Open Access
REVIEW
Research of Low-Carbon Operation Technologies for PEDF Parks: Review, Prospects, and Challenges
1 Institute of Electricity Consumption and Measurement Technology, CSG Electric Power Research Institute, Guangzhou, 510663, China
2 Guangdong Provincial Key Laboratory of Intelligent Measurement and Advanced Metering of Power Grid, Guangzhou, 510610, China
3 Micro Energy Group, Guangzhou Institute of Energy Conversion, Chinese Academy of Sciences, Guangzhou, 510640, China
* Corresponding Author: Tonghe Wang. Email:
Energy Engineering 2025, 122(4), 1221-1248. https://doi.org/10.32604/ee.2025.061452
Received 25 November 2024; Accepted 14 February 2025; Issue published 31 March 2025
Abstract
With the severe challenges brought by global climate change, exploring and developing clean and renewable energy systems to upgrade the energy structure has become an inevitable trend in related research. The comprehensive park systems integrated with photovoltaic, energy storage, direct current, and flexible loads (PEDF) is able to play an important role in promoting energy transformation and achieving sustainable development. In order to fully understand the advantages of PEDF parks in energy conservation and carbon reduction, this paper summarizes existing studies and prospects future research directions on the low-carbon operation of the PEDF park. This paper first introduces carbon emission monitoring and evaluation methods, and then analyzes bi-level optimal dispatch strategies for flexible loads. Meanwhile, the paper provides a prospective analysis of the innovations that can be brought by advanced technologies to the PEDF park. Finally, this paper puts forward the challenges faced by current research and provides prospects for future research directions. This paper emphasizes that related research should focus on collaborating key technologies of PEDF systems and integrating advanced innovations to address challenges and fully leverage the advantages of PEDF technology in energy saving and carbon reduction. This paper aims to provide systematic theoretical guidance and supplements for the research and practice of the PEDF technology.Keywords
Under the severe challenge of global climate change, energy transition has become a common focus of attention for all countries. With the acceleration of industrialization and urbanization, energy demand continues to grow. Traditional fossil energy systems not only have limited resources, but also generate a large amount of greenhouse gases during use, exacerbating the crisis of global warming. Therefore, exploring and developing clean and renewable energy (RE) systems to upgrade the energy structure has become an inevitable trend in global energy development [1].
As a new type of energy system, the comprehensive park system integrated with photovoltaic, energy storage, direct current, and flexible load (PEDF) technologies (PEDF park for short) demonstrates enormous application potential and development prospects [2]. The system achieves direct utilization of solar energy through photovoltaic (PV) power generation, reducing dependence on fossil fuels; balancing energy supply and demand through energy storage (ES) systems to improve energy utilization efficiency; optimizing energy transmission through direct current (DC) distribution to reduce transmission losses; achieve intelligent regulation of adjustable loads, enhancing the flexibility and stability of the system. These characteristics make the PEDF park of great significance in promoting energy transformation and achieving low-carbon development [3].
Related research has explored various components of the PEDF system, including the specific implementation methods and optimization strategies of the four key components (PV, ES, DC distribution, and flexible load), gradually improving the PEDF theoretical framework. Research on PV technologies focuses on improving the efficiency of PV power generation [4], reducing the cost of PV panels [5], and optimizing the layout of PV panels on building surfaces [6]. In terms of ES technology, the application of battery energy storage (BES) [7], supercapacitor ES [8], etc., in PEDF systems, as well as the optimization configuration and dispatch strategies of ES systems [9,10], have been explored. Research on DC distribution systems has proposed topology structures [11], control strategies [12], and interface technologies with other systems [13] to improve energy transmission efficiency and stability. The research on flexible load technology aims to achieve flexible interaction between flexible loads in energy systems and power grids, including demand side response [14], virtual power plants [15], and other functions to improve the flexibility and reliability of the system [16].
Although the PEDF technology has many advantages in theory, it still faces problems of insufficient technological maturity and poor stability in practical applications. Further optimization and improvement of related technologies are needed to enhance the overall performance and stability of the system [17]. In addition, PEDF technology involves the integration and optimization of multiple subsystems. How to achieve collaborative work between various subsystems and improve the overall efficiency and reliability of the system is also an important challenge facing current academic research [18].
At present, there are some survey and prospect papers in the field of PEDF technology, which have made contributions in constructing theoretical foundations, depicting technological panorama, and outlooking for future development prospects. They not only systematically analyze the core concepts, technical architecture, and global development trends and future trends of PEDF technology, but also highlight the profound impact of this technology on improving energy system efficiency and promoting low-carbon economic transformation through detailed case studies. In [19], the authors emphasize the advantages of applying DC distribution in the energy management and carbon reduction in PEDF buildings, but they simply provide overall feasibility analysis that lacks support for theoretical and technical details. Similarly, reference [20] aims to establish a DC distribution network architecture in order to facilitate an energy management system for the PEDF building, but how PV, ES, DC distribution networks, and flexible loads will collaborate under the energy management system is not explored in depth. In contrast, reference [21] summarizes the structure of DC microgrid in PEDF buildings and analyzes its coordinated control strategies in detail. The work focuses on control strategy selection under different control modes, but how different control strategies contribute to the energy utilization and carbon reduction is not involved. In [22], the authors provide an overview of PEDF technologies as well as application examples of PEDF buildings. Although great technical details are included in the work, the technologies of the four key components of PEDF systems are introduced in a rather separate way so that the interaction between these components are not clearly described.
In summary, these studies still have some shortcomings. On the one hand, existing review papers tend to summarize PV, ES, DC distribution, and flexible load technologies separately, lacking a systematic view and analysis of the various components and their interaction mechanisms of PEDF technology. On the other hand, there is insufficient academic exploration on the regulation strategies of PEDF technology in specific complicated systems, lacking systematic theoretical analysis and empirical verification.
1.2 Motivations and Contributions of This Paper
Given the above background, it is particularly important to further explore the low-carbon operation of PEDF parks, which simultaneously pays attention to the goal of reducing carbon emission during the energy utilization, dispatch, and management in the park. The PEDF technology can provide an innovative technological path for low-carbon operation in the park with its unique advantages. However, to achieve this goal, it is necessary to conduct in-depth research on the specific application strategies of PEDF technology in the park to ensure that the technology can maximize its benefits. Therefore, this paper aims to provide a systematic review of low-carbon operation in PEDF parks based on the current research status, analyze the problems and challenges, and propose future research directions and priorities. The research in this paper not only helps to deepen the understanding and recognition of PEDF technologies, but also provides useful references and inspirations for promoting the practical application and development of PEDF parks in the future.
Different from most review papers that usually separately introduce the progress of four key technologies of PV, ES, DC distribution, and flexible load control, this paper constructs a comprehensive theoretical framework by exploring their interaction mechanisms. This paper summarizes the methods of carbon emission monitoring and evaluation, as well as the bi-level optimal dispatch strategies for flexible loads. This not only provides a comprehensive understanding of the application of PEDF technology in specific complex systems, but also provides scientific and strategic support for the subsequent research on low-carbon operation of PEDF parks. Furthermore, from an application perspective, this paper highlights the enormous potential of PEDF technology in promoting energy structure optimization and upgrading, and achieving low-carbon development. In particular, this paper emphasizes the deep integration of intelligence and automation technology, the construction of energy Internet, the research and development of new RE technology and ES technology, and other future research directions, which provides useful reference for the practical application and future development of PEDF technology. This not only enhances the practical value of this paper, but also provides innovative ideas for the transformation and upgrading of energy systems.
In the following sections, we will first outline the basic concepts and characteristics of PEDF park systems (Section 2), then introduce current carbon emission monitoring and assessment methods (Section 3), the theory of bi-level dispatch of flexible loads (Section 4), and the innovations in low-carbon operation strategies that can be brought by advanced technologies (Section 5), and finally analyze the challenges faced and future development trends (Section 6). The outline of this paper is shown in Fig. 1. Through the analysis of these contents, we hope to provide support and guidance for the further research and application of PEDF parks.
Figure 1: Outline of this paper
2 Overview of PEDF Park Energy System
A PEDF park integrates PV power generation, ES systems, DC distribution system, and flexible load management technologies, forming an efficient, collaborative, green, and low-carbon energy ecosystem. The core feature of this park lies in its highly integrated technology system and flexible management mode. It can help to achieve self-sufficiency, efficient utilization, and flexible dispatch of energy. The concept of PEDF park emphasizes the overall optimization and sustainable development of the energy system, i.e., the system can provide energy services continuously, stably, and efficiently during long-term operation, while reducing negative impacts on the environment. This section first introduces the main components of the PEDF park, and then analyzes the role and its advantages in the energy system.
2.1 Main Components of PEDF Park Energy System
A typical PEDF park system can be described as Fig. 2. Below is a brief introduction to the main components of the system [14]:
Figure 2: Main components of the PEDF park system
• PV Generation: PV power generation is the main energy supply method for PEDF parks. It can capture solar energy and convert it into electrical energy for the park. This not only provides clean and RE, but also reduces the carbon emissions of the park. To ensure maximum power generation efficiency, PV generation is usually combined with weather forecasting and other predictive technologies.
• ES System: The ES system can store electrical energy when there is an excess of energy and release it when there is a shortage of energy, playing a role in energy buffering and balancing. In addition to serving as a backup power source, the ES system can also act as flexible load and participate in various services such as peak shaving, frequency regulation, and reactive power compensation, helping the system achieve efficient energy utilization.
• DC Distribution System: The DC distribution system is a key link in energy transmission and distribution within the PEDF park. It can adjust the flow of electrical energy according to the system status to ensure supply-demand balance. Compared to traditional AC distribution systems, DC distribution systems have higher transmission efficiency, lower energy loss, and simpler topology. Specifically, the direct current generated by PV power generation can be directly connected to the DC bus, reducing unnecessary AC/DC conversion processes and improving energy utilization efficiency.
• Loads: The energy loads of PEDF park includes adjustable flexible loads and nonadjustable nonflexible loads. Flexible loads typically include temperature controlling loads (e.g., air conditioning, boilers, water heaters) or charging piles. These flexible loads can dynamically adjust power demand based on energy supply, improving energy utilization efficiency and enhancing the energy resilience of the park.
• Operating Center: The operating center is not only the monitoring core of the entire park, but also undertakes key tasks of energy dispatch, system management, and security assurance. Specifically, the operating center monitors the power generation status of the PV system, the charging and discharging status of the ES system, and the operation status of the DC distribution system in real time through an integrated information system. At the same time, it can flexibly arrange energy resources and perform optimized energy allocation based on the energy needs of various devices in the park. The powerful data analysis capability of the operating center can deeply explore the energy usage situation in the park, providing scientific basis for energy management and optimization in the park.
Note that the batteries in electric vehicles (EV) can also serve as distributed ES devices for collaborative optimization with the PEDF park, playing a role in peak shaving and valley filling [23], smoothing grid fluctuations [24], and promoting low-carbon operation of the park [25]. In addition, there exists a close and dynamic interaction between the power grid and the PEDF park [26]. The power grid, as the backbone of energy transmission and distribution, provides necessary power supplementation and emergency support for the park. When the PV power generation in the park is insufficient or the ES system cannot meet the demand, the power grid can quickly respond and deliver electricity to the park to ensure its normal operation. On the contrary, when there is an excess of PV power generation in the park, the park can also feed back the excess electricity to the power grid, promoting the consumption of clean energy and the green transformation of the power grid.
