Open Access
REVIEW
Overview and Prospect of Distributed Energy P2P Trading
School of Automation and Electrical Engineering, Lanzhou Jiaotong University, Lanzhou, 730070, China
* Corresponding Author: Jiajia Liu. Email:
Energy Engineering 2025, 122(1), 379-404. https://doi.org/10.32604/ee.2024.058137
Received 05 September 2024; Accepted 12 November 2024; Issue published 27 December 2024
Abstract
After a century of relative stability in the electricity sector, the widespread adoption of distributed energy resources, along with recent advancements in computing and communication technologies, has fundamentally altered how energy is consumed, traded, and utilized. This change signifies a crucial shift as the power system evolves from its traditional hierarchical organization to a more decentralized approach. At the heart of this transformation are innovative energy distribution models, like peer-to-peer (P2P) sharing, which enable communities to collaboratively manage their energy resources. The effectiveness of P2P sharing not only improves the economic prospects for prosumers, who generate and consume energy, but also enhances energy resilience and sustainability. This allows communities to better leverage local resources while fostering a sense of collective responsibility and collaboration in energy management. However, there is still no extensive implementation of such sharing models in today’s electricity markets. Research on distributed energy P2P trading is still in the exploratory stage, and it is particularly important to comprehensively understand and analyze the existing distributed energy P2P trading market. This paper contributes with an overview of the P2P markets that starts with the network framework, market structure, technical approach for trading mechanism, and blockchain technology, moving to the outlook in this field.Keywords
Abbreviations
DRE | Distributed Renewable Energy |
P2P | Peer-to-Peer |
MILP | Mixed Integer Linear Programming |
MINLP | Mixed Integer Nonlinear Programming |
ADMM | Alternating Direction Method of Multipliers |
BES | Battery Energy Storage |
PV | Photovoltaic |
RL | Reinforcement Learning |
DQN | Deep Q-Network |
DDPG | Deep Deterministic Policy Gradient |
MAPPO | Multi-intelligent Agent Proximal Policy Optimisation |
DRL | Deep Reinforcement Learning |
EV | Electric Vehicle |
As China’s energy market reforms intensify and the power system moves toward decarbonization, the future distribution network is expected to see a significant increase in decentralized and small-scale distributed energy sources. Encouraging distributed energy sources to participate in market trading further promotes the reform of the power system and the trading model [1]. In this kind of trading market, the traditional consumer of electricity can not only consume electricity but also be a producer of electricity, and there will be no clear boundaries between consumers and producers [2]. While this transition increases the flexibility of the power supply at the consumer end, it also challenges the complexity and regulatory control of the power network [3]. To economically and rationally manage producers and consumers, the distribution-side electricity market must establish a local residual power trading platform that serves both producers and consumers [4], thus building a trend at the level of the electricity sales market from the traditional centralized model of electricity production and consumption to the decentralized autonomy and heterogeneous synergistic development.
Distributed renewable energy (DRE) participation in the electricity trading market is mainly in two ways, centralized and decentralized. In the centralized mode, the user-side resources are integrated and dispatched by the market directly or through market-authorized energy aggregators, and the advantage of this mode is that it can compensate for the uncertainty of distributed renewable energy to a certain extent, and enhance market competitiveness and bidding flexibility. However, it is equivalent to adding a third party between the market and the distributed energy subject, and it needs a reasonable mechanism design for the distribution of benefits between the subjects, while the peer-to-peer (P2P) market transaction mode makes the distributed energy subject can directly participate in the market competition and be responsible for its profit and loss as an independent user, which embodies the concepts of open interconnection, user-centredness, distributedness, and P2P sharing, etc. [5].
The energy flow in the traditional electricity market is unidirectional, where electricity is generated by large-scale power plants, transmitted through high-voltage transmission networks, transformed at substations, and finally distributed to end-users through distribution networks. In P2P energy trading, DRE-equipped users can trade and share energy directly with each other, and they are both generators and consumers of energy [6]. P2P energy trading encourages users to sell the excess energy they generate to other demanders in the interconnected community at prices higher than feed-in tariffs and lower than retail tariffs [7], which enhances the profits of energy generators and reduces energy consumers’ costs [8], and promotes local consumption of distributed generation.
As a result, there has been an increasing amount of research on P2P energy sharing in academia in the last few years. This research can be categorized into three groups: (1) studies that focus on the decision-making process of different participants [9,10]. Literature [9] focuses on various game-theoretic and auction-theoretic approaches by following a systematic classification and gives specific game-theoretic and auction-theoretic models that have recently been used in P2P energy trading. Literature [10] introduced a distributed energy management framework: ELEKTRA, which uses a distributed non-cooperative game theory approach to facilitate producers and consumers in determining the optimal amount of energy to purchase from the utility company while ensuring that their energy needs are met. This addresses how producers and consumers strategically consume energy to meet their needs while maximizing their returns by procuring additional energy from the utility. (2) studies that address the impact of P2P sharing on physical energy networks [11,12]. Literature [12] proposes a methodology based on sensitivity analysis to assess the impact of P2P transactions on the network and to guarantee an exchange of energy that does not violate network constraints. (3) studies blockchain technology as a security technology for P2P platforms [13,14]. Literature [13] is the first systematic review of blockchains in the energy sector.
However, most of these studies focus on very specific topics and lack of a general overview of the topic. The P2P model of energy sharing and trading is still in its infancy in the electricity market. Therefore, comprehensively understanding and analyzing the existing distributed energy P2P trading market is the key to researching a more efficient and practical market mechanism, which is of great significance in terms of consuming distributed energy generation and constructing a new type of power trading market. The main contributions of this paper are as follows:
The main contributions of this paper are as follows:
(1) It redefines the P2P market structure by categorizing it into three dynamic types: centralized, decentralized, and distributed, based on transaction dispersion, highlighting the growing importance of distributed markets in today’s landscape.
(2) A comprehensive analysis of technical methodologies in current P2P market mechanisms reveals distinct scenarios, advantages, and limitations, serving as a strategic guide for selecting the most suitable market approach.
(3) The paper delves into blockchain technology’s role in P2P trading, highlighting its ability to enhance security and traceability via distributed storage and encryption, while also addressing its potential scalability challenges.
(4) It showcases pioneering P2P energy trading projects, pinpointing specific obstacles hindering their widespread adoption and implementation.
