Open Access
REVIEW
Anomaly Detection in Textured Images with a Convolutional Neural Network for Quality Control of Micrometric Woven Meshes
1 Université de Lorraine CNRS Inria LORIA, Nancy, 54000, France
2 IUT de Saint-Dié Université de Lorraine, Saint-Dié-des-Vosges, 88100, France
3 Technical University of Cluj-Napoca, Cluj-Napoca, 400114, Romania
* Corresponding Author: Pierre-Frédéric Villard. Email:
(This article belongs to the Special Issue: Materials and Energy an Updated Image for 2021)
Fluid Dynamics & Materials Processing 2022, 18(6), 1639-1648. https://doi.org/10.32604/fdmp.2022.021726
Received 04 February 2022; Accepted 07 March 2022; Issue published 27 June 2022
Abstract
Industrial woven meshes are composed of metal materials and are often used in construction, industrial and residential activities or applications. The objective of this work is defect detection in industrial fabrics in the quality control stage. In order to overcome the limitations of manual methods, which are often tedious and time-consuming, we propose a strategy that can automatically detect defects in micrometric steel meshes by means of a Convolutional Neural Network. The database used for such a purpose comes from real problem data for anomaly detection in micrometric woven meshes. This detection is performed through supervised classification with a Convolutional Neural Network using a VGG19 architecture. We define a pipeline and a strategy to tackle the related small amount of data. It includes i) augmenting the database with translation, rotation and symmetry, ii) using pre-trained weights and iii) checking the learning curve behaviour through cross-validation. The obtained results show that, despite the small size of our databases, detection accuracy of 96% was reached.Keywords
Cite This Article
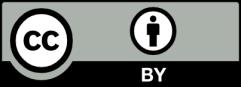
This work is licensed under a Creative Commons Attribution 4.0 International License , which permits unrestricted use, distribution, and reproduction in any medium, provided the original work is properly cited.