Open Access
ARTICLE
Fatigue Life Estimation of High Strength 2090-T83 Aluminum Alloy under Pure Torsion Loading Using Various Machine Learning Techniques
Electromechanical Engineering Department, University of Technology-Iraq, Baghdad, Iraq
* Corresponding Author: Mustafa Sami Abdullatef. Email:
(This article belongs to the Special Issue: Recent advancements in thermal fluid flow applications)
Fluid Dynamics & Materials Processing 2023, 19(8), 2083-2107. https://doi.org/10.32604/fdmp.2023.027266
Received 23 October 2022; Accepted 14 December 2022; Issue published 04 April 2023
Abstract
The ongoing effort to create methods for detecting and quantifying fatigue damage is motivated by the high levels of uncertainty in present fatigue-life prediction approaches and the frequently catastrophic nature of fatigue failure. The fatigue life of high strength aluminum alloy 2090-T83 is predicted in this study using a variety of artificial intelligence and machine learning techniques for constant amplitude and negative stress ratios (). Artificial neural networks (ANN), adaptive neuro-fuzzy inference systems (ANFIS), support-vector machines (SVM), a random forest model (RF), and an extreme-gradient tree-boosting model (XGB) are trained using numerical and experimental input data obtained from fatigue tests based on a relatively low number of stress measurements. In particular, the coefficients of the traditional force law formula are found using relevant numerical methods. It is shown that, in comparison to traditional approaches, the neural network and neuro-fuzzy models produce better results, with the neural network models trained using the boosting iterations technique providing the best performances. Building strong models from weak models, XGB helps to predict fatigue life by reducing model partiality and variation in supervised learning. Fuzzy neural models can be used to predict the fatigue life of alloys more accurately than neural networks and traditional methods.Graphic Abstract
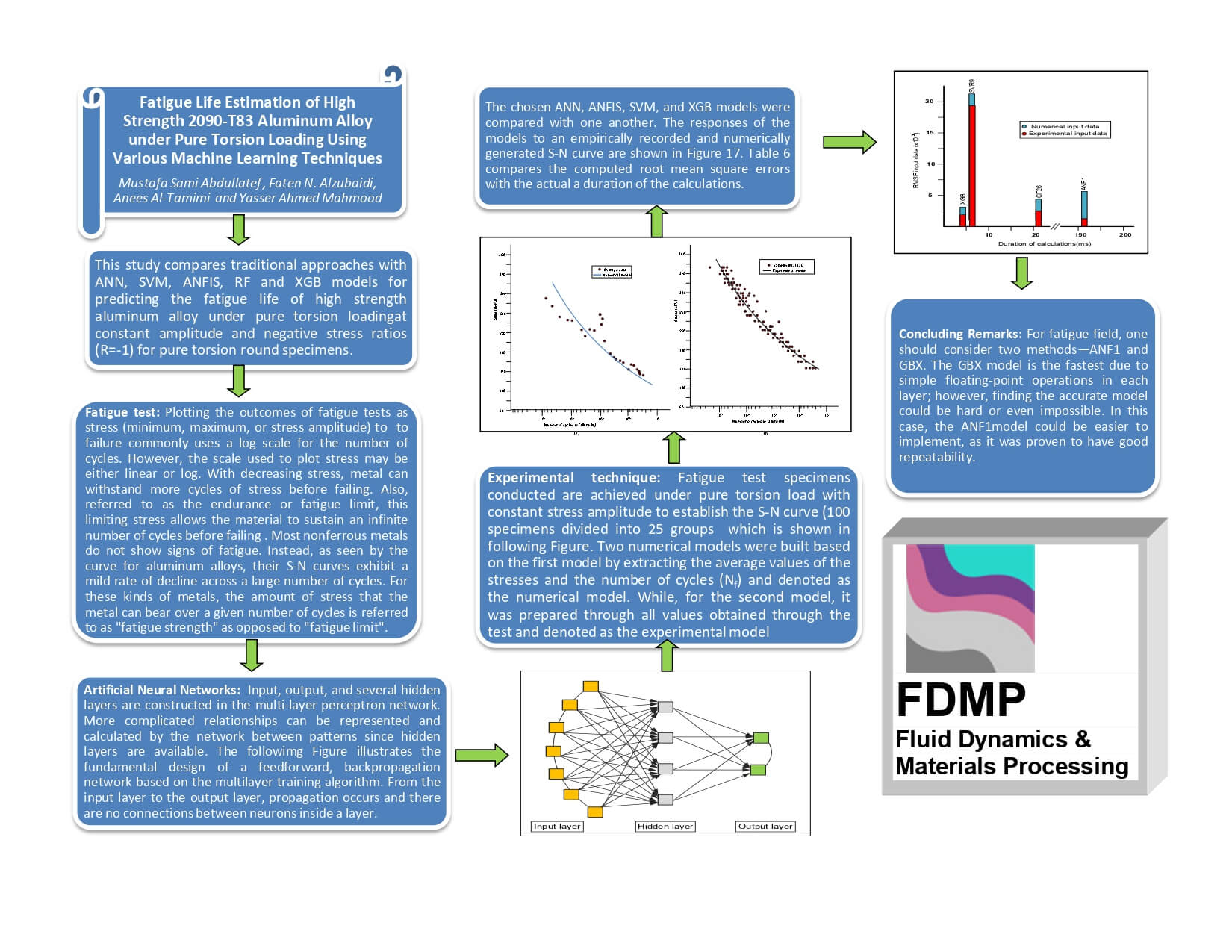
Keywords
Cite This Article
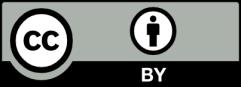
This work is licensed under a Creative Commons Attribution 4.0 International License , which permits unrestricted use, distribution, and reproduction in any medium, provided the original work is properly cited.