(5) Finally, the paper critically assesses existing research gaps and outlines potential future trajectories for P2P trading markets, offering a valuable roadmap for further exploration.
2 P2P Trading Network Framework and Market Structure
2.1 P2P Trading Network Framework
The concept of P2P is also known as the sharing economy, which usually takes place on the distribution network. Electricity can be bought or sold directly between producers and consumers without the intermediary of a traditional energy supplier. The P2P energy trading network framework is shown in Fig. 1 and can be divided into two layers: the virtual layer and the physical layer [15].
Figure 1: P2P energy trading framework diagram
The physical layer consists of a distribution network, which can either be a conventional distributed grid network managed by an independent system operator or an independent microgrid connected to the traditional grid. At the physical layer, the main elements include grid connections, smart metering, and communication facilities.
The virtual layer plays a key role in enabling energy transactions between participants through a secure information system and communication network. In the virtual layer, participants can exchange the necessary information to determine the type, quantity, and price per unit of energy among themselves. They can create buy and sell orders and transmit these orders to other participants through the communication network. Participants can make their own decisions to trade and reach trading agreements with other participants based on their energy needs and generation capacity. The virtual layer includes the information system, market operations, and energy management systems.
In a P2P energy trading network, the physical layer and virtual layer are interconnected through their collaborative roles in facilitating energy transactions. The physical layer includes the distribution network, which can be either a conventional grid or an independent microgrid, as well as grid connections, smart metering, and communication facilities that facilitate the actual flow of energy and data monitoring. This infrastructure provides the foundation for energy delivery and real-time usage tracking. Conversely, the virtual layer enables transactions between participants through a secure information system and communication network. It facilitates the exchange of critical data, such as energy type, quantity, and pricing, allowing users to create buy and sell orders and transmit them to other participants. This layer empowers users to make informed trading decisions based on their energy needs and generation capabilities.
The relationship between the two layers is one of mutual dependence: the physical layer supports the actual energy transfer while the virtual layer governs the transaction processes. Effective communication between these layers ensures that energy supply aligns with demand, enabling efficient market operations and responsive trading agreements. Together, they create a cohesive ecosystem that optimizes energy distribution and facilitates dynamic trading in a decentralized manner.
Considering the way the trading process is executed and the way information is communicated between participants, P2P energy market structures can be classified into three categories [16]: centralized, decentralized, and distributed markets, as shown in Fig. 2.
Figure 2: Three types of market structure for P2P energy trading
In a centralized marketplace, the trading process and exchange of information is carried out in a centralized manner as shown in Fig. 2a. There is a coordinator in the network who coordinates and manages data transfer and communication in the network. Different producers and consumers interested in the transaction will communicate their requirements or wishes to the coordinator. The coordinator directly controls the prices and quantities of energy transactions between producers and consumers and distributes revenues among producers and consumers according to predefined rules. Although individual peers within the market do not directly engage in communication or negotiation regarding energy-sharing parameters, they can indirectly impact these parameters by autonomously setting their energy generation and pricing. Subsequently, this information is shared with the coordinator, influencing the overall decision-making process for energy sharing. One of the greatest advantages of centralized markets is that they maximize social welfare [17,18]. As the penetration of distributed energy sources increases, P2P sharing may incur some computational burden [19]. During P2P sharing, complex data processing and interactions are required, which may have an impact on system performance and stability. In addition, direct control and scheduling in centralized markets may involve monitoring and managing participants’ loads and distributed energy sources, which may raise privacy and security concerns.
In decentralized markets, participants can trade energy directly with each other without relying on centralized institutions or third parties for mediation [20], as shown in Fig. 2b. This decentralized trading model gives participants greater autonomy and flexibility to make decisions based on their needs and preferences [21,22]. In addition, decentralized markets provide better privacy protection. Participants’ transaction and energy consumption information does not need to be disclosed or shared through a coordinator or third party. Instead, this information can be transmitted directly between participants, encrypted, and protected to ensure privacy. However, in a decentralized market, the utility grid company may face difficulties in scheduling resources due to the absence of a centralized coordinator, resulting in less efficient operation of the distribution system [23]. At the same time, individual participants are more concerned with their interests and may neglect the welfare of the whole community or collective.
Distributed markets combine the characteristics of both models of centralized and decentralized markets, as shown in Fig. 2c. In this market type, there is usually a system operator, which issues price signals that indirectly influence the behavior and decisions of market participants. This mechanism allows decision-making and schedule-setting for energy trading to be carried out independently by individual participants, increasing the participation of community members while increasing the privacy of participants [24]. Members of the community can collaborate to maximize the welfare of the community as a whole [25]. In a distributed energy trading market, communities leverage their collective resources and expertise to optimize energy production and consumption, fostering collaboration that enhances overall welfare and efficiency compared to decentralized markets where individual actions are more isolated. This communal approach allows members to share local renewable energy resources, such as solar or wind power, leading to reduced costs and lower energy losses. Profits in these markets are generated through P2P energy trading mechanisms, where surplus energy is sold to neighbors at competitive rates, and through participation in incentive programs that reward renewable energy generation and energy efficiency. Additional sources of profits include cost savings from shared infrastructure investments, demand response programs that allow for flexible energy consumption, and potential revenue from selling carbon credits or innovative energy services. Community efficiency is increased compared to decentralized market types. Trading programs can be checked and adjusted by the system operator through trading prices [26].
Presently, distributed markets dominate the landscape of P2P energy trading, amalgamating the benefits of decentralized and centralized market models. However, they are not without flaws. A notable issue is the unequal distribution of profits among members within the community [27]. This disparity in profit distribution can lead to conflicts among members and impede the progress of trading activities.
A comparison of the advantages and disadvantages of the different market types for P2P trading is shown in Table 1.
3 Technical Approach for P2P Trading Mechanism
According to existing research, the technical approach or P2P trading mechanism is mainly based on four theories to reflect the connection between competition, energy supply and demand, risk, and other factors in the trading process. They are game theory, auction theory, constraint optimization, and reinforcement learning, as shown in Fig. 3.
