Tansig, which has a correlation of 0.99903 and an error of 3.7959 ×10. It has also been noticed that the Logsig function can also be a good model due to its correlation of 0.99890 and an error of 4.9218 ×10. Thus our ANN with Tansig and Logsig functions demonstrates a high correlation between the actual output and the predicted output.Graphic Abstract
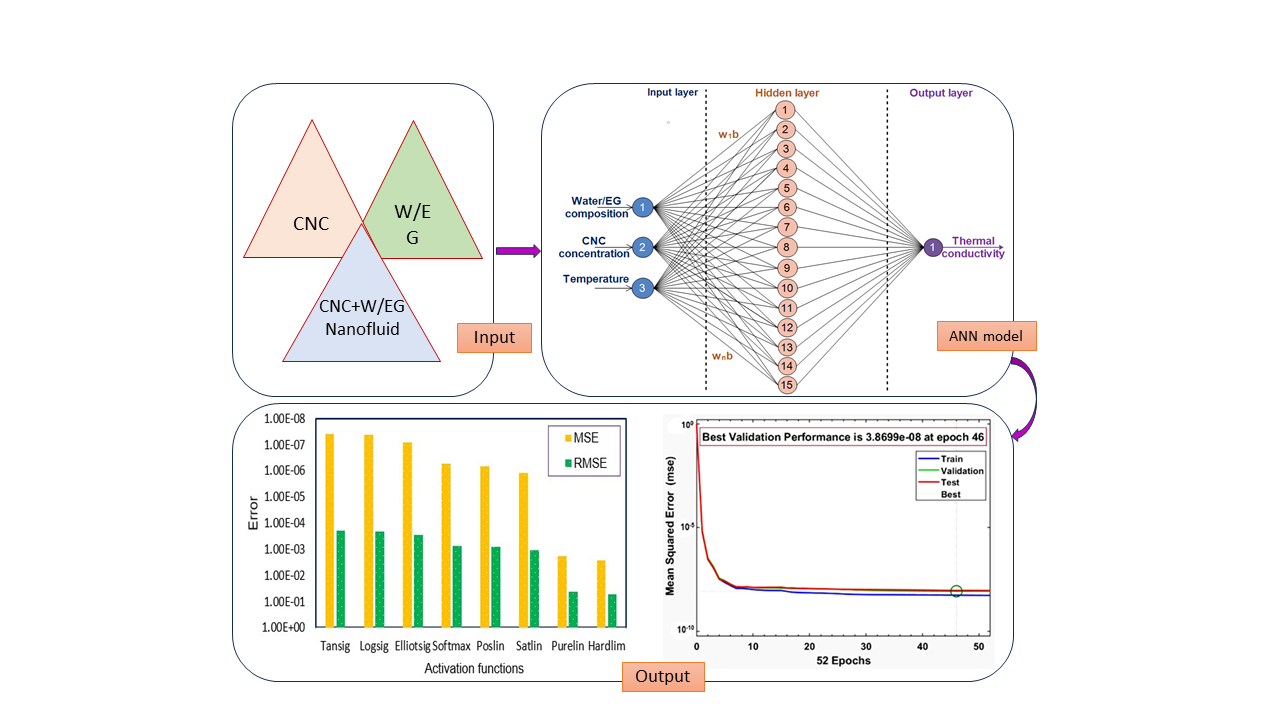
Keywords
Cite This Article
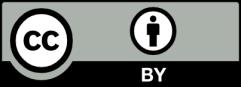
This work is licensed under a Creative Commons Attribution 4.0 International License , which permits unrestricted use, distribution, and reproduction in any medium, provided the original work is properly cited.