Open Access
ARTICLE
Machine Learning-Based Prediction of Oil-Water Flow Dynamics in Carbonate Reservoirs
School of Geosciences, Yangtze University, Wuhan, 430100, China
* Corresponding Author: Xianhe Yue. Email:
(This article belongs to the Special Issue: Meshless, Mesh-Based and Mesh-Reduction Methods Based Analysis of Fluid Flow in Porous Media)
Fluid Dynamics & Materials Processing 2022, 18(4), 1195-1203. https://doi.org/10.32604/fdmp.2022.020649
Received 05 December 2021; Accepted 01 March 2022; Issue published 06 April 2022
Abstract
Because carbonate rocks have a wide range of reservoir forms, a low matrix permeability, and a complicated seam hole formation, using traditional capacity prediction methods to estimate carbonate reservoirs can lead to significant errors. We propose a machine learning-based capacity prediction method for carbonate rocks by analyzing the degree of correlation between various factors and three machine learning models: support vector machine, BP neural network, and elastic network. The error rate for these three models are 10%, 16%, and 33%, respectively (according to the analysis of 40 training wells and 10 test wells).Keywords
Cite This Article
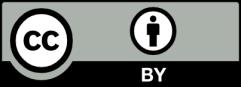
This work is licensed under a Creative Commons Attribution 4.0 International License , which permits unrestricted use, distribution, and reproduction in any medium, provided the original work is properly cited.