Figure 3: Classification block diagram of P2P trading mechanism technical approach
Game theory, also known as countermeasure theory, is a mathematical model and analytical method that studies how decision-makers choose strategies to achieve optimal results in an environment of mutual influence and can be divided into two main branches: cooperative game theory and non-cooperative game theory. As an analytical tool for resolving conflicts and disputes, game theory is widely used in the electricity market. It can help to study the conflict of interests between transaction subjects and provide reasonable decision-making and allocation schemes. For example, game theory can be used to solve cost-benefit apportionment problems, such as system network loss apportionment, standby cost apportionment, electricity substitution benefit apportionment, and unit start-up and shutdown cost apportionment.
Non-cooperative game theory emphasizes the rational behavior of individuals and is mainly used to study how participants make decisions to maximize their own interests when their interests are affected by each other. In non-cooperative games, there is usually a lack of direct communication and coordination between the participants, who choose strategies based on their judgment to achieve the most favorable outcome for themselves [28]. In non-cooperative games, Nash equilibrium is a stable choice of strategy, when all participants follow Nash equilibrium strategy, if one participant unilaterally changes his/her strategy and tries to gain a greater benefit, it will destroy the equilibrium state, which may lead to the other participants reacting or taking antagonistic actions.
Elcbay, a microgrid P2P trading platform mentioned in the literature [29], used a non-cooperative game theoretic approach to bidding out supply and demand and found the Nash equilibrium of the game. The study assumes that each market player knows the trading strategies of the other market players. Literature [30,31] proposed distributed algorithms to find the Nash equilibrium point considering the privacy protection of the participants. Literature [32] further applies non-cooperative game theory to microgrid P2P transactions and proves that there is a unique Nash equilibrium point. The above literature modeled the competitive behavior between buyers as a non-cooperative game but did not consider the constraints of the distribution network. Addressing the problem of power network constraints, the literature [33] utilises the generalized Nash equilibrium theory to find the optimal strategies of market members. However, the existing literature usually adopts simpler assumptions when dealing with power network constraints, such as ignoring voltage constraints and phase angle constraints. Such simplifying assumptions may not accurately reflect the real operation of the power system, thus limiting the reliability and applicability of the model. How to build a non-cooperative game model that is more in line with the real power network model remains a challenging research problem.
A particular Non-cooperative game model for P2P transactions is the Stackelberg game. The Stacklelberg game is a master-slave game in which at least one participant is defined as the leader and the other participants are defined as the followers [34]. In the P2P trading mechanism, the interactive behavior between the seller and the buyer can usually be modeled as a multi-leader-multi-follower Stackelberg game. The seller is a multiple leader and the buyer is a multiple follower. Literature [35] modeled the Stackelberg game between buyers and sellers between multiple microgrids and proved the existence of Stackelberg equilibrium. However, the model underestimates the physical constraints of new energy sources. Literature [36] considers the physical constraints of distributed new energy sources and establishes a Stackelberg game model for buyers and sellers. Considering user privacy protection, literature [37,38] propose a distributed computation method of Stackelberg equilibrium for the Stackelberg game model of buyers and sellers.
Cooperative game theory, on the other hand, focuses on collective rational behavior and is used to study how participants arrive at cooperation and how the benefits of cooperation can be fairly and reasonably distributed. Cooperative game theory usually involves cooperation and coordination between multiple players who negotiate, cooperate and make joint decisions to maximize their common interests. Cooperative games can usually be divided into coalition games and coalition formation games. Coalition games focus on the negotiated distribution of benefits generated by participants in the process of cooperation. While the coalition formation game focuses on the establishment of a coalition between participants and the choice of the form of cooperation.
In the literature [39], the supply-side electricity sales and purchases parties form a cooperative alliance and optimize the scheduling of P2P electricity trading to maximize social benefits. Literature [40] constructs an energy alliance game to collaboratively optimize the energy storage units of the producer and consumer to maximize the economic benefits of the producer and consumer. The allocation schemes of classical cooperative game theory mainly include core and Shapley values. The Shapley value is based on the marginal contribution of market members to the overall cooperative process to calculate the distribution of benefits to individual members, while the core is a collection of allocation schemes, none of which is jointly opposed by the game participants. Literature [41,42], respectively, allocates additional benefits from cooperation based on the Shapley value and kernel concepts in cooperative game theory. Moreover, since kernel and Shapley values require considering all possible coalition formation scenarios and calculating the benefits of each coalition, the computational complexity increases dramatically in large-scale cooperative games, making it difficult to use these methods directly.
Literature [43] also adopts the ‘median price’ theory to classify coalition benefits. This theory is simpler to calculate than the complexity of apportionment theories such as Shapley’s value and has been tested in the European market. Literature [44,45] further provide some alternative methods for calculating the allocation scheme of cooperative games to reduce the computational complexity and avoid listing all potential coalitions. Literature [46] studies the cooperative and competitive relationships between microgrids and the impact of different forms of coalition formation on the benefits and strategy choices of each party.
Classical cooperative game theory has some limitations in considering the coupling between market members’ behaviors and physical network constraints. This limitation mainly stems from the fact that classical cooperative game theory usually assumes that the interactions between market members are uncoupled and ignore constraints and externalities on the physical network. In order to address this issue, researchers have proposed an extended cooperative game theory, i.e., cooperative games with externalities. Literature [47] uses the theory of externality cooperative games to maximize the social welfare of distribution network operators, buyers, and sellers and achieve a fair profit distribution under the consideration of network constraints.
Auction theory is an economic methodology utilized for resource allocation, employing competitive bidding to reconcile supply and demand. Within an auction market, numerous potential buyers and sellers concurrently submit multiple purchase and sales orders to the auctioneer. Subsequently, the auctioneer determines the liquidation price of the goods. Consequently, sellers with asking prices meeting or below the clearing price sell their goods, while buyers with bids meeting or exceeding the clearing price purchase their goods at that price point. Energy auctions aim to secure the optimal arrangement for balancing demand and power supply at minimal expense.
Double auction are commonly used as a market clearing mechanism in P2P energy trading frameworks, whereby buyers and sellers bid simultaneously to match orders of interest. This auction mechanism introduces a demand-side response in which all members can participate.
The step-by-step process of the double auction in P2P trading is as follows: (1) Within the gate opening to gate closure time of the P2P market, buyer prosumers submit their bids to an auctioneer, while seller prosumers present their asks. (2) After gate closure, bids are sorted in descending order of the bid price, while the asks are ordered in ascending order of the asking price. (3) After ordering, the aggregated supply and demand curves are created and intersect to determine the auction price as shown in Fig. 4. Each curve in Fig. 4 contains two important pieces of information price and electricity, which correspond to two axes respectively. Buyer with bids above the auction price and sellers with asks below it will participate in the trading process. Conversely, buyers with bids below the auction price and sellers with asks above it will be unable to trade energy in the P2P market.
