Open Access
ARTICLE
Photovoltaic Power Prediction Cosidering Mode Switching and Parallel Weight Adjustment
1 School of Electrical Engineering and Automation, Henan Polytechnic University, Jiaozuo, 454003, China
2 Key Laboratory of Smart Grid of Ministry of Education, Tianjin University, Tianjin, 300072, China
3 Dispatching Center, Dongguan Power Supply Bureau of Guangdong Power Grid Co., Ltd., Dongguan, 523000, China
* Corresponding Author: Penghui Liu. Email:
(This article belongs to the Special Issue: Modelling, Optimisation and Forecasting of Photovoltaic and Photovoltaic thermal System Energy Production)
Energy Engineering 2025, 122(4), 1387-1402. https://doi.org/10.32604/ee.2025.062627
Received 23 December 2024; Accepted 10 February 2025; Issue published 31 March 2025
Abstract
The photovoltaic (PV) output process is inherently complex, often disrupted by a multitude of meteorological factors, while conventional detection methods at PV power stations prove inadequate, compromising prediction accuracy. To address this challenge, this paper introduces a power prediction method that leverages modal switching (MS), weight factor adjustment (WFA), and parallel long short-term memory (PALSTM). Initially, historical PV power station data is categorized into distinct modes based on global horizontal irradiance and converted solar angles. Correlation analysis is then employed to evaluate the impact of various meteorological factors on PV power, selecting those with strong correlations for each specific mode. Subsequently, the weights of meteorological parameters are optimized and adjusted, and a PALSTM neural network is constructed, with its parallel modal parameters refined through training. Depending on the prediction time and input data mode characteristics, the appropriate mode channel is selected to forecast PV power station generation. Ultimately, the feasibility of this method is validated through an illustrative analysis of measured data from an Australian PV power station. Comparative test results underscore the method’s advantages, particularly in scenarios where existing detection methods are lacking and meteorological factors frequently fluctuate, demonstrating its superior prediction accuracy and stability.Keywords
Cite This Article
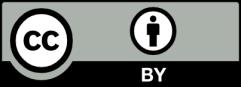
This work is licensed under a Creative Commons Attribution 4.0 International License , which permits unrestricted use, distribution, and reproduction in any medium, provided the original work is properly cited.