Open Access
ARTICLE
Prediction and Optimization of the Thermal Properties of TiO2/Water Nanofluids in the Framework of a Machine Learning Approach
1 Sate Key Laboratory of Complex Nonferrous Clean Utilization, Kunming University of Science and Technology, Kunming, 650093, China
2 Faculty of Metallurgical and Energy Engineering, Kunming University of Science and Technology, Kunming, 650093, China
3 Jiangxi Guoxing Intelligent Energy Co., Ganzhou, 330300, China
4 Faculty of Media and Information Engineering, Yunnan Open University, Kunming, 650500, China
* Corresponding Author: Shan Qing. Email:
(This article belongs to the Special Issue: Advances in Nanofluids: Modelling, Simulation and Applications)
Fluid Dynamics & Materials Processing 2023, 19(8), 2181-2200. https://doi.org/10.32604/fdmp.2023.027299
Received 24 October 2022; Accepted 14 December 2022; Issue published 04 April 2023
Abstract
In this study, comparing multiple models of machine learning, a multiple linear regression (MLP), multilayer feed-forward artificial neural network (BP) model, and a radial-basis feed-forward artificial neural network (RBF-BP) model are selected for the optimization of the thermal properties of TiO2/water nanofluids. In particular, the least squares support vector machine (LS-SVM) method and radial basis support vector machine (RB-SVM) method are implemented. First, curve fitting is performed by means of multiple linear regression in order to obtain bivariate correlation functions for thermal conductivity and viscosity of the nanofluid. Then the aforementioned models are used for a predictive analysis of the dependence of its thermal conductivity and viscosity on temperature and volume fraction. The results show that the least squares support vector machine (LS-SVM) has a prediction accuracy higher than the other models. The model predicts the thermal conductivity of TiO2/water MSE = 1.0853 × 10−6, R2 = 0.99864, MAE = 0.00092, RMSE = 0.00104, and the viscosity of TiO2/water MSE = 8.1397 × 10−6, R2 = 0.99995, MAE = 0.00074, RMSE = 0.0009.Keywords
Cite This Article
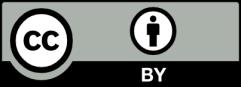