Figure 4: Clearing mechanism of double auction
Literature [48–50] propose a P2P energy trading mechanism with a two-way auction mechanism based on a blockchain platform, where producers and consumers consider demand response for flexible bidding to facilitate direct energy trading between producers and consumers to maximize revenue. Literature [51] proposes a double auction mechanism for P2P transactions between hybrid electric vehicles, which considers demand response when setting the traded power and price to alleviate the peak load. Literature [52] develops a double auction retail energy market model for electricity and heat coordination, and formulated bidding control strategies for electricity and heat loads to improve economic benefits while ensuring user comfort.
To maximize the benefits of each participant in a P2P trading market, existing studies usually model the individual trading process as a constrained optimization model, and the modeling process usually needs to take into account factors such as energy supply and demand constraints of the participants, network capacity constraints, and market rules. Optimization algorithms can be classified into distributed optimization and centralized optimization. Distributed optimization is widely used in the modeling of market mechanisms because of its decentralized nature which is compatible with the nature of P2P transactions. The distributed optimization algorithms commonly used for P2P trading include mixed integer linear programming (MILP) and alternating direction method of multipliers (ADMM).
MILP represents a subset of integer linear programming where certain variables are required to be integers while others can be non-integers. This form of optimization entails linear objective functions and constraints, with some variables constrained to be integers, typically binary, while others remain continuous. In the context of P2P energy trading, these binary variables commonly arise during the energy management phase. Here, producers aim to efficiently dispatch their local energy generation and storage resources for self-use, determining any surplus or deficit of energy available for trading within the local energy market.
Literature [53] presents an optimization model for a local energy community where each participant, acting as a producer-consumer, possesses solar photovoltaic systems and battery energy storage (BES). These participants engage in electricity trading activities with both the grid and P2P markets, facilitated by retailers and P2P traders. Their proposed formulated algorithm considers the optimal decisions for charging and discharging the BES, the amount of electricity traded with the grid, and the amount of electricity traded in the P2P market, taking into account both the social welfare of the prosumer and the operational constraints of the equipment. In addition, they incorporate the battery depreciation cost and the levelised cost of photovoltaic (PV) generation into the objective function.
Linearisation or convexification of the optimization problem can transform an otherwise complex non-convex problem into a simpler convex MILP problem. Literature [54] proposes an approximate optimization algorithm called ECO-Trade, which aims at coordinating P2P energy trading between smart homes in microgrids. The algorithm utilizes bilinear programming to decompose the non-convex mixed integer nonlinear programming (MINLP) problem into multiple MILP problems and introduces the Pareto optimality condition, which achieves a fair distribution of costs among the participants. Experimental results show that the algorithm is able to significantly reduce computation time while minimizing the impact on accuracy. Literature [55] linearises the problem of trading electric heat energy between commercial and residential producers and consumers into a MILP problem via McCormick relaxation. This linearisation makes the problem easier to solve and finds an approximate optimal solution in an acceptable time Literature [56] takes into account factors such as the uncertainty of PV power generation and the flexibility of loads, and models the problem as a MILP problem by linearising the nonlinear and bilinear terms in the objective function, using the total revenue as the objective function. The PV power allocation is optimized and load balancing is regulated to achieve more efficient P2P energy trading. Literature [57–59] manages the uncertainty of local electricity supply and consumption and uses MILP to optimize local generation and virtual bidding processes to achieve a reduction in the total cost of supply.
ADMM stands out among distributed optimization algorithms as a focal point of research due to its straightforward formulation and assured convergence for convex problems. It effectively addresses convex optimization challenges by breaking them down into more manageable subproblems than the original, facilitating easier handling.
In the context of P2P energy trading, literature [60] proposes a scheme based on ADMM to optimize the allocation of costs for the use of public infrastructure and services. ADMM is used in the literature [61] to locally optimize the reactive power compensation of each inverter participating in a P2P trading for voltage control. ADMM is further used in the literature [62] to solve the network loss problem at the physical layer. The ADMM algorithm is used to solve the community’s social utility function and payment bargaining problem in the P2P energy sharing problem presented in the literature [63]. The convergence of the algorithm is ensured by dividing the decision variables into two blocks and updating them alternately. Literature [64] applied ADMM to derive a generalized Nash equilibrium in a non-cooperative game, resulting in an energy allocation scheme for energy buildings. Additionally, they employed adaptive penalty parameter selection to enhance the convergence speed of the ADMM algorithm.
Reinforcement learning (RL) is a continuous decision-making algorithm that simulates an intelligent body making decisions through continuous learning to maximize rewards in its interaction with the environment. In the process of reinforcement learning, the future state is only related to the current state and behavior, and the previous historical state and decision-making information cannot influence the current state transfer process. Reinforcement learning contains four elements: state, action, strategy, and reward, and the specific principle is shown in Fig. 5.
Figure 5: Principle diagram of RL
Based on whether RL constructs models to simulate external environments, RL can be classified into RL algorithms with and without models. For the former, represented by dynamic programming methods, transfer probabilities, behavioral strategies and rewards need to be known and the optimal strategies are obtained by iteratively solving for function zeros. Model-free algorithms do not need to build a model and achieve the optimal estimation of state transfer probabilities and reward functions through the interaction between the intelligent body and the environment, and their feature of not needing to know the whole market information is consistent with the characteristics of small-scale P2P energy sharing. Therefore, many studies have applied reinforcement learning to energy-sharing strategies to help energy sellers make trading decisions.