2.2 Collaborative Interaction in PEDF Park System
The collaborative interaction between the main components of the PEDF system is the key to achieving efficient energy utilization, flexible dispatch, and optimized management. As illustrated by Fig. 3, the PEDF system has the following interactive modes simultaneously:
Figure 3: Collaborative interaction in a PEDF park system, where solid lines contain energy interaction, while dashed lines only involve information exchange
• Interaction between PV and ES: The ES system is responsible for storing the electricity generated by PVs and releasing it when nee0ded. This complementary relationship ensures a stable supply of system energy. In addition, due to the influence of weather conditions, the output of PV power generation is intermittent and fluctuating. The ES system can smooth the output of PV power generation and reduce the instability of energy supply by dynamically adjusting the charging and discharging status.
• Interaction between PV, ES, and DC distribution: The DC electricity generated by PVs can be directly transmitted through the DC distribution system, avoiding energy loss during the AC conversion process. At the same time, the system can intelligently control the charging and discharging of the ES system based on real-time energy supply and demand to ensure a balanced energy supply.
• Interaction between DC distribution and loads: The DC distribution system provides stable DC power supply for flexible loads. Due to the high requirements for power quality in flexible loads, the efficient power supply method of DC distribution systems can meet these demands and ensure the smooth operation of loads.
• System-level comprehensive interaction: With the assistance of the operation center, the PEDF system dynamically adjusts and optimizes dispatch based on real-time energy supply and demand, grid status, and electricity price signals, achieving system-level resource coordination, ensuring stable system operation, and improving energy utilization efficiency.
2.3 Advantages of PEDF Park in Energy System
The distinct operating mode and advanced technology system of the PEDF park has the potential to not only promote the optimization and upgrading of the energy structure, but also improve energy utilization efficiency. From a technical perspective, the continuous progress and innovation of PV technology, such as the research and application of efficient PV conversion materials [27,28], lay a solid foundation for the energy self-sufficiency of the park. At the same time, by adopting advanced BES technology, heat pump storage, and other methods, the ES system can ensure the continuity and reliability of energy supply in the park [29,30]. Moreover, with the support of vehicle-to-vehicle (V2V) and vehicle-to-grid (V2G) technologies, EVs are no longer just a means of transportation, but can also serve as distributed ES units that can perform flexible charging and discharging operations according to the needs of the park and the grid [31,32]. This helps to improve the flexibility and resilience of the PEDF energy system.
The practice of PEDF park benefits from the guidance of advanced concepts of energy internet and smart grid [33,34]. These theories emphasize the openness, interactivity, and intelligence of the energy system, providing a macro perspective and strategic direction for the construction of PEDF parks. In the park, by building an energy management system based on big data, cloud computing, and the Internet of things (IoT), comprehensive perception and intelligent dispatch of energy production, transmission, storage, and consumption can be achieved. This can improve the refinement level of energy management, and provide scientific basis for energy optimization in the park through data analysis and prediction.
The value of the PEDF park is reflected in its various advantages. Economically, the park can optimize energy allocation and reduce operating costs by implementing refined energy management strategies. Specifically, the energy supply and demand within the park can be accurately matched, avoiding unnecessary energy waste and thereby reducing operating costs. In terms of social benefits, PEDF park conforms to the low-carbon environmental protection concept and focuses on sustainable development. This operation model, which mainly relies on renewable energy as the main source of energy supply, can not only reduce carbon emissions and protect the ecological environment, but also shape the green image of the park. In addition, from the perspective of technological innovation, PEDF park integrates cutting-edge energy technologies and efficient management methods. These technologies cover all aspects of energy production, transmission, storage, and application, and their innovative ideas can help explore new paths for the future development of the global energy system. Therefore, with its technological advantages and benefits, the PEDF park is expected to become an important paradigm for future energy systems.
The differences between PEDF systems and common integrated energy or power systems mainly lie in technology integration, energy efficiency, and environmental protection. The PEDF system achieves deep integration of four core technologies: PV power generation technology reduces the system’s dependence on fossil fuels; ES technology ensures the stability and reliability of power supply; DC distribution technology reduces losses during power transmission and conversion; flexible load control technology enables interact with the power grid in a friendly manner. This integration can not only improve the energy utilization efficiency of the system, achieving flexible dispatch and optimized allocation of electricity, but also reduce energy waste and carbon emissions, which is friendly to the environment. However, general integrated energy or power systems often lack such deep technological integration, making it difficult to achieve the same energy utilization efficiency and environmental friendliness.
3 Carbon Emission Monitoring and Evaluation Technologies
Carbon emission monitoring mainly relies on high-precision monitoring devices and professional monitoring technologies to obtain real-time and continuous carbon emission data. While carbon emission evaluation mainly relies on quantifying, analyzing, and evaluating the carbon emission of a specific region or enterprise based on activity data and emission factors. They are fundamental and crucial in the formulation of low-carbon operation strategies for PEDF parks. This section focuses on how carbon emission monitoring and evaluation methods provide data support for regulatory strategies.
3.1 Carbon Emission Monitoring Methods
Currently, carbon emission monitoring technologies mainly include two categories: direct measurement methods and indirect estimation methods.
As one of the mainstream technologies for carbon emission monitoring, direct measurement method relies on the use of high-precision and high-sensitivity instruments and devices, such as continuous emission monitoring systems (CEMS) [35], to directly monitor emission sources like industrial chimneys or automobile exhaust in real time, ensuring the immediacy and accuracy of data acquisition. CEMS provides a solid data foundation for carbon emission regulation by analyzing the concentration and flow rate of CO2, SO2, and other components in the emitted gas, combined with calibration parameters, to calculate real-time emissions.
For the PEDF park, the direct measurement method is not only used for monitoring traditional emission sources, but also requires special attention to the cleanliness of PV panels [36], conversion efficiency [37], and indirect carbon emissions during the charging and discharging process of ES systems (e.g., emissions during battery manufacturing and maintenance) [38]. By installing high-precision environmental monitoring sensors and energy efficiency monitoring systems, dust accumulation on the surface of PV panels, temperature changes, and ES system charging and discharging efficiency can be monitored in real time, indirectly estimating the additional carbon emissions caused by efficiency loss.
The indirect estimation method is based on statistics and model prediction. This method constructs a mathematical model reflecting the relationship between carbon emissions and economic activities by collecting and analyzing key information including energy consumption data (e.g., the consumption of fossil fuels like coal, oil, and natural gas), production activity parameters (output and process parameters), and emission factors (carbon emissions per unit activity level) [39–41].
For PEDF parks, indirect estimation of carbon emissions is usually based on energy consumption and corresponding energy emission factors. The basic formula can be written as follows [42]:
CCO2=n∑i=1mi⋅EFi(1)
where CCO2 is the carbon dioxide emission of the park, mi represents the consumption of the i-th type of energy (electricity, coal, etc.), EFi represents the emission factor of the i-th energy source (carbon emissions per unit of energy consumption), and n represents the total number of energy types. In particular, these emission factors are usually considered unchanged during a short time period and can be obtained from carbon emission databases [43].
In the PEDF park, in particular, the carbon emissions of PV power generation are theoretically zero (or extremely low, only considering indirect emissions from manufacturing and maintenance), while the carbon emissions of ES systems may be reflected in their manufacturing, maintenance, and waste disposal processes, which need to be estimated through methods like life cycle assessment (LCA) [44,45]. On the one hand, although PV power generation itself does not generate carbon emissions, its efficiency directly affects the park’s dependence on fossil fuels, thereby indirectly affecting carbon emissions. By increasing the PV power generation efficiency, the dependence of the park on the external power grid can be reduced, thereby reducing carbon emissions [46]. On the other hand, although the charging and discharging efficiency of ES systems does not directly affect carbon emissions, it will affect the overall energy efficiency and the flexibility of energy management in the park [47]. Indirectly, inefficient ES systems may also lead to increased use of fossil fuels, thereby increasing carbon emissions.
In order to conduct LCA on a PEDF park, it is necessary to extensively collect data on park infrastructure, such as energy consumption for mining and production of building materials, emissions during park design and construction, as well as energy efficiency and emission information for PV power generation systems, ES systems, and distribution systems [48]. At the same time, operation and maintenance data are equally important, including energy consumption, real-time emission data, resources required for facility maintenance, and waste generated. In the stage of waste disposal, the retirement methods, energy consumption, and emission data of buildings, facilities, and equipment are also indispensable [49]. These data can be obtained through questionnaire surveys, on-site investigations, corporate reports, literature, and professional databases, and analyzed using LCA software or other professional tools to accurately assess the carbon footprint and other environmental impacts of the park [50].
3.2 Carbon Emission Evaluation Method
Commonly used carbon emission evaluation methods include LCA and input output analysis (IOA). In the construction of the carbon emission evaluation model for the PEDF park, targeted adjustments and optimizations are needed for LCA and IOA.
LCA is a systematic analytical tool that quantifies the environmental footprint of a product or service by tracking its entire process from cradle to grave, including direct and indirect emissions [51]. The construction of LCA model involves the steps of data collection, system boundary definition, and impact assessment. It can provide policy makers with a comprehensive overview of carbon emissions throughout the entire lifecycle of products or services, supporting low-carbon design and green consumption decisions.
In a PEDF park, the LCA model needs to pay special attention to the environmental impact of key components, especially PV panels [52,53] and ES batteries [54,55], throughout their entire lifecycle. This includes the processes of raw material extraction, production and manufacturing, transportation and installation, use and maintenance, and disposal. By collecting detailed environmental impact data and constructing a unique LCA database for the park, it can provide support for evaluating the overall and individual project carbon emissions of the park. Taking PV panels as an example, their lifecycle carbon emissions CLCA can be roughly expressed as:
CLCA=Cmanufacturing+Ctransportation+Cinstallation+Coperation+Cdisposal(2)
where Cmanufacturing, Ctransportation, Cinstallation, Coperation, Cdisposal are the carbon emission during the stage of manufacturing, transportation, installation, operation, and disposal respectively. The carbon emissions for each of these stages need to be calculated based on specific data and emission factors.
In contrast, IOA focuses on analyzing carbon flows and cumulative effects from a macroeconomic perspective [56]. Fig. 4 shows an example of the input and output of a PEDF park. This helps construct an input-output relationship matrix, which reflects the use of products from various sectors as production factors for other sectors This reveals the economic connections and carbon transfer pathways between sectors, and provides a powerful tool for understanding the relationship between economic structure and carbon emissions [57]. Through linear algebraic operations, the contribution of different economic activities to carbon emissions can be calculated, providing a scientific basis for formulating low-carbon economic policies.
Figure 4: Input and output of a PEDF park
The application of the IOA model in the PEDF park needs to consider the interaction between the park and external power grid. We can analyze the carbon flow and cumulative effects in the park’s economic activities by constructing an extended input-output matrix that includes ES systems, PV generation systems, and external power grids. Specifical attention should be paid to the impact of the intermittency of PV power generation and the regulation capability of ES systems on carbon emissions in the park. By simulating carbon flow paths under different operating scenarios, the potential and challenges of low-carbon operation in the park can be evaluated [58].
