Open Access
ARTICLE
Application of an Artificial Neural Network Method for the Prediction of the Tube-Side Fouling Resistance in a Shell-And-Tube Heat Exchanger
1 Research Laboratory “Process, Energy, Environment & Electrical Systems”, National Engineering School of Gabes, Gabes, 6029, Tunisia
2 CMGPCE Laboratory, French Institute of Refrigeration (IFFI), National Conservatory of Arts and Crafts (CNAM), Paris, 75141, France
* Corresponding Author: Rania Jradi. Email:
(This article belongs to the Special Issue: Materials and Energy an Updated Image for 2021)
Fluid Dynamics & Materials Processing 2022, 18(5), 1511-1519. https://doi.org/10.32604/fdmp.2022.021925
Received 13 February 2022; Accepted 09 March 2022; Issue published 27 May 2022
Abstract
The accumulation of undesirable deposits on the heat exchange surface represents a critical issue in industrial heat exchangers. Taking experimental measurements of the fouling is relatively difficult and, often, this method does not lead to precise results. To overcome these problems, in the present study, a new approach based on an Artificial Neural Network (ANN) is used to predict the fouling resistance as a function of specific measurable variables in the phosphoric acid concentration process. These include: the phosphoric acid inlet and outlet temperatures, the steam temperature, the phosphoric acid density, the phosphoric acid volume flow rate circulating in the loop. Some statistical accuracy indices are employed simultaneously to justify the interrelation between these independent variables and the fouling resistance and to select the best training algorithm allowing the determination of the optimal number of hidden neurons. In particular, the BFGS quasi-Newton back-propagation approach is found to be the most performing of the considered training algorithms. Furthermore, the best topology ANN for the shell and tube heat exchanger is obtained with a network consisting of one hidden layer with 13 neurons using a tangent sigmoid transfer function for the hidden and output layers. This model predicts the experimental values of the fouling resistance with AARD% = 0.065, MSE = 2.168 × 10−11, RMSE = 4.656 × 10−6 and r2 = 0.994.Keywords
Cite This Article
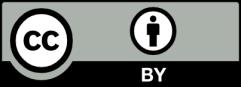
This work is licensed under a Creative Commons Attribution 4.0 International License , which permits unrestricted use, distribution, and reproduction in any medium, provided the original work is properly cited.