Literature [65] establishes a P2P electricity trading model for small-scale prosumers, equates the trading problem to a Markov decision process and analyses the learning elements, proposes a Q-learning algorithm based on the uniform discrete processing of the energy storage actions, and analyses and validates the proposed method by means of the photovoltaic sharing multiuser group arithmetic example. Literature [66] proposes a smart energy community management approach that applies the Q-learning algorithm to the energy trading process. Further considering the demand response, literature [67] applies the Q-learning algorithm to optimally model the response behavior of price-sensitive loads and improves the load forecasting accuracy of electricity sellers using machine learning techniques. Literature [68] applies RL and deep learning to solve the energy trading decision-making problem for microgrids. Deep Q-Network (DQN) is incorporated into a reinforcement learning framework to construct deep reinforcement learning (DRL). In addition, literature [69] takes electric vehicles (EVs) in the context of the day-ahead electricity market as the object of study and employs an iterative algorithm of fitted Q-functions to learn the optimal charging behaviors of EVs and to formulate the day-ahead consumption plan of the fleet. Literature [70] proposes a deep deterministic policy gradient (DDPG) algorithm to optimize energy trading decisions for new energy generation and load uncertainty, which takes into account network constraints and proposes to incentivize energy trading with network tariffs.
Multi-intelligent Body Reinforcement Learning is an extension of reinforcement learning, which applies to strategy solving for multiple objects. In P2P energy trading, each user involved in the transaction constitutes a multi-intelligent body system. Applying the multi-intelligence body reinforcement learning method can effectively avoid modeling the complex microgrid energy trading system, and using historical data for training to obtain the trading strategies of each user, which can be deployed to real-time energy trading. Literature [71] proposed a local energy trading method based on multi-intelligent agent proximal policy optimization (MAPPO) in conjunction with a two-way auction mechanism to address the stochastic nature of bidding in two-way auctions and the uncertainty of distributed renewable energy sources. Also using multi-intelligent agent DDPG, literature [72] combines multi-intelligent agent DDPG with parameter sharing techniques to speed up the sharing of experience and learning of policies among all agents.
3.5 Comparison of Different P2P Trading Mechanisms Technical Approach
The results of the comparative analysis of different technical approaches for P2P energy trading mechanisms are summarised in Table 2.
Market mechanisms based on game theory can model the electricity market as a game model in which the behavior of the players interacts and determines their choices. The mathematical representation of the game theory model can be determined by explicitly defining the players, strategies, and payoff functions. The interests of all participants are taken into account, and the economic equilibrium of the electricity market is analyzed from the perspective of participants’ interactions, which is scalable. However, all participants are assumed to be rational, and this assumption is often not valid in practice. In addition, it is difficult to consider the limitations of distribution network constraints, while multiple equilibria are often encountered when solving the model.
The auction-based market mechanism is the most widely used at present. In the double auction process, buyers and sellers truthfully declare their respective asking and bidding prices according to their real preferences to ensure the effective operation of the market. Thus, the auction mechanism satisfies the nature of individual rationality and incentive compatibility. Individual rationality and incentive compatibility ensure that participants act by their true wishes, reduce information asymmetry, enhance market transparency, and favor the formation of a level playing field. It also ensures that participants can maximize their interests in the auction process, increasing market participation and activity. However, the mechanism requires market participants to engage in the bidding process frequently. Excessive competition can sometimes lead to sustained auction price spikes, and secondly, a large number of participants bidding in the distribution network can lead to non-negligible transaction costs. In addition, the auction mechanism must also introduce a third party to achieve price clearing, which may cause problems such as personal information leakage.
Market mechanisms based on constrained optimization models have a clear physical meaning by considering constraints such as participants’ energy supply and demand constraints, network capacity constraints, and market rules in model building. However, it is usually not able to model the intelligent behavior of the participants, and in the renewable energy P2P power trading market, it is difficult to model the distributed generation for its uncertainty and resolve the optimal offer strategy of the generator. Also, the technique cannot be used in P2P markets with a large number of participants due to computational and communication scalability limitations.
Reinforcement learning-based models model the complex electricity market as a collection of rule-based intelligence, which are simulated to continuously try to make optimal strategies in the environment. In environments with incomplete information, the intelligences achieve complex dynamic equilibria through interactions with the environment and other intelligences. This distributed approach to decision-making allows intelligence to cope with uncertainty and show flexibility in complex systems. Compared to the first three groups of approaches, this set of models is more flexible and easy to implement, opening up a new modeling and analysis method for dealing with the complexity of the power trading business. However, data collection bottlenecks limit further diffusion of the technique, as system operators rarely have access to energy operational data due to market participants’ privacy protection. Furthermore, incomplete data on grid operations may lead to inaccurate results. Additionally, parameter uncertainty within the P2P energy sharing market can impact the outcomes of machine learning algorithms. Consequently, learning-based optimization may not satisfy the robustness demands of P2P energy sharing markets.
The supporting technology for the P2P platform refers to blockchain technology. P2P market can allow distributed agents to interact actively, and thus, new platforms are needed to support distributed principal transactions. Blockchain technology has gained widespread attention for its ability to create a viable trading environment for distributed players. Most of the current tradable energy market platforms are based on blockchain technology.
In P2P energy trading, prosumers with different generation and demand capacities participate in transactions from different locations in the distribution network. Multiple small energy and financial transactions take place between them, and this information needs to be stored in a secure and reliable database. In addition, all participants should be able to access the database in order to cross-verify and trust the trading system. These characteristics will be difficult to achieve if a centralized database system is used to implement P2P energy transactions. To address these challenges, blockchain technology was introduced to overcome the limitations of centralized database systems. Blockchain is a distributed database system [73] that is decentralized through a P2P computer network. As shown in Fig. 6, transaction data is packed in blocks and linked to previous blocks by hashing, forming a tamper-proof blockchain. This linkability and hashing mechanism ensures the invariance of the blockchain data.
Figure 6: Blockchain structure
Blockchain networks can be further classified into public, private, and federated chains based on the permissions of the participants and the degree of decentralization. A public chain is a blockchain network that is open to everyone and anyone is free to join and leave the system. An important feature of a public chain is decentralization. A private chain is a non-public blockchain that is generally created and controlled by a centralized body. Since a private chain is only open to authorized nodes, the read and write access to the data of individual nodes is strictly controlled by the central mechanism. While private chains have a low degree of decentralization and are directly controlled by a central organization, federated chains are in the middle of public and private chains and are the joint responsibility of several organizations. Each organization is therefore able to take responsibility for managing one or more nodes, while access to read and write data is the sole responsibility of the organization. Members of a federated chain must also be invited and achieve consensus before they can participate while having considerable autonomy. In addition, blockchain also supports smart contracts, which are essentially computer protocols that can automatically enforce the terms of a contract. The introduction of smart contracts can effectively improve the operational efficiency and security of the system, and reduce the dependence on third-party organizations.