In IOA, the calculation of carbon emissions is usually solved through a system of linear equations. Assume that there is a simplified input-output table that includes various sectors within the park and their interactions with the external power grid. The following is a simplified mathematical formula, the core idea of which is to use the input-output relationship matrix A and carbon emission vector C to solve for the carbon emission contributions of each sector [59]:
C=E(I−A)−1Y(3)
where E is the direct carbon emission coefficient matrix, I is an identity matrix, and Y is the total output vector of each sector (or a vector containing other relevant economic indicators). Note that Eq. (3) here is a highly simplified example. In practical applications, the IOA model might be more complicated, involving the interaction of multiple sectors, products, and services.
In order to construct an input-output matrix, it is necessary to collect a large number of data on the input and output of each component in the system. These data may come from various sources, such as corporate financial statements, industry reports, or government statistical data [53]. After calculating the input-output matrix A and direct carbon emission coefficient matrix E of the PEDF system, the overall carbon emissions of the system can be calculated and key emission components can be identified, providing scientific basis for the formulation of subsequent emission reduction strategies.
3.3 Guidance on Low-Carbon Operation Based on Emission Monitoring and Evaluation
The monitoring and evaluation results of carbon emissions play a crucial role in the low-carbon operation of PEDF parks. They provide precise data support and scientific basis for energy management and emission reduction strategies in the park.
In terms of optimizing economic operation, carbon emission monitoring and evaluation results play an important role. The park can construct an economic operation optimization model with the objective function of minimizing the total operating cost, in which the cost of user carbon emissions is an important component [60–62]. For example, this model can be probably represented as:
minZ=m∑i=1ciXi+λCCO2(4)
where Z represents the total operating cost of the park, ci represents the unit operation cost of the i-th type of energy, Xi represents the consumption of the i-th type of energy, λ is the carbon emission cost coefficient, and CCO2 is the total carbon emissions of the park. By solving this model, the park can obtain the optimal output scheme of each device and the optimal dispatching strategy of user load, so as to realize the dual optimization of low-carbon operation and economy.
Carbon emission monitoring data can reveal the contribution of the source and load sides to carbon emissions, thereby guiding the park to take more scientific and reasonable measures in energy dispatch and demand response (DR) [63,64]. For example, by adjusting the output of PV power generation and ES batteries, as well as optimizing user load demand, the park can achieve the minimization of carbon emissions. The model aims to minimize the overall cost of users as the objective function, where the carbon emission cost is an important component. By solving this model, the park can guide users to participate in DR so as to further reduce carbon emissions.
4 Introduction of Bi-Level Optimal Dispatch for Flexible Loads in the PEDF Park
In the comprehensive energy management system of the PEDF park, flexible load, as a crucial flexible resource, plays an indispensable role in promoting the low-carbon and efficient operation of the park. The flexible load resources within the park include fast response EV charging stations, ES systems, as well as relatively slow response adjustable production devices, building lighting, and air conditioning (AC) systems, all of which demonstrate extremely high regulation potential and flexibility. In order to maximize the flexibility of utilizing these adjustable load resources, a bi-level optimal dispatch strategy is usually considered for the PEDF park [65–67].
Fig. 5 shows an example of the theoretical framework of bi-level optimal dispatch strategies for adjustable load regulation in the PEDF park system. The first-level dispatch is mainly responsible for system level (or macro level) resource management and load allocation, while the second-level dispatch focuses on user side or microgrid level (micro level) specific load control and optimization [68,69]. This layered design helps simplify the dispatch process, improve dispatch efficiency, and enhance system flexibility and response speed.
Figure 5: Bi-level optimal dispatch framework for flexible loads in the PEDF park
4.1 First-Level Dispatch: System Level Optimization
The core of the first-level dispatch is the optimal allocation based on PV power generation and load forecasting technology, as well as ES system status management. In addition, the model may also integrate short-term and medium-term prediction data of PV power generation, which is obtained by combining physical models (e.g., solar radiation intensity [70]) with statistical learning algorithms (e.g., time series regression [71] and random forest [72]). The charging and discharging status information of the ES system can also be taken into consideration. Its dynamic changes can be described through state space models [73] or Markov chains [74].
Based on this, the first-level dispatch needs to solve a supply-demand balance problem that includes multiple time periods. The optimization objectives should at least include the minimization of electricity costs and carbon emission costs. The cost of electricity consumption depends on the net load of the park (considering the adjustment amount of flexible load, the charging and discharging power of the ES system, or the amount of PV power generation curtailment), while the cost of carbon emissions can be calculated based on the carbon emission monitoring or evaluation model in the previous subsection [14]. In addition, the constraints of the model need to consider the supply-demand balance of the park system, the charging and discharging restrictions of the ES system, the adjustment range of flexible loads, the curtailment restrictions of PV power generation, etc.
4.2 Second-Level Dispatch: User Side or Microgrid Level Optimization
The second-level dispatch serves as a refined regulation link at the user side or microgrid level in the energy management system of the PEDF park. It receives the macro load allocation plan issued by the first-level dispatch and combines real-time operating data of each load point (e.g., power consumption, switch status, and adjustable range) to adopt a more flexible intelligent control strategy [75]. In this case, the second-level dispatch can quickly respond to load fluctuations or prediction errors, dynamically adjust load allocation using feedback control mechanisms, and ensure the stability and economy of system operation. For example, when it is predicted that PV power generation will exceed demand, the second-level dispatch can prioritize increasing the consumption of flexible loads or activating the charging mode of ES systems to maximize the utilization of RE [76].
In the process of formulating load regulation strategies, the optimization approach of “peak shaving and valley filling” is usually considered [77]. The core of this strategy is to adjust users’ electricity consumption behavior, transfer the load from peak hours to off peak hours, optimize load distribution, reduce the peak valley difference, and reduce dependence on traditional fossil fuels [78]. In the specific implementation process, a series of personalized load adjustment plans can be developed based on users’ electricity habits and the energy policies of the park, such as setting the temperature range of the intelligent temperature control system [79] and adjusting the charging time of EVs [80]. These measures can not only ensure the electricity demand of users, but also improve energy utilization efficiency and user satisfaction.
4.3 Implementation Steps of the Bi-Level Dispatch
The implementation process of the bi-level dispatch strategy for adjustable load could include the following steps [69,81]:
(1) PV forecasting, load forecasting, and ES optimization: Based on historical data and forecasting models, formulate PV forecasting, load forecasting, and ES system optimization model and solve the optimal dispatch strategy.
(2) First-level dispatch: Based on the forecasting results and the status of the ES system, develop a load allocation plan and distribute it to each user or microgrid.
(3) Second-level dispatch: Each user or microgrid controls and optimizes specific loads based on the load allocation plan issued by the first-level dispatch, combined with the actual operating conditions and adjustable potential of their respective loads.
(4) Real-time monitoring and dynamic adjustment: Monitor the operating status of the load in real time, and dynamically adjust based on real-time data to ensure the accuracy and efficiency of load control.
In order to improve the performance of the two-level dispatch strategy for flexible loads, the algorithm can be optimized through parameter adjustment. By finely adjusting the key parameters in the algorithm, the effectiveness of the dispatch strategy can be further optimized. For example, in a load allocation plan, the weight or priority of load allocation can be adjusted based on the characteristics and demands of different loads [82,83].
Existing works have proposed numerous bi-level dispatch methods for flexible loads. According to different regulatory measures and mechanisms, these dispatch methods can be roughly divided into four categories: price-based guidance, DR incentive, source-load collaboration, and integrated energy system (IES) optimization. Table 1 provide a comparison between these methods, where their advantages and disadvantages are also described.
4.4 Typical Evaluation Indicators for Bi-Level Dispatch
In the PEDF park system, the bi-level dispatch of flexible loads is a key strategy to achieve efficient energy utilization, ensure stable system operation, and promote low-carbon development. To evaluate the implementation effectiveness of this strategy, a series of scientific and comprehensive evaluation indicators need to be adopted. We will provide a detailed introduction to the commonly used evaluation indicators for the bi-level dispatch of flexible loads in the PEDF park system.
Energy efficiency is an important indicator for measuring the energy utilization efficiency of the PEDF park system. It is defined as the ratio of the useful energy output by the system to the total energy input by the system [84]:
REE=EoutEin(5)
where REE is the energy efficiency ratio, Ein is represents the total energy input to the system (including renewable energy generation, energy storage and discharge, etc.), and Eout indicates the useful energy output by the system (i.e., the energy that can be utilized by load devices).
In the bi-level dispatch of flexible loads, by reasonably arranging the switching time and power allocation of loads, renewable energy can be maximally utilized for power generation and energy waste can be reduced. The improvement of energy efficiency is therefore one of the key indicators to measure the effectiveness of dispatch strategies. Generally, the higher the energy efficiency ratio, the higher the energy utilization efficiency of the system and the smaller the impact on the environment.
4.4.2 Penetration Rate of Renewable Energy
The penetration rate of RE refers to the proportion of RE generation in the total power generation in the PEDF park system, calculated by [85]:
RREP=EreEre+Eother×100%(6)
where RREP is the RE penetration rate, Ere is the amount of RE generation, and Eother is the power generation of other possible energy generation (such as fossil fuels).
This indicator reflects the degree of dependence of the system on RE in its energy structure. Therefore, the increase in the penetration rate of RE is an important indicator for measuring the effectiveness of the system in energy transition and low-carbon development. A higher RE penetration rate means a reduction in the system’s dependence on fossil fuels, which helps to reduce carbon emissions and achieve sustainable development. In the bi-level dispatch of flexible load, by accurately predicting the power generation and load demand of RE, the charging and discharging strategies of the ES system can be optimized to ensure the full utilization of RE generation.
System reliability is an important indicator for measuring the stable operation and ability to respond to unexpected situations of PEDF park systems. In the bi-level dispatch of flexible loads, system reliability can be evaluated through multiple dimensions, including the stability of load supply, and the fluctuation rates of voltage and frequency.
The stability of load supply can be measured by the load satisfaction rate [86], which is the ratio of the actual load supplied by the system to the energy demand of load:
RLS=LrealLdemand×100%(7)
where RLS is the load satisfaction rate, Lreal is the actual load supplied, and Ldemand is the estimated energy demand of load.
The fluctuation rate of grid voltage and frequency can be evaluated as follows [87]:
δU=NU∑i=1(Ui−UrefUref)2(8)
δf=Nf∑i=1(fi−freffref)2(9)
where δU and δf are the fluctuation rates of voltage and frequency, NU and Nf are the numbers of sample points of voltage and frequency, Ui and fi are the voltage and frequency of the i-th sample point, and Uref and fref are the reference values of voltage and frequency.
By optimizing dispatch strategies, the PEDF system can ensure stable operation during peak load periods and fluctuations in RE generation, thereby improving power supply quality and reliability. Focusing on improving system reliability can help reduce energy losses and enhance user satisfaction and trust.
