Open Access
ARTICLE
Advanced Predictive Analytics for Green Energy Systems: An IPSS System Perspective
1 Electric Engineering Department, Huaian Hongneng Group Co., Ltd., Huaian, 223002, China
2 Faculty of Automation, Huaiyin Institute of Technology, Huaian, 223002, China
* Corresponding Author: Jie Ji. Email:
Energy Engineering 2025, 122(4), 1581-1602. https://doi.org/10.32604/ee.2025.061010
Received 14 November 2024; Accepted 20 January 2025; Issue published 31 March 2025
Abstract
The rapid development and increased installed capacity of new energy sources such as wind and solar power pose new challenges for power grid fault diagnosis. This paper presents an innovative framework, the Intelligent Power Stability and Scheduling (IPSS) System, which is designed to enhance the safety, stability, and economic efficiency of power systems, particularly those integrated with green energy sources. The IPSS System is distinguished by its integration of a CNN-Transformer predictive model, which leverages the strengths of Convolutional Neural Networks (CNN) for local feature extraction and Transformer architecture for global dependency modeling, offering significant potential in power safety diagnostics. The IPSS System optimizes the economic and stability objectives of the power grid through an improved Zebra Algorithm, which aims to minimize operational costs and grid instability. The performance of the predictive model is comprehensively evaluated using key metrics such as Root Mean Square Error (RMSE), Mean Absolute Percentage Error (MAPE), and Coefficient of Determination (R2). Experimental results demonstrate the superiority of the CNN-Transformer model, with the lowest RMSE and MAE values of 0.0063 and 0.00421, respectively, on the training set, and an R2 value approaching 1, at 0.99635, indicating minimal prediction error and strong data interpretability. On the test set, the model maintains its excellence with the lowest RMSE and MAE values of 0.009 and 0.00673, respectively, and an R2 value of 0.97233. The IPSS System outperforms other models in terms of prediction accuracy and explanatory power and validates its effectiveness in economic and stability analysis through comparative studies with other optimization algorithms. The system’s efficacy is further supported by experimental results, highlighting the proposed scheme’s capability to reduce operational costs and enhance system stability, making it a valuable contribution to the field of green energy systems.Graphic Abstract
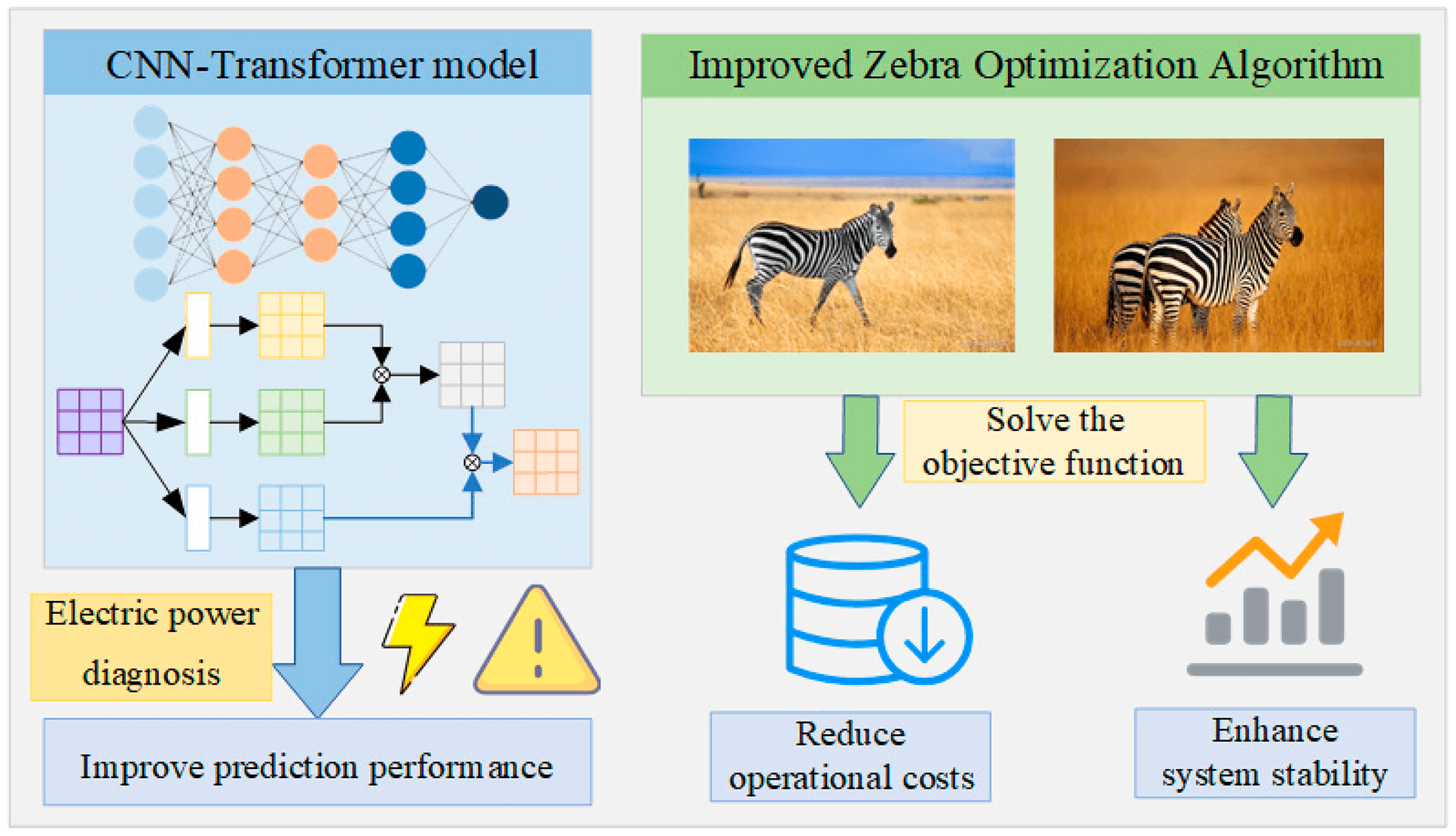
Keywords
Cite This Article
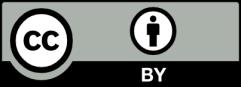
This work is licensed under a Creative Commons Attribution 4.0 International License , which permits unrestricted use, distribution, and reproduction in any medium, provided the original work is properly cited.