Blockchain-based P2P transactions have attracted academic attention. Literature [74] designed a federated blockchain for P2P trading between hybrid electric vehicles, which can improve transaction security and privacy protection. Literature [75] proposed a federated blockchain for supporting P2P trading in microgrids. A credit-based payment scheme was designed to overcome the weakness of blockchain technology in terms of transaction confirmation delay. In literature [76], a blockchain based energy trading model is proposed, in which users independently request transactions based on actual energy output and consumption demand to achieve an energy supply balance within the power grid. At the same time, Vickrey Clark Groves auction strategy is designed to constrain user bidding. Finally, a smart contract for multilateral auctions was written and tested on Ethereum, and the analysis results showed the feasibility of the proposed model. Literature [77] proposes a two-tier blockchain framework to take into account power system physical network constraints. Literature [78] uses blockchain for decentralized electricity trading. The information chain is used to complete the authentication and access of the trading parties, and the transaction settlement without third-party trust institutions is completed by signing smart contracts.
While blockchain technology holds great potential for enhancing P2P markets, its scalability remains a significant challenge that needs further exploration. Addressing these issues will be essential for the successful implementation of blockchain systems in large-scale P2P transactions. These issues are mainly in the following areas:
(1) Transaction Throughput: Many blockchain networks face limitations in transaction throughput, which refers to the number of transactions that can be processed per second. For example, Bitcoin can handle approximately 7 transactions per second, while Ethereum processes around 30. In contrast, traditional payment systems like Visa can handle thousands of transactions per second. This disparity raises concerns about whether blockchain can meet the demands of large-scale P2P markets.
(2) Network Congestion: As more users engage in P2P transactions, network congestion can lead to slower transaction times and higher fees. During peak usage periods, users may experience delays in transaction confirmation, which can deter participation and affect user satisfaction.
(3) Data Storage: The size of blockchain data can grow rapidly as more transactions are added. This expansion poses challenges for nodes that must store and maintain a complete copy of the blockchain. As the data grows, it requires more storage capacity and computing power, potentially limiting participation from smaller nodes and reducing the decentralization that blockchain technology aims to achieve.
(4) Consensus Mechanisms: Different consensus mechanisms (e.g., Proof of Work, Proof of Stake) have varying scalability implications. For instance, Proof of Work, while secure, is energy-intensive and slow, making it less suitable for high-frequency P2P transactions. Alternative consensus mechanisms may offer improvements in scalability, but their effectiveness and security trade-offs must be thoroughly examined.
(5) Interoperability: The lack of interoperability between different blockchain platforms can hinder scalability in P2P markets. If users are confined to specific blockchains, it can limit their ability to engage with a broader market, reducing the overall efficiency and attractiveness of blockchain-based P2P systems.
In recent years, P2P energy trading has seen various trials and projects worldwide. This section provides a detailed overview of typical projects.
The Piclo project is the first distributed energy trading pilot launched by the innovative technology company “Open Utility” in collaboration with the renewable energy supplier “Good Energy” in the UK. This project employs a bidirectional auction as the market mechanism, matching orders every 30 min based on market demand and participant preferences to facilitate energy trading. Unlike most demonstration projects, Piclo considers the geographical location of participants during matching, allowing nearby market participants to prioritize order matching, thus reducing energy transmission losses. It also offers data visualization and analytics tools to help customers understand their energy consumption and make adjustments to balance market supply and demand.
The Brooklyn Microgrid project consists of an energy market in Brooklyn, New York, with participants distributed across three interconnected distribution networks. In this project, energy trading occurs between community producers and consumers via smart contracts on an Ethereum-based blockchain platform. Consumption and generation data are transmitted from the Transactive Grid smart meters to participants’ blockchain accounts to create buy and sell orders. Orders are sent to a market mechanism maintained by smart contracts. When buy and sell orders match, payments are executed, and a new block containing all current market information is added to the blockchain. This microgrid helps grid operators reduce the impact of grid supply interruptions through complete decoupling and managing energy supply within the community during natural disasters.
The Sonnen Community is developed by the German storage manufacturer SonnenBatterie. It is a community composed of SonnenBatterie storage and photovoltaic systems, allowing members to share distributed energy without the need for traditional energy suppliers. Community members can fully meet their energy needs on sunny days, often generating a surplus. The excess energy does not enter the utility grid but goes into a virtual energy pool, which can provide energy to other members when they cannot generate enough due to weather conditions. A central software connects and monitors all SonnenCommunity members while balancing energy supply and demand. This approach is similar to Piclo, but SonnenCommunity clearly emphasizes the importance of storage systems.
The RENew Nexus project, funded by the Australian government, is a P2P energy trading pilot project for residential households supported by Powerledger. The project is carried out in three phases. The first phase involved a solar trading trial that lasted seven months from 2018 to 2019, with 18 producers and consumers participating. Results indicated that blockchain P2P trading reduced overall generation costs, providing strong validation of the practical effects of P2P energy trading. The second phase, from October 2019 to January 2020, initially had 30 participants (later reduced to 29) and demonstrated greater economic benefits for producers and consumers through the installation of batteries and participation in virtual power plants. The third phase involves the construction of a microgrid containing a 670-kilowatt-hour shared storage system, expected to provide convenience for 36 households by exchanging excess energy through batteries.
Finland’s P2P-Smart Test is a P2P energy trading platform based on smart grid technology. Advanced information and communication technologies are applied in this project to establish and manage the P2P energy trading platform. These technologies include smart meters, blockchain, data analytics, and real-time communication. By utilizing these technologies, consumers can trade energy directly with generators and receive real-time energy data and trading information. The P2P-SmartTest project also involves the concept of regional markets, establishing an energy trading market within specific areas to promote local energy production and consumption. The P2P methods developed aim to ensure flexibility for demand-side users and achieve effective integration and optimization of distributed energy resources. By developing distributed wireless ICT solutions and integrating necessary network control functions, the project meets the needs for energy trading, network optimization, and real-time communication, facilitating the normal operation of resilient distribution systems.
5.6 Barriers to the Application of the P2P Trading
Although P2P energy trading platforms have a number of projects around the world, there are still some potential barriers to the promotion and application of P2P trading. One of the primary challenges is regulatory hurdles, as many regions have complex legal frameworks that either do not recognize or actively discourage P2P trading. This lack of regulatory support creates uncertainty for participants and can deter investment in these innovative models. Furthermore, the existing grid infrastructure is often centralized and not equipped to handle the decentralized nature of P2P transactions, necessitating costly upgrades and modifications that can be logistically challenging and time-consuming.
In addition to regulatory and infrastructural challenges, there is considerable resistance from traditional utility companies, which may view P2P models as a threat to their established business practices. This opposition can manifest in lobbying against supportive regulations or implementing strategies to maintain their market dominance, ultimately stifling innovation. Technological limitations also pose a challenge, as ensuring the reliability, security, and interoperability of platforms like blockchain is critical for successful P2P trading but remains a complex task. Moreover, many potential participants lack awareness and understanding of how P2P trading works, which can limit engagement and reduce the pool of active users.
Economic factors further complicate the landscape; the viability of P2P trading is heavily influenced by market prices and transaction costs, which can fluctuate and impact participants’ willingness to engage. Social dynamics play a crucial role as well; trust among community members is essential for the success of these models, and any existing social conflicts can undermine collaborative efforts. Finally, scalability issues present significant challenges, as the ability to integrate small, localized systems into larger energy networks can be fraught with logistical difficulties. Addressing these multifaceted barriers requires comprehensive collaboration among regulators, technology developers, utility companies, and community members to create supportive policies and foster an environment conducive to the growth and adoption of P2P energy trading.
6 Outlook of Distributed Energy P2P Research
6.1 Research on Market Mechanisms Considering Network Constraints
In an electricity market, the energy market members are constrained by the actual electricity network, such as line capacity and voltage limitations. If energy trading behaviors do not comply with these network constraints, problems such as voltage fluctuations and violation of line restrictions may occur, which seriously threaten the safe and stable operation of the distribution network. In a centralized tradable energy market, the market operator has relevant information about the distribution network and market members’ offers. Therefore, it is easy to obtain a market clearing scheme that satisfies the network constraints. In distributed P2P energy trading, market participants trade directly with each other, and market members do not have detailed information such as distribution network and market member offers, so the market clearing scheme that satisfies the network constraints cannot be obtained directly. The study of P2P energy trading market mechanism considering distribution network constraints becomes a research difficulty.
6.2 Research on Pricing Mechanisms Considering Network Fee and Loss Cost Sharing
In P2P energy trading, energy trading among market members reduces the energy dependence of distribution network users on the utility. As a result, the utility’s revenues will drop significantly and its financial situation will deteriorate. In this context, the utility’s business model will shift from selling electricity to providing network services. Specifically, as market members rely on the utility’s network for their energy transactions, the utility will charge them network fee to compensate for its financial revenue. The essence of the transit charge is that the utility shares the cost of planning, constructing, and operating the distribution lines with the market members. In addition, since power transmission over the network results in line losses, compensation is required in P2P transactions. A common way to compensate for this is through the inclusion of power transmission losses as a discounted cost in the fees. In order to realize this compensation mechanism and to ensure that the network fee and loss costs are equitably distributed to each participant, research into pricing mechanisms that take into account the sharing of over-the-network and loss costs is therefore still to be investigated.
6.3 Research on P2P Modeling Considering the Uncertainty of New Energy Generation
The rapid development of renewable energy and the diversification of load demand bring greater uncertainty challenges to the organization of electricity markets and the operation of distribution networks. Traditional electricity markets address the deviation between actual generation and consumption and the market traded quantity through standby markets or imbalance deviation penalties. However, as renewable energy scales up, traditional standby or balancing markets require more reserve capacity and higher system flexibility to ensure real-time balancing of power system energy. In addition, renewable energy is subject to higher deviation assessment costs, thus limiting its market competitiveness. Despite the abundance of flexible resources such as demand-side flexible loads and energy storage devices, their distribution is scattered, making it difficult to achieve effective resource allocation. It is necessary to fully integrate and explore load-side flexibility resources through market-based methods to provide spare capacity support for renewable energy to participate in market trading. In the existing P2P energy trading model, renewable energy participates in trading mainly based on their generation forecasts, and although there have been studies modeling the uncertainty of renewable energy output, these models need to be further optimized and improved.
6.4 Research on P2P Trading Model Considering Large-Scale Energy Storage Access
With the continuous access of distributed renewable energy, the problem of insufficient flexibility resources in the distribution network has become increasingly obvious. Despite the gradual maturity of energy storage technologies and the reduction of installation and maintenance costs, the development of customer-side energy storage still faces many challenges, especially considering the infrastructure of existing facilities. Therefore, more cost-effective energy storage application scenarios and models need to be explored to effectively promote the development of demand-side energy storage, thereby steadily improving the flexibility of distribution networks. The traditional distributed energy storage framework usually requires that energy storage equipment be installed individually for each user, but at present, user-side energy storage still faces many problems, such as the long cycle of recovering installation costs, the lack of installation conditions or willingness of some users, and the poor economics of individual energy storage. To this end, it is necessary to make full use of the differences in user energy demand to achieve the complementary sharing of energy storage resources, and to study management strategies and transaction models that can be extended to large-scale energy storage, to further explore the application potential of energy storage.
6.5 Research on Market Mechanisms Taking into Account Irrational Decisions
Unlike traditional electricity markets, the core of the P2P energy market mechanism is user-centered, where users must have the autonomy to control energy production and consumption and be able to set energy prices freely. In addition, the market needs to protect user privacy, lower barriers to participation, reduce transaction costs, and incentivize active participation in the market. Although there have been many studies on market mechanisms for tradable energy, the existing mechanisms have their own strengths and weaknesses and do not fully fulfill these objectives. In addition, existing market mechanisms usually assume that market members are fully rational and make decisions based on expected returns, ignoring irrational factors such as psychological expectations. In practice, distributed energy sources are usually owned by small individual investors, and these small individuals have difficulty making rational decisions in the same way as traditional large-scale power generation conglomerates. Similarly, the stochastic and volatile nature of distributed renewable energy increases risk and uncertainty in energy markets, making it more difficult for market members to make rational decisions. Therefore, tradable energy market mechanisms must take into account the potentially irrational decision-making of members and have the ability to quantify and hedge risk and uncertainty. Prospect theory is able to quantify individual psychological factors and can be used to analyze the potentially irrational decisions of market members.