4.4.4 Levelized Cost of Electricity
Levelized cost of electricity (LCOE) is an important indicator for measuring the economic efficiency of PEDF park systems. It represents the average cost per kilowatt hour of electricity produced by the system throughout its entire lifecycle. The calculation can be written as [88–90]:
LCOE=∑Tt=1Ct(1+r)t−1+Cinit−Sresale∑Tt=1Et(1+r)t−1(10)
where T is the system lifespan (usually measured in years), Ct is the operation and maintenance cost in the t-th year, r is a fixed discount rate, Cinit is the initial investment cost, Sresale is the possible residual value at the end of the lifespan, and Et is the amount of electricity generated by the system in the t-th year.
A lower LCOE means that the system is more competitive in terms of economic benefits, which helps attract more investment and promote the widespread application of the PEDF technology. In the bi-level dispatch of flexible loads, optimizing load allocation, ES charging and discharging strategies, and utilizing RE generation can reduce the operating costs and energy waste of the system, thereby reducing LCOE.
5 Innovation of Low-Carbon Operation Strategies Brought by Advance Technologies
To improve the intelligence level, enhance the ability to cope with uncertainties, and promote the efficient utilization of energy, a series of new technologies can be introduced and applied. This section will analyze the innovation and opportunities brought by the application of these technologies to the low-carbon operation of PEDF parks.
5.1 Advanced Technologies of Sensors, Communication, and Internet of Things
In the low-carbon operation of the PEDF park system, advanced sensors and communication technology constitute an indispensable key infrastructure. The introduction of these technologies will greatly improve the intelligence and refinement level of the energy management system in the park.
High-precision and low-power sensors has been widely used in real-time monitoring of PV panels [91], ES batteries [92], distribution systems [93], and flexible loads [94]. These sensors adopt advanced sensing principles and signal processing technologies. They can collect real-time and accurate operational status data of the system, including but not limited to sunlight intensity, temperature, voltage, current, power factor, etc. Taking PV panels as an example, high-precision sensors can monitor the surface light intensity and temperature distribution in real time, thereby accurately calculating the power generation efficiency and output power of the PV panels [91]. Similarly, sensors on ES batteries can monitor voltage, current, temperature, and remaining capacity, providing scientific basis for battery charging and discharging strategies [92]. The sensors on the DC distribution system and flexible loads are responsible for monitoring the voltage stability and current fluctuations of the distribution network, and power demand of loads, ensuring the stable operation of the system and efficient utilization of energy [93,94].
On the basis of data collection, high-speed and low latency communication networks ensure real-time transmission and processing of data. These communication networks adopt advanced communication protocols and transmission technologies, such as low-power wide area network communication technologies (e.g., Zigbee [95], LoRa [96], and NB-IoT [97]), as well as high-speed communication technologies (e.g., 5G [98] and optical fiber [99]), which can achieve seamless connection and efficient data transmission between sensors and central control units. Through these communication networks, the data collected by sensors can be transmitted in real-time to the operating center for processing and analysis, providing strong support for the timely execution and feedback of control strategies. At the same time, these communication networks can also support remote access and sharing of data, enabling the energy management system of the park to exchange data with other systems, further enhancing the interconnectivity of the system.
The Internet of things (IoT) technology can build a massive data network by connecting various devices, sensors, and controllers within the park, providing real-time and accurate data support for energy management in the park [100]. Based on the massive data collected through IoT technology, the PEDF system can maximize its energy utilization. IoT technology can further facilitate real-time data processing and analysis through the combination of edge computing and cloud computing. Edge computing reduces data transmission delay and bandwidth occupation by preliminarily processing and filtering data on the device side or in the local network [101]; Cloud computing is responsible for the storage, analysis, and optimization of global data, providing powerful computing capabilities for intelligent decision-making in the system [102]. This combination of edge computing and cloud computing can not only improve the response speed and energy efficiency of the system, but also provide a more intelligent and refined solution for the energy management of the park.
5.2 Artificial Intelligence and Machine Learning
Artificial intelligence (AI) and machine learning (ML) technologies, as outstanding representatives of modern information technology, can bring revolutionary changes to energy management in PEDF parks.
In the PEDF park, AI technology can capture and analyze the data of the complicated system dynamics in real time through deep learning algorithms. In more detail, AI algorithms can predict energy supply and demand trends through comprehensive analysis of these data, providing scientific basis for the formulation of regulatory strategies. For example, using deep learning models such as long short-term memory (LSTM) network [103] or convolutional neural network (CNN) [104], AI can predict the PV power generation and load demand in the future for a period of time, so as to adjust the charging and discharging strategy of ES batteries in advance to ensure the stability and efficiency of energy supply.
At the same time, ML algorithms also play a crucial role in the low-carbon operation of PEDF park systems. ML algorithms can mine the operating rules of systems from historical data, continuously optimize control models, and achieve more flexible and accurate control. This data-driven optimization method can not only improve energy efficiency, but also significantly reduce operation and maintenance costs [105]. Specifically, ML algorithms can model and train historical data through clustering analysis, regression analysis, or classification prediction, in order to obtain the optimal operating strategy of the system under different conditions [106–108]. Through continuous learning and optimization, AI and ML algorithms can gradually adapt to changes and uncertainties in the system, achieving more accurate and efficient energy management, which not only enhances energy utilization efficiency and reduces operation and maintenance costs, but also provides strong guarantees for the sustainable development of the park.
Blockchain technology, with its unique features of decentralization, transparency, security, and immutability [109], is gradually demonstrating its enormous potential for energy management and trading in PEDF parks. The introduction of this technology not only provides a more efficient, secure, and transparent platform for energy trading in the park, but also provides strong technical support for the implementation and supervision of low-carbon operation strategies [110].
First of all, blockchain technology can build a distributed and highly trusted energy trading platform for energy producers, consumers, and operators within the PEDF park. This platform is based on the decentralized nature of blockchain, realizing peer-to-peer trading and settlement of energy, avoiding the centralized bottleneck and information asymmetry problems in traditional energy trading [111]. On the blockchain, every energy transaction is recorded as an immutable block, ensuring transparency and traceability of the transaction. Meanwhile, through the application of encryption algorithms and consensus mechanisms, blockchain technology also ensures the security of transaction data, preventing data leakage and tampering [112].
The application of blockchain technology can also play a profound role in low-carbon operation regulation. Through blockchain technology, energy data within the park can be accurately and completely recorded, verified, and traced. Specifically, each piece of operational data can be uploaded in real-time to the blockchain, forming a complete energy data chain [113]. These data not only provide scientific basis for the formulation of low-carbon operation and regulation strategies, but also provide strong data support for the efficient utilization of energy and low-carbon transformation. Meanwhile, the immutability of blockchain technology ensures the authenticity and reliability of these data [114], which can provide a more accurate and comprehensive information foundation for energy management in the park.
In addition, blockchain technology also supports smart contracts that can further promote the automation and compliance of energy transactions [115]. Smart contract is an automated contract based on blockchain technology, whose terms and logic are written in code form and embedded on the blockchain. When specific conditions are met, smart contracts will automatically execute without human intervention [116]. In the energy trading of the PEDF Park, smart contracts can set transaction price, transaction quantity, and transaction time, and automatically monitor the performance of both parties in the transaction. Once the transaction is completed, the smart contract will automatically settle and make payments, greatly improving the efficiency and compliance of the transaction. Meanwhile, the application of smart contracts can also reduce transaction costs and risks, providing a more convenient and secure solution for energy trading in the park [117].
6 Challenges and Future Prospects
Current research on PEDF park systems is facing multiple challenges in terms of low-carbon operation. This section will delve into the causes of these challenges and their impact on system development. At the same time, this section will also explore how to address these challenges and look forward to future research directions and priorities, in order to promote the greater role of the PEDF park system in low-carbon development.
6.1 Current Research Challenges
In the exploration low-carbon operation strategies for PEDF park systems, related research has encountered a series of complicated and multidimensional challenges.
The integration and compatibility of PEDF technology is the primary challenge [22]. The effective integration of PV, ES, DC distribution, and flexible energy utilization technologies is the key to achieving efficient system operation [118]. However, there is a lack of unified technical standards and interface specifications, which makes compatibility between different components a major challenge. In addition, the integration of large-scale distributed RE poses a severe challenge to the stability of the power system [119]. How to effectively cope with the intermittency and uncertainty of RE while ensuring the stable operation of the system is a technical challenge. This requires researchers to not only explore more advanced regulation strategies, but also make breakthroughs in improving the performance of key components such as PV panels, ES devices, and flexible control devices, which directly affect the overall performance of the PEDF park system [120].
Note that the main objective of implementing the PEDF park system is to achieve low-carbon operation. The balance between environmental protection and energy utilization optimization requires researchers not only to have a deep understanding of the mechanisms of energy supply and demand, but also to be able to develop regulation algorithms that can adapt to complex and changing energy environments [121]. Faced with multiple challenges such as the occurrence of supply-demand contradictions [122], peak-valley contradictions [123], or volatility contradictions [124], how to design regulatory strategies that ensure system stability and achieve low-carbon goals will become a difficult research topic.
At the economic level, the construction and operation costs of the PEDF park system can be relatively high, including device procurement, installation and commissioning, operation and maintenance expenses. In order to reduce these costs and enhance the economic feasibility of the system, it is necessary to improve the overall economic benefits of the system through technological innovation and cost control strategies [125]. At the same time, it is necessary to explore more reasonable green finance credit models to support the construction and operation of PEDF parks. However, the current green finance credit market is not yet perfect, with problems such as difficult and expensive financing [126]. This requires researchers not only to address technological challenges, but also to pay attention to changes in the economy, market, and policies.
6.2 Future Research Directions
In response to the above challenges, this paper will then conduct a prospective analysis of future research directions and priorities.
First of all, the deep integration of intelligence and automation technologies is the key to promoting the low-carbon operation of the PEDF park system. Future research will focus more on deeply integrating these advanced technologies into the core processes of system prediction, dispatch, and control, aiming to achieve efficient energy utilization and carbon emissions reduction. For example, by using deep learning algorithms to make more accurate predictions of energy generation and consumption [127], combined with IoT technology to achieve seamless connections and efficient collaboration between devices [128], it can enhance the intelligence level and response speed of the system. Meanwhile, to address the challenges of technology compatibility, future research will explore unified standards and interfaces to ensure smooth integration between different components. In response to the instability of RE, intelligent adaptive control strategies will be explored to enhance system resilience.
The construction of energy Internet will become another important direction of future research. With the combination of power network, information network, transportation network, and so on, energy Internet is gradually becoming a bridge connecting various energy facilities and users [129]. Future research will be committed to building a more complete energy Internet system to promote the deep integration and efficient interaction between the PEDF park system and the regional energy market. This includes optimizing the information flow and energy flow in the energy Internet [130], improving the overall synergy and flexibility of the system [33], and exploring how to use the energy Internet platform to carry out innovative power trading and ancillary services [131,132]. At the same time, future study will also focus on how to promote the cooperation between market mechanism and policy improvement for future PEDF park systems.
The research and development of new ES technologies and RE technologies are also full of potential. With the advancement of technology and the continuous reduction of costs, new ES technologies, such as flow batteries [133] and solid-state batteries [134], may gradually replace traditional ES methods, providing more reliable and efficient RE solutions for PEDF parks. Meanwhile, the innovation of RE technologies will also provide more high-quality energy supply for the system, further reducing carbon emissions. To address economic challenges, future research will closely monitor the latest developments in these technologies and explore how to better apply them to the PEDF park system to achieve more low-carbon and efficient energy utilization [135]. The breakthrough in this field will inject new vitality into the development of PEDF park systems, promoting its continuous progress towards an economical, efficient, and sustainable direction.
Based on the scenario of the PEDF park system, this paper first analyzes the methods of carbon emission monitoring and evaluation, as well as the two-level optimization dispatch strategy for flexible loads, then explores the technological innovation opportunities for the low-carbon operation of the park, and finally summarizes the challenges and future research directions faced by related research. This paper aims to summarize existing research results, explore future research directions, and provide theoretical support and practical guidance for the low-carbon development of PEDF parks.
After investigating on related works of low-carbon operation in PEDF parks, we extract several innovative insights and suggestions for the development of this field. First, for the low-carbon operation of PEDF park, we advocate deeper excavation of the “source-network-load-storage” collaboration mechanism. Unlike the approaches of isolating the performance of each component in some existing papers, we recommend a global perspective to achieve smooth and efficient integration between key components. Second, in carbon emission monitoring and assessment, we emphasize the construction of a comprehensive monitoring system that covers the entire lifecycle. Methods such as LCA or IOA are recommended to examine the carbon footprint of PEDF parks from a broader perspective. Last, different from traditional dispatch that primarily pursues economic benefits, the dispatch of PEDF systems focuses more on ensuring load demand while maximizing the utilization of RE through refined management.
PEDF Park, with its advantages of high efficiency, low cost, and flexibility, helps optimize the energy structure and promote low-carbon development, and has enormous potential for application. In the future, the integration of technological progress and intelligence will drive innovation in PEDF and provide support for building an efficient energy system.
Acknowledgement: Not applicable.
Funding Statement: This work was supported by National Key R&D Program of China for International S&T Cooperation Projects (Grant No. 2019YFE0118700), which was provided by the Ministry of Science and Technology of the People’s Republic of China (https://www.most.gov.cn/ (accessed on 1 January 2025)), and the grant was received by Yun Zhao. This work was supported by Science and Technology Project of CSG Electric Power Research Institute (Grant No. SEPRIK23B052), which was provided by CSG Electric Power Research Institute (http://www.sepri.csg.cn/ (accessed on 1 January 2025)), and the grant was received by Ziwen Cai.
Author Contributions: The authors confirm contribution to the paper as follows: Study conception and design: Ziwen Cai, Yun Zhao; Data collection: Zongyi Wang; Analysis and interpretation of results: Yunfeng Li; Draft manuscript preparation: Tonghe Wang, Hao Wang. All authors reviewed the results and approved the final version of the manuscript.
Availability of Data and Materials: The raw data supporting the conclusion of this article will be made available by the authors with reasonable request.
Ethics Approval: Not applicable.
Conflicts of Interest: The authors declare no conflicts of interest to report regarding the present study.
Nomenclature
AC | Air Conditioning |
AI | Artificial Intelligence |
BES | Battery Energy Storage |
CEMS | Continuous Emission Monitoring System |
CNN | Convolutional Neural Network |
DC | Direct Current |
DR | Demand Response |
ES | Energy Storage |
EV | Electric Vehicle |
GA | Genetic Algorithm |
IES | Integrated Energy System |
IOA | Input Output Analysis |
IoT | Internet of Things |
LCA | Life Cycle Assessment |
LCOE | Levelized Cost Of Electricity |
LSTM | Long Short-Term Memory |
ML | Machine Learning |
MOEA/D | Multi-Objective Evolutionary Algorithm/Decomposition |
MPPT | Maximum Power Point Tracking |
NN | Neural Network |
NSGA-II | Non-Dominated Sorting Genetic Algorithm II |
PEDF | Photovoltaic, Energy storage, Direct current, and Flexible load |
PSO | Particle Swarm Optimization |
PV | Photovoltaic |
PV-ES | Photovoltaic and Energy Storage |
RE | Renewable Energy |
RF | Random Forest |
RL | Reinforcement Learning |
SVM | Support Vector Machine |
SVR | Support Vector Regression |
V2G | Vehicle-to-Grid |
V2V | Vehicle-to-Vehicle |
References
1. Xu C, Xiao X, Chen H. A novel method for forecasting renewable energy consumption structure based on compositional data: evidence from China, the USA, and Canada. Environ Dev Sustain. 2024;26(2):5299–333. doi:10.1007/s10668-023-02935-5. [Google Scholar] [CrossRef]
2. Lu H, Pan X, Zhou Y, Wang X, Huang Q, Cai D. Research status and prospect of energy storage technology in PEDF building. In: 2024 6th Asia Energy and Electrical Engineering Symposium (AEEES); 2024 Mar 28–31; Chengdu, China. p. 1011–6. doi:10.1109/AEEES61147.2024.10544781. [Google Scholar] [CrossRef]
3. Su X, Yu R, Zhang Z, Ma Y, Chao H, Lv Z, et al. Research on the methodology on optimal operation for PEDF systems in low-carbon emission buildings scenario. In: 2024 3rd International Conference on Energy and Electrical Power Systems (ICEEPS); 2024 Jul 14–16; Guangzhou, China. p. 40–4. doi:10.1109/ICEEPS62542.2024.10693255. [Google Scholar] [CrossRef]
4. Xiao D, Liu T. Optimized photovoltaic system for improved electricity conversion. Int J Low Carbon Technol. 2022;17:456–61. doi:10.1093/ijlct/ctab103. [Google Scholar] [CrossRef]
5. Kim S, An J, Choi H, Hong T. Assessment the technical and economic performance of a window-integrated PV system using third-generation PV panels. Energy Build. 2023;286(3):112978. doi:10.1016/j.enbuild.2023.112978. [Google Scholar] [CrossRef]
6. Vahdatikhaki F, Salimzadeh N, Hammad A. Optimization of PV modules layout on high-rise building skins using a BIM-based generative design approach. Energy Build. 2022;258(17):111787. doi:10.1016/j.enbuild.2021.111787. [Google Scholar] [CrossRef]
7. Jaga OP, GhatakChoudhuri S. A smart control for self-reliant single-phase, grid-tied photovoltaic-battery storage system. Sustain Energy Technol Assess. 2023;57(11):103269. doi:10.1016/j.seta.2023.103269. [Google Scholar] [CrossRef]
8. Alharbi AG, Olabi AG, Rezk H, Fathy A, Ali Abdelkareem M. Optimized energy management and control strategy of photovoltaic/PEM fuel cell/batteries/supercapacitors DC microgrid system. Energy. 2024;290:130121. doi:10.1016/j.energy.2023.130121. [Google Scholar] [CrossRef]
9. Chen S, Lin F, Zhao X, Li H. Optimal configuration method of photovoltaic energy storage in distribution network based on source-load coordination. J Phys: Conf Ser. 2024;2826(1):012035. doi:10.1088/1742-6596/2826/1/012035. [Google Scholar] [CrossRef]
10. Zhu JH, Ren H, Gu J, Zhang X, Sun C. Economic dispatching of Wind/photovoltaic/storage considering load supply reliability and maximize capacity utilization. Int J Electr Power Energy Syst. 2023;147(1):108874. doi:10.1016/j.ijepes.2022.108874. [Google Scholar] [CrossRef]
11. Jia K, Shi Z, Wang C, Li J, Bi T. Active converter injection-based protection for a photovoltaic DC distribution system. IEEE Trans Ind Electron. 2022;69(6):5911–21. doi:10.1109/TIE.2021.3086726. [Google Scholar] [CrossRef]
12. Hu D, Peng Y, Wan K, Wang X, Wei W. An improved distributed secondary control for multi-voltage-level DC distribution networks with virtual impedance arrangement. IET Power Electron. 2023;16(7):1169–80. doi:10.1049/pel2.12460. [Google Scholar] [CrossRef]
13. Becker JKM, Zuo Y, Paolone M, Kettner AM. Harmonic power-flow study of hybrid AC/DC grids with converter-interfaced distributed energy resources. In: 2023 IEEE Belgrade Power Tech; 2023 Jun 25–29; Belgrade, Serbia. p. 1–6. doi:10.1109/PowerTech55446.2023.10202734. [Google Scholar] [CrossRef]
14. Chen W, Zhao Y, Wu X, Cai Z, Guo M, Lu Y. Stackelberg game-based optimal dispatch for PEDF park and power grid interaction under multiple incentive mechanisms. Energy Eng. 2024;121(10):3075–93. doi:10.32604/ee.2024.051404. [Google Scholar] [CrossRef]
15. Rahimi M, Ardakani FJ, Olatujoye O. Improving flexible optimal scheduling of virtual power plants considering dynamic line rating and flexible supply and demand. Int J Electr Power Energy Syst. 2023;150(4):109099. doi:10.1016/j.ijepes.2023.109099. [Google Scholar] [CrossRef]
16. Tan Z, Chen Y, Fei W, Zhou N, Yang Y, Shen X. Multi-function collaborative flexible source-load intelligent interactive operation control technology for industrial communities. J Electr Syst. 2024;20(2):653–62. doi:10.52783/jes.1218. [Google Scholar] [CrossRef]
17. Liu C, Dai J, Duan C, Xue X. Simulation and analysis of fault characteristics of PEDF building energy systems. In: 2023 6th Asia Conference on Energy and Electrical Engineering (ACEEE); 2023 Jul 21–23; Chengdu, China. p. 354–8. doi:10.1109/ACEEE58657.2023.10239612. [Google Scholar] [CrossRef]
18. Yin J, Lang X, Duan J, Liang S, Li X. Interruption characteristics of self-powered hybrid DC circuit breaker with synergistic effect of step-down and divided current. Int J Electr Power Energy Syst. 2024;159(8):109989. doi:10.1016/j.ijepes.2024.109989. [Google Scholar] [CrossRef]
19. Chen S, Cai D, Luo S, Lu H, Li J, Huang Q. Technology review of DC power distribution system in PEDF buildings. In: 2024 6th Asia Energy and Electrical Engineering Symposium (AEEES); 2024 Mar 28–31; Chengdu, China. p. 963–8. doi:10.1109/AEEES61147.2024.10544965. [Google Scholar] [CrossRef]
20. Lu H, Pan X, Zhou Y, Chen S, Huang Q, Cai D. DC power distribution system architecture of PEDF buildings: a comprehensive review. In: 2024 6th Asia Energy and Electrical Engineering Symposium (AEEES); 2024 Mar 28-31; Chengdu, China. p. 986–91. doi:10.1109/AEEES61147.2024.10544555. [Google Scholar] [CrossRef]
21. Ye Z, Cai D, Luo S, Lu H, Li J, Huang Q. A review of coordinated control of DC microgrids in PEDF buildings. In: 2024 6th Asia Energy and Electrical Engineering Symposium (AEEES); 2024 Mar 28–31; Chengdu, China. p. 721–6. doi:10.1109/AEEES61147.2024.10544841. [Google Scholar] [CrossRef]
22. Liu X, Liu X, Jiang Y, Zhang T, Hao B. Photovoltaics and energy storage integrated flexible direct current distribution systems of buildings: definition, technology review, and application. CSEE J Power Energy Syst. 2023;9(3):829–45. doi:10.17775/CSEEJPES.2022.04850. [Google Scholar] [CrossRef]
23. Xie T, Su Y, Zhang G, Zhang K, Li H, Wang R. Optimizing peak-shaving cooperation among electric vehicle charging stations: a two-tier optimal dispatch strategy considering load demand response potential. Int J Electr Power Energy Syst. 2024;162:110228. doi:10.1016/j.ijepes.2024.110228. [Google Scholar] [CrossRef]
24. İnci M, Bayındır KÇ. Single-stage vehicular fuel cell system with harmonic elimination capability to suppress distortion effects of electric vehicle parking lots. J Power Sources. 2024;597(2):234175. doi:10.1016/j.jpowsour.2024.234175. [Google Scholar] [CrossRef]
25. Guo D, Li J, Zhang S, Liu R, Sun F, Zhang H, et al. Cooperative operation strategy of electric vehicle and photovoltaic power station considering carbon reduction benefit under dynamic electricity price. Environ Sci Pollut Res Int. 2023;30(40):92922–36. doi:10.1007/s11356-023-28886-y. [Google Scholar] [PubMed] [CrossRef]
26. Wang F, Yan J, Zhang B, Huang G, Zou X, Li Z. Research on grid-friendly PEDF building power distribution system. In: CIRED 2022 Shanghai Workshop; 2022 Sep 21–22; Shanghai, China. [Google Scholar]
27. Akshayveer, Kumar A, Singh AP, Singh OP. Effect of new overhead phase change material enclosure designs on thermo-electric performance of a photovoltaic panel. J Energy Storage. 2022;46(3):103814. doi:10.1016/j.est.2021.103814. [Google Scholar] [CrossRef]
28. Chen J, Zhao H, Li Z, Zhao X, Gong X. Highly efficient tandem luminescent solar concentrators based on eco-friendly copper iodide based hybrid nanoparticles and carbon dots. Energy Environ Sci. 2022;15(2):799–805. doi:10.1039/D1EE02554F. [Google Scholar] [CrossRef]
29. Iwata F, Fujimoto Y, Hayashi Y. Residential battery storage system sizing for the medically vulnerable from the life continuity planning perspective: toward economic operation using uncertain photovoltaic output. IEEJ Transactions Elec Engng. 2022;17(6):833–46. doi:10.1002/tee.23572. [Google Scholar] [CrossRef]
30. Saleem A, Ambreen T, Ugalde-Loo CE. Energy storage-integrated ground-source heat pumps for heating and cooling applications: a systematic review. J Energy Storage. 2024;102:114097. doi:10.1016/j.est.2024.114097. [Google Scholar] [CrossRef]
31. İnci M, Büyük M, Özbek NS. Sliding mode control for fuel cell supported battery charger in vehicle-to-vehicle interaction. Fuel Cells. 2022;22(5):212–26. doi:10.1002/fuce.202200105. [Google Scholar] [CrossRef]
32. Tian P, Yan S, Pan B, Shi Y. Two-stage optimization for efficient V2G coordination in distribution power system. In: 2024 IEEE International Conference on Communications, Control, and Computing Technologies for Smart Grids (SmartGridComm); 2024 Sep 17–20; Oslo, Norway. p. 245–51. doi:10.1109/SmartGridComm60555.2024.10738072. [Google Scholar] [CrossRef]
33. Zhang C, Liu S. Meta-energy: when integrated energy Internet meets metaverse. IEEE/CAA J Autom Sin. 2023;10(3):580–3. doi:10.1109/JAS.2023.123492. [Google Scholar] [CrossRef]
34. Odamov U, Makhammadiev F. Advantages of smart grid over conventional integrated electrical system (IES). In: AIP Conference Proceedings; 2024; Namangan, Uzbekistan: AIP Publishing. doi:10.1063/5.0197781. [Google Scholar] [CrossRef]
35. Tang L, Jia M, Yang J, Li L, Bo X, Min Z. Chinese industrial air pollution emissions based on the continuous emission monitoring systems network. Sci Data. 2023;10(1):153. doi:10.1038/s41597-023-02054-w. [Google Scholar] [PubMed] [CrossRef]
36. Tawalbeh M, Al-Othman A, Kafiah F, Abdelsalam E, Almomani F, Alkasrawi M. Environmental impacts of solar photovoltaic systems: a critical review of recent progress and future outlook. Sci Total Environ. 2021;759(4):143528. doi:10.1016/j.scitotenv.2020.143528. [Google Scholar] [PubMed] [CrossRef]
37. Li R, Meng T, Song G, Huang D, Hu S, Jiang L, et al. A GIS-based assessment of the carbon emission reduction potential of the solar-enhanced char-cycling biomass pyrolysis process in China. Renew Energy. 2024;237:121496. doi:10.1016/j.renene.2024.121496. [Google Scholar] [CrossRef]
38. Beuse M, Steffen B, Dirksmeier M, Schmidt TS. Comparing CO2 emissions impacts of electricity storage across applications and energy systems. Joule. 2021;5(6):1501–20. doi:10.1016/j.joule.2021.04.010. [Google Scholar] [CrossRef]
39. Pan J, Yang Z, Wei X, Zhou Z. A real-time estimation method of carbon emission for cement corporations based on load identification. J Phys: Conf Ser. 2024;2814(1):012008. doi:10.1088/1742-6596/2814/1/012008. [Google Scholar] [CrossRef]
40. Wang S, Wang L, Peng B, Liu Y, Ma W. Research on carbon emission measurement techniques for accounting and grid auxiliary services. J Phys: Conf Ser. 2024;2876(1):012021. doi:10.1088/1742-6596/2876/1/012021. [Google Scholar] [CrossRef]
41. Tang Y, Li Y, Yuan X, Pimm A, Cockerill TT, Wang Q, et al. Estimation of emission factors from purchased electricity for European countries: impacts on emission reduction of electricity storage. Environ Sci Technol. 2022;56(8):5111–22. doi:10.1021/acs.est.1c06490. [Google Scholar] [PubMed] [CrossRef]
42. Kong Y, Liu S. Spatio-temporal evolution and driving factors of carbon dioxide emissions from energy consumption in the Yellow River Basin. J Phys: Conf Ser. 2023;2468(1):012124. doi:10.1088/1742-6596/2468/1/012124. [Google Scholar] [CrossRef]
43. IEA. Emissions Factors 2024. [cited 2025 Jan 7]. Available from: https://www.iea.org/data-and-statistics/data-product/emissions-factors-2024. [Google Scholar]
44. Li J, Li L, Yang R, Jiao J. Assessment of the lifecycle carbon emission and energy consumption of lithium-ion power batteries recycling: a systematic review and meta-analysis. J Energy Storage. 2023;65:107306. doi:10.1016/j.est.2023.107306. [Google Scholar] [CrossRef]
45. Ma Y, Li F, Wang L, Wang G, Kong L. Life cycle carbon emission assessments and comparisons of cast iron and resin mineral composite machine tool bed in China. Int J Adv Manuf Technol. 2021;113(3):1143–52. doi:10.1007/s00170-021-06656-9. [Google Scholar] [CrossRef]
46. You C, Khattak SI, Ahmad M. Impact of innovation in solar photovoltaic energy generation, distribution, or transmission-related technologies on carbon dioxide emissions in China. J Knowl Econ. 2024;15(1):3600–34. doi:10.1007/s13132-023-01284-y. [Google Scholar] [CrossRef]
47. Elalfy DA, Gouda E, Kotb MF, Bureš V, Sedhom BE. Comprehensive review of energy storage systems technologies, objectives, challenges, and future trends. Energy Strategy Rev. 2024;54(45):101482. doi:10.1016/j.esr.2024.101482. [Google Scholar] [CrossRef]
48. Wang Z, Yang X, Zhan Z, Huang Z. Carbon emission measurement and evaluation of large public buildings in the materialization stage. Energy Build. 2024;324(1):114840. doi:10.1016/j.enbuild.2024.114840. [Google Scholar] [CrossRef]
49. Wang H, Huang H, Zhang J, Hu Z, Zhou Q. Environmental processes assessment of a building system based on LCA-emergy–carbon footprint methodology. Processes. 2023;11(11):3113. doi:10.3390/pr11113113. [Google Scholar] [CrossRef]
50. Rossi F, Zuffi C, Parisi ML, Fiaschi D, Manfrida G. Comparative scenario-based LCA of renewable energy technologies focused on the end-of-life evaluation. J Clean Prod. 2023;405:136931. doi:10.1016/j.jclepro.2023.136931. [Google Scholar] [CrossRef]
51. Yi Y, Wu J, Zuliani F, Lavagnolo MC, Manzardo A. Integration of life cycle assessment and system dynamics modeling for environmental scenario analysis: a systematic review. Sci Total Environ. 2023;903(4):166545. doi:10.1016/j.scitotenv.2023.166545. [Google Scholar] [PubMed] [CrossRef]
52. Zarzavilla M, Quintero A, Abellán MA, Serrano FL, Austin MC, Tejedor-Flores N. Comparison of environmental impact assessment methods in the assembly and operation of photovoltaic power plants: a systematic review in the Castilla—La Mancha Region. Energies. 2022;15(5):1926. doi:10.3390/en15051926. [Google Scholar] [CrossRef]
53. Maniscalco M, Longo S, Miccichè G, Cellura M, Ferraro M. A critical review of the environmental performance of bifacial photovoltaic panels. Energies. 2024;17(1):226. doi:10.3390/en17010226. [Google Scholar] [CrossRef]
54. Jasper FB, Späthe J, Baumann M, Peters JF, Ruhland J, Weil M. Life cycle assessment (LCA) of a battery home storage system based on primary data. J Clean Prod. 2022;366(7):132899. doi:10.1016/j.jclepro.2022.132899. [Google Scholar] [CrossRef]
55. Le ST, Nguyen TN, Bui DK, Teodosio B, Ngo TD. Comparative life cycle assessment of renewable energy storage systems for net-zero buildings with varying self-sufficient ratios. Energy. 2024;290(2):130041. doi:10.1016/j.energy.2023.130041. [Google Scholar] [CrossRef]
56. Wang PP, Li YP, Huang GH, Wang SG. A multivariate statistical input-output model for analyzing water-carbon nexus system from multiple perspectives—Jing-Jin-Ji region. Appl Energy. 2022;310:118560. doi:10.1016/j.apenergy.2022.118560. [Google Scholar] [CrossRef]
57. Han J, Tan Z, Chen M, Zhao L, Yang L, Chen S. Carbon footprint research based on input-output model-a global scientometric visualization analysis. Int J Environ Res Public Health. 2022;19(18):11343. doi:10.3390/ijerph191811343. [Google Scholar] [PubMed] [CrossRef]
58. Li J, Tian Y, Zhang Y, Xie K. Assessing spatially multistage carbon transfer in the life cycle of energy with a novel multi-flow and multi-node model: a case of China’s coal-to-electricity chain. J Clean Prod. 2022;339:130699. doi:10.1016/j.jclepro.2022.130699. [Google Scholar] [CrossRef]
59. Zhu K, Liu Q, Xiong X, Zhang Y, Wang M, Liu H. Carbon footprint and embodied carbon emission transfer network obtained using the multi-regional input-output model and social network analysis method: a case of the Hanjiang River basin. China Front Ecol Evol. 2022;10:941520. doi:10.3389/fevo.2022.941520. [Google Scholar] [CrossRef]
60. Liu J, Zhao T. Optimal operation of electricity-gas-heating-cooling integrated energy systems with SCPR-based carbon trading using a novel SMABC algorithm. Renew Energy. 2024;232(2):121138. doi:10.1016/j.renene.2024.121138. [Google Scholar] [CrossRef]
61. Wang L, Ren X, Ma Y, Liu Z, Dong W, Ni L. Optimal low-carbon scheduling of integrated energy systems considering stepped carbon trading and source-load side resources. Energy Rep. 2024;12(3):3145–54. doi:10.1016/j.egyr.2024.09.002. [Google Scholar] [CrossRef]
62. Zhong X, Zhong W, Liu Y, Yang C, Xie S. Optimal energy management for multi-energy multi-microgrid networks considering carbon emission limitations. Energy. 2022;246(4):123428. doi:10.1016/j.energy.2022.123428. [Google Scholar] [CrossRef]
63. Zhang J, Liu Z. Low carbon economic scheduling model for a park integrated energy system considering integrated demand response, ladder-type carbon trading and fine utilization of hydrogen. Energy. 2024;290(28):130311. doi:10.1016/j.energy.2024.130311. [Google Scholar] [CrossRef]
64. Duan J, Xia Y, Cheng R, Gao Q, Liu F. Low carbon and economic optimal operation of electricity-gas integrated energy system considering demand response. Sustain Energy Grids Netw. 2024;38(17):101290. doi:10.1016/j.segan.2024.101290. [Google Scholar] [CrossRef]
65. Guo J, Dou C, Zhang Z, Yue D. Bi-level economic dispatch strategy for virtual power plants based on electric vehicles aggregation. Electr Power Syst Res. 2023;225(6):109783. doi:10.1016/j.epsr.2023.109783. [Google Scholar] [CrossRef]
66. Chen Y, Sun Q, Li Y, Du Z, Guo B, Zhang Y. Bi-level coordinated optimal dispatching method of power system considering renewable energy uncertainty and flexible load aggregators. J Phys: Conf Ser. 2021;1920(1):012044. doi:10.1088/1742-6596/1920/1/012044. [Google Scholar] [CrossRef]
67. Wu J, Liu Y, Chen X, Wang C, Li W. Data-driven adjustable robust Day-ahead economic dispatch strategy considering uncertainties of wind power generation and electric vehicles. Int J Electr Power Energy Syst. 2022;138(2):107898. doi:10.1016/j.ijepes.2021.107898. [Google Scholar] [CrossRef]
68. Keshta HE, Hassaballah EG, Ali AA, Abdel-Latif KM. Multi-level optimal energy management strategy for a grid tied microgrid considering uncertainty in weather conditions and load. Sci Rep. 2024;14(1):10059. doi:10.1038/s41598-024-59655-7. [Google Scholar] [PubMed] [CrossRef]
69. Liu C, Wang C, Yin Y, Yang P, Jiang H. Bi-level dispatch and control strategy based on model predictive control for community integrated energy system considering dynamic response performance. Appl Energy. 2022;310(5):118641. doi:10.1016/j.apenergy.2022.118641. [Google Scholar] [CrossRef]
70. Kasmi G, Touron A, Blanc P, Saint-Drenan YM, Fortin M, Dubus L. Remote-sensing-based estimation of rooftop photovoltaic power production using physical conversion models and weather data. Energies. 2024;17(17):4353. doi:10.3390/en17174353. [Google Scholar] [CrossRef]
71. Scott C, Ahsan M, Albarbar A. Machine learning for forecasting a photovoltaic (PV) generation system. Energy. 2023;278(3):127807. doi:10.1016/j.energy.2023.127807. [Google Scholar] [CrossRef]
72. Zhang L, Lu S, Ding Y, Duan D, Wang Y, Wang P, et al. Probability prediction of short-term user-level load based on random forest and kernel density estimation. Energy Rep. 2022;8:1130–8. doi:10.1016/j.egyr.2022.02.256. [Google Scholar] [CrossRef]
73. Gao W, Zhang Y, Zhang L, Ai C, Chen L. Research on a power smoothing control strategy for energy storage hydraulic wind turbines. Energy Sci Eng. 2023;11(3):989–1004. doi:10.1002/ese3.1375. [Google Scholar] [CrossRef]
74. Kim WS, Eom H, Kwon Y. Optimal design of photovoltaic connected energy storage system using Markov chain models. Sustainability. 2021;13(7):3837. doi:10.3390/su13073837. [Google Scholar] [CrossRef]
75. Zhang J, Sang L, Xu Y, Sun H. Networked multiagent-based safe reinforcement learning for low-carbon demand management in distribution networks. IEEE Trans Sustain Energy. 2024;15(3):1528–45. doi:10.1109/TSTE.2024.3355123. [Google Scholar] [CrossRef]
76. Guo M, Wang W, Chen R, Li Y. Research on bi-level model power dispatch considering the uncertainty of source and load. Sustain Energy Technol Assess. 2022;53(2):102689. doi:10.1016/j.seta.2022.102689. [Google Scholar] [CrossRef]
77. Wang L, Yu J, Ji W. Flexible load participation in peaking shaving and valley filling based on dynamic price incentives. Energy Eng. 2024;121(2):523–40. doi:10.32604/ee.2023.041881. [Google Scholar] [CrossRef]
78. El Feghali J, Sandou G, Guéguen H, Haessig P, Faille D, Bouia H, et al. Electrical grid flexibility via heat pump and thermal storage control. IFAC-PapersOnLine. 2022;55(11):84–9. doi:10.1016/j.ifacol.2022.08.053. [Google Scholar] [CrossRef]
79. Charanand S, Uganya G. Load control using temperature and humidity sensor and comparing temperature with microcontroller device. In: 2022 International Conference on Innovative Computing, Intelligent Communication and Smart Electrical Systems (ICSES); 2022 Jul 15–16; Chennai, India. [Google Scholar]
80. Bhundar HS, Golab L, Keshav S. Using EV charging control to provide building load flexibility. Energy Inform. 2023;6(1):5. doi:10.1186/s42162-023-00261-8. [Google Scholar] [PubMed] [CrossRef]
81. Qing Q, Meng Y, Wu J, Xiao S, Zhang M, Zheng S, et al. Research and application of key technologies for real-time dispatching and control of multi-level power grid with adjustable load. In: 4th Asia Energy and Electrical Engineering Symposium (AEEES); 2022 Mar 25–28; Chengdu, China. [Google Scholar]
82. Zhang Z, Du X, Shang Y, Zhang J, Zhao W, Su J. Research on demand response potential of adjustable loads in demand response scenarios. Energy Eng. 2024;121(6):1577–605. doi:10.32604/ee.2024.047706. [Google Scholar] [CrossRef]
83. Zuo J, Xu C, Wang W, Ji Y. Distributionally robust optimization for virtual power plant clusters considering carbon emission-based dynamic dispatch priority. Front Energy Res. 2023;11:1214263. doi:10.3389/fenrg.2023.1214263. [Google Scholar] [CrossRef]
84. Martínez DM, Ebenhack WB, Wagner TP. Chapter 2—dealing with energy units, measures, and statistics. In: Energy efficiency. Amsterdam, The Netherlands: Elsevier Science; 2019. p. 35–66. [Google Scholar]
85. Shang Y, Xia M, Yi H, Wang Y, Liu D, Xu B. Estimation of the maximum penetration rate of renewable energy considering constraints of grid inertia and primary frequency regulation. In: 2023 4th International Conference on Advanced Electrical and Energy Systems (AEES); 2023 Dec 1–3; Shanghai, China. [Google Scholar]
86. Qiu H, Gu W, Xu Y, Yu W, Pan G, Liu P. Tri-level mixed-integer optimization for two-stage microgrid dispatch with multi-uncertainties. IEEE Trans Power Syst. 2020;35(5):3636–47. doi:10.1109/TPWRS.2020.2987481. [Google Scholar] [CrossRef]
87. Kaewnukultorn T, Hegedus S. Impact of impedances and solar inverter grid controls in electric distribution line with grid voltage and frequency instability. Energies. 2024;17(21):5503. doi:10.3390/en17215503. [Google Scholar] [CrossRef]
88. Pagnini L, Bracco S, Delfino F, de-Simón-Martín M. Levelized cost of electricity in renewable energy communities: uncertainty propagation analysis. Appl Energy. 2024;366(3):123278. doi:10.1016/j.apenergy.2024.123278. [Google Scholar] [CrossRef]
89. Veronese E, Manzolini G, Moser D. Improving the traditional levelized cost of electricity approach by including the integration costs in the techno-economic evaluation of future photovoltaic plants. Int J Energy Res. 2021;45(6):9252–69. doi:10.1002/er.6456. [Google Scholar] [CrossRef]
90. Xu Y, Pei J, Yuan J, Zhao G. Concentrated solar power: technology, economy analysis, and policy implications in China. Environ Sci Pollut Res Int. 2022;29(1):1324–37. doi:10.1007/s11356-021-15779-1. [Google Scholar] [PubMed] [CrossRef]
91. Ma M, He B, Wang N, Shen R. A method for monitoring the solar resources of high-scale photovoltaic power plants based on wireless sensor networks. Sustain Energy Technol Assess. 2022;53(4):102678. doi:10.1016/j.seta.2022.102678. [Google Scholar] [CrossRef]
92. Yu Y, Vincent T, Sansom J, Greenwood D, Marco J. Distributed internal thermal monitoring of lithium ion batteries with fibre sensors. J Energy Storage. 2022;50:104291. doi:10.1016/j.est.2022.104291. [Google Scholar] [CrossRef]
93. Kang Z, Zhang Y, Du Y. Application of multi-sensor information fusion technology in fault early warning of smart grid equipment. Energy Inform. 2024;7(1):123. doi:10.1186/s42162-024-00433-0. [Google Scholar] [CrossRef]
94. Nematirad R, Ardehali MM, Khorsandi A, Mahmoudian A. Optimization of residential demand response program cost with consideration for occupants thermal comfort and privacy. IEEE Access. 2024;12:15194–207. doi:10.1109/ACCESS.2024.3358404. [Google Scholar] [CrossRef]
95. Wang Y, Xiang Y. Photovoltaic-storage energy system management considering wireless data communication. Energy Rep. 2022;8(21):267–73. doi:10.1016/j.egyr.2022.05.152. [Google Scholar] [CrossRef]
96. Zhu Y, Xu X, Yan Z, Lu J. Data acquisition, power forecasting and coordinated dispatch of power systems with distributed PV power generation. Electr J. 2022;35(5):107133. doi:10.1016/j.tej.2022.107133. [Google Scholar] [CrossRef]
97. Siva Balan RV, Gouri MS, Senthilnathan T, Gondkar SR, Gondar RR, Loveline Zeema J, et al. Development of smart energy monitoring using NB-IOT and cloud. Meas Sens. 2023;29:100884. doi:10.1016/j.measen.2023.100884. [Google Scholar] [CrossRef]
98. Fang D, Guan X, Lin L, Peng Y, Sun D, Hassan MM. Edge intelligence based economic dispatch for virtual power plant in 5G Internet of energy. Comput Commun. 2020;151(1):42–50. doi:10.1016/j.comcom.2019.12.021. [Google Scholar] [CrossRef]
99. Poczekajło P, Suszyński R, Antosz A. Review of the usage of fiber optic technologies in electrical power engineering and a development outline in Poland. Energy Rep. 2024;11(23):5227–34. doi:10.1016/j.egyr.2024.04.068. [Google Scholar] [CrossRef]
100. Govindasamy S, Balapattabi SR, Kaliappan B, Badrinarayanan V. Energy management in microgrids using IoT considering uncertainties of renewable energy sources and electric demands: GBDT-JS approach. Electr Eng. 2023;105(6):4409–26. doi:10.1007/s00202-023-01947-8. [Google Scholar] [CrossRef]
101. Verde Romero DA, Villalvazo Laureano E, Jiménez Betancourt RO, Navarro Álvarez E. An open source IoT edge-computing system for monitoring energy consumption in buildings. Results Eng. 2024;21(1):101875. doi:10.1016/j.rineng.2024.101875. [Google Scholar] [CrossRef]
102. Rao CK, Sahoo SK, Yanine FF. A literature review on an IoT-based intelligent smart energy management systems for PV power generation. Hybrid Adv. 2024;5(3):100136. doi:10.1016/j.hybadv.2023.100136. [Google Scholar] [CrossRef]
103. Wang Q, Li N. A long short-term memory neural network algorithm for data-driven spatial load forecasting. Int J Intell Inf Technol. 2024;20(1):1–13. doi:10.4018/IJIIT. [Google Scholar] [CrossRef]
104. Guo W, Xu L, Wang T, Zhao D, Tang X. Photovoltaic power prediction based on hybrid deep learning networks and meteorological data. Sensors. 2024;24(5):1593. doi:10.3390/s24051593. [Google Scholar] [PubMed] [CrossRef]
105. Bhavsar S, Pitchumani R, Ortega-Vazquez MA, Costilla-Enriquez N. A hybrid data-driven and model-based approach for computationally efficient stochastic unit commitment and economic dispatch under wind and solar uncertainty. Int J Electr Power Energy Syst. 2023;151(3):109144. doi:10.1016/j.ijepes.2023.109144. [Google Scholar] [CrossRef]
106. Bui VH, Hussain A, Zarrabian S, Kump PM, Su W. Clustering-based optimal operation of charging stations under high penetration of electric vehicles. Sustain Energy Grids Netw. 2023;36(2):101178. doi:10.1016/j.segan.2023.101178. [Google Scholar] [CrossRef]
107. Zhang Z, Zhang Q, Liang H, Gorbani B. Optimizing electric load forecasting with support vector regression/LSTM optimized by flexible Gorilla troops algorithm and neural networks a case study. Sci Rep. 2024;14(1):22092. doi:10.1038/s41598-024-73893-9. [Google Scholar] [PubMed] [CrossRef]
108. Li G, Zhang R, Bu S, Zhang J, Gao J. Probabilistic prediction-based multi-objective optimization approach for multi-energy virtual power plant. Int J Electr Power Energy Syst. 2024;161(22):110200. doi:10.1016/j.ijepes.2024.110200. [Google Scholar] [CrossRef]
109. Wang T, Hua H, Wei Z, Cao J. Challenges of blockchain in new generation energy systems and future outlooks. Int J Electr Power Energy Syst. 2022;135:107499. doi:10.1016/j.ijepes.2021.107499. [Google Scholar] [CrossRef]
110. Tan S, Liu G. A low carbon management model for regional energy economies based on blockchain technology. Heliyon. 2023;9(9):e19966. doi:10.1016/j.heliyon.2023.e19966. [Google Scholar] [PubMed] [CrossRef]
111. Jiang T, Luo H, Yang K, Sun G, Yu H, Huang Q, et al. Blockchain for energy market: a comprehensive survey. Sustain Energy Grids Netw. 2025;41(1):101614. doi:10.1016/j.segan.2024.101614. [Google Scholar] [CrossRef]
112. Cui D, He J, Zhang G, Hou Z. Blockchain-based distributed power market trading mechanism. Comput Mater Continua. 2022;72(2):2845–58. doi:10.32604/cmc.2022.026568. [Google Scholar] [CrossRef]
113. Boumaiza A. Carbon and energy trading integration within a blockchain-powered peer-to-peer framework. Energies. 2024;17(11):2473. doi:10.3390/en17112473. [Google Scholar] [CrossRef]
114. Khan AA, Laghari AA, Liu DS, Shaikh AA, Ma DD, Wang CY, et al. EPS-ledger: blockchain Hyperledger sawtooth-enabled distributed power systems chain of operation and control node privacy and security. Electronics. 2021;10(19):2395. doi:10.3390/electronics10192395. [Google Scholar] [CrossRef]
115. Chien I, Karthikeyan P, Hsiung PA. Prediction-based peer-to-peer energy transaction market design for smart grid. Eng Appl Artif Intell. 2023;126(6):107190. doi:10.1016/j.engappai.2023.107190. [Google Scholar] [CrossRef]
116. Damisa U, Nwulu NI, Siano P. Towards blockchain-based energy trading: a smart contract implementation of energy double auction and spinning reserve trading. Energies. 2022;15(11):4084. doi:10.3390/en15114084. [Google Scholar] [CrossRef]
117. Suthar S, Cherukuri SHC, Pindoriya NM. Peer-to-peer energy trading in smart grid: frameworks, implementation methodologies, and demonstration projects. Electr Power Syst Res. 2023;214:108907. doi:10.1016/j.epsr.2022.108907. [Google Scholar] [CrossRef]
118. Wang C, Jia X, Liu T, Zhao P, Dong S, Wang J. Research progress on technology and standard of DC household appliances in PEDF building. In: 2023 6th International Conference on Energy, Electrical and Power Engineering (CEEPE); 2023 May 12–14; Guangzhou, China. [Google Scholar]
119. Teferi TG, Tella TG, Hampannavar S. Impact of large-scale renewable energy integration on the grid voltage stability. Results Eng. 2024;23(3):102398. doi:10.1016/j.rineng.2024.102398. [Google Scholar] [CrossRef]
120. Ying X, Yu G, Wang J, Huang J, Zhang Y, Wang X. Review of PEDF air conditioning systems for flexible energy utilization. Int J Res Eng Sci. 2023;11(4):59–62. [Google Scholar]
121. Li T, Shi Z, Han D. Research on the impact of energy technology innovation on total factor ecological efficiency. Environ Sci Pollut Res Int. 2022;29(24):37096–114. doi:10.1007/s11356-021-18204-9. [Google Scholar] [PubMed] [CrossRef]
122. Gan L, Jiang P, Lev B, Zhou X. Balancing of supply and demand of renewable energy power system: a review and bibliometric analysis. Sustain Futur. 2020;2(10):100013. doi:10.1016/j.sftr.2020.100013. [Google Scholar] [CrossRef]
123. Jiang Z, Zheng X, Chen Q. Optimal dispatch of integrated energy system with low-carbon complementation of source side and load side. In: Proceedings of the 7th Purple Mountain Forum on Smart Grid Protection and Control (PMF2022); 2022 Aug 14–15; Nanjing, China. [Google Scholar]
124. Detemple J, Kitapbayev Y, Reppen AM. Renewable energy investment under stochastic interest rate with regime-switching volatility. Energy Econ. 2024;136(4):107734. doi:10.1016/j.eneco.2024.107734. [Google Scholar] [CrossRef]
125. Wang Y, Liu Y, Zhao K, Deng H, Wang F, Zhuo F. PEDF (photovoltaics, energy storage, direct current, flexibility) microgrid cost optimization based on improved whale optimization algorithm. In: 2023 IEEE 14th International Symposium on Power Electronics for Distributed Generation Systems (PEDG); 2023 Jun 9–12; Shanghai, China. [Google Scholar]
126. Tang D, Fu B, Boamah V. Implementation effect of China’s green finance pilot policy based on synthetic control method: a green innovation perspective. Environ Sci Pollut Res Int. 2023;30(18):51711–25. doi:10.1007/s11356-023-25977-8. [Google Scholar] [PubMed] [CrossRef]
127. Cano-Martínez J, Peñalvo-López E, León-Martínez V, Valencia-Salazar I. Dynamic energy prices for residential users based on Deep Learning prediction models of consumption and renewable generation. Renew Energ Power Qual J. 2023;21(1):76–80. doi:10.24084/repqj21.226. [Google Scholar] [CrossRef]
128. Song J, Han B, Guo J, Chen X, Zhao B, Wang S. Reliable collaboration mechanism of side end resources for discrete services of virtual power plants. In: 8th International Conference on Artificial Intelligence and Security (ICAIS); 2022 Jul 22–26; Qinghai, China. [Google Scholar]
129. Zhang Y, He S, Pang M, Li Q. Green technology innovation of energy Internet enterprises: study on influencing factors under dual carbon goals. Energies. 2023;16(3):1405. doi:10.3390/en16031405. [Google Scholar] [CrossRef]
130. Huang J, Han W, Tian H, Qiu J, Chan CC. The application and challenge of energy router in energy Internet. Energy Internet. 2024;1(1):14–26. doi:10.1049/ein2.12006. [Google Scholar] [CrossRef]
131. Li Y, Geng J, Zhu K, Guo X. Energy Internet-oriented power trading platform: review and prospect. In: 7th Asia Conference on Power and Electrical Engineering (ACPEE); 2022 Apr 15–17; Hangzhou, China. [Google Scholar]
132. Cao J, Jin J, Ming Y, Sun C, Zeng X, Jiao Z, et al. Human-cyber-physical systems for energy Internet—a review. Energies. 2023;16(15):5624. doi:10.3390/en16155624. [Google Scholar] [CrossRef]
133. Luo Z, Qu D, Fan L, Yang S. State of charge estimation for the vanadium redox flow battery based on the Sage-Husa adaptive extended Kalman filter. Int J Circuit Theory Appl. 2024;52(1):380–95. doi:10.1002/cta.3734. [Google Scholar] [CrossRef]
134. Shah R, Mittal V, Precilla AM. Challenges and advancements in all-solid-state battery technology for electric vehicles. Journals. 2024;7(3):204–17. doi:10.3390/j7030012. [Google Scholar] [CrossRef]
135. Li G, Zhang Z, Li J, Liu D. Optimizing hybrid energy storage: a multi-objective approach for hydrogen-natural gas systems with carbon-emission management. Int J Hydrogen Energy. 2024;81(1):1003–19. doi:10.1016/j.ijhydene.2024.07.338. [Google Scholar] [CrossRef]
Cite This Article
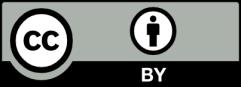
This work is licensed under a Creative Commons Attribution 4.0 International License , which permits unrestricted use, distribution, and reproduction in any medium, provided the original work is properly cited.