6.6 Research to Enable Data Access and Privacy Security
The importance of P2P energy sharing is to provide accurate and useful cross-regional energy transactions and data sharing for producer decision-making. However, such data sharing may pose a threat to the privacy of participants. Therefore, there is a need to ensure that the personal information of the participants is secured along with the accessible data.
To ensure the security of transactions, distributed ledger technologies such as blockchain are now widely used in P2P energy trading. Blockchain technology ensures the security and traceability of transactions through distributed data storage and encryption mechanisms. It prevents malicious participants from tampering with transaction records or injecting false information, thus increasing the security of the system. However, providing security through technologies such as blockchain may entail high computational costs. Maintaining a distributed ledger and performing cryptographic calculations may require significant computing resources and energy consumption, thereby increasing the cost of the system. Therefore, there is a need to consider cost-effectiveness and adopt more efficient and cost-effective ways to protect the security of transactions while ensuring the privacy and security of transactional decisions.
6.7 Research on Market Mechanisms for Ancillary Services
In the distributed energy P2P trading market, highly power-electronic user entities can respond in real-time to enhance the flexibility of real-time operation of the power system. The construction of auxiliary service markets such as standby market, frequency support, voltage regulation, etc. can fully mobilize the flexibility of the user body and provide support for the safe, stable, and flexible operation of the main grid.
At present, the tradable auxiliary service field is mainly concentrated in the standby field, and less attention is paid to other business fields. In the auxiliary service market, user-side subjects usually need to respond to system demands in real-time in order to provide the required auxiliary services. However, due to the small capacity of user-side subjects, the response capability of a single user is limited. On the one hand, aggregation can be used to integrate the capacity of multiple user-side subjects, but the aggregation model may introduce communication delays, resulting in the user-side subjects not being able to participate in auxiliary services promptly. On the other hand, decentralized control methods can also be explored to enable user-side subjects to respond to system demands more independently. In addition, the participation of highly power-electronic distributed energy resources in the ancillary services market can lead to a highly heterogeneous service market. Therefore, for other auxiliary service markets, decentralized control methods and efficient communication models for real-time auxiliary services, as well as new control algorithms for collaborative control of heterogeneous subjects, are current areas for further research.
Distributed energy P2P trading can incentivize renewable energy trading among users, and enhance renewable energy consumption capacity by adjusting real-time power prices to balance market supply and demand. This paper addresses the key issues of the distributed energy P2P trading market from the aspects of network structure, market type classification, market mechanism, and key technologies, and obtains the following conclusions:
(1) According to the different degrees of market transaction dispersion, the P2P trading market type structure can be divided into three categories: centralized market, decentralized market, and distributed market. Distributed markets are widely used because they concentrate on the advantages of decentralized and centralized market types.
(2) The underlying theories used in the design of existing P2P trading market mechanisms are explained in detail. Comparing and analyzing the use scenarios and advantages and disadvantages of these methods, it provides a reference for choosing to use the appropriate market mechanism in the appropriate scenario.
(3) Blockchain technology ensures the security and traceability of transactions through distributed data storage and encryption mechanisms. However, its scalability remains a significant challenge.
(4) Provides a detailed description of a typical existing P2P energy trading project and identifies some potential barriers in the process of promoting and applying the P2P trading model.
(5) Discussions are made on the future research direction of the distributed energy P2P trading market, which provides a reference for further research to be done on transactions oriented to distributed energy.
Acknowledgement: None.
Funding Statement: This research was funded by the National Natural Science Foundation of China (52167013), the Key Program of Natural Science Foundation of Gansu Province (24JRRA225) and Natural Science Foundation of Gansu Province (23JRRA891).
Author Contributions: The authors confirm contribution to the paper as follows: study conception and design: Jiajia Liu, Mingxing Tian; data collection: Xusheng Mao; analysis and interpretation of results: Jiajia Liu; draft manuscript preparation: Jiajia Liu. All authors reviewed the results and approved the final version of the manuscript.
Availability of Data and Materials: The authors confirm that the data supporting the findings of this study are available within the article.
Ethics Approval: Not applicable.
Conflicts of Interest: The authors declare no conflicts of interest to report regarding the present study.
References
1. Y. Zahraoui, T. Korõtko, A. Rosin, and H. Agabus, “Market mechanisms and trading in microgrid local electricity markets: A comprehensive review,” Energies, vol. 16, no. 5, pp. 1–52, Feb. 2023. doi: 10.3390/en16052145. [Google Scholar] [CrossRef]
2. I. El-Sayed, K. Khan, X. Dominguez, and P. Arboleya, “A real pilot-platform implementation for blockchain-based peer-to-peer energy trading,” presented at 2020 IEEE Power Energy Soc. Gen. Meet. (PESGMMontreal, QC, Canada, Aug. 2020. doi: 10.1109/PESGM41954.2020.9281855. [Google Scholar] [CrossRef]
3. J. P. Iria, F. J. Soares, and M. A. Matos, “Trading small prosumers flexibility in the energy and tertiary reserve markets,” IEEE Trans. Smart Grid, vol. 10, no. 3, pp. 2371–2382, Jan. 2019. doi: 10.1109/TSG.2018.2797001. [Google Scholar] [CrossRef]
4. M. I. Azim, W. Tushar, T. K. Saha, C. Yuen, and D. Smith, “Peer-to-peer kilowatt and negawatt trading: A review of challenges and recent advances in distribution networks,” Renew. Sustain. Energy Rev., vol. 169, no. 3, Nov. 2022, Art. no. 112908. doi: 10.1016/j.rser.2022.112908. [Google Scholar] [CrossRef]
5. W. Tushar, C. Yuen, T. K. Saha, T. Morstyn, and A. C. Chapman, “Peer-to-peer energy systems for connected communities: A review of recent advances and emerging challenges,” Appl. Energy, vol. 282, no. 3, pp. 2567–2580, Jan. 2021. doi: 10.1016/j.apenergy.2020.116131. [Google Scholar] [CrossRef]
6. J. Kim and Y. Dvorkin, “A P2P-dominant distribution system architecture,” IEEE Trans. Power Syst., vol. 35, no. 4, pp. 2716–2725, Dec. 2019. doi: 10.1109/TPWRS.2019.2961330. [Google Scholar] [CrossRef]
7. T. Morstyn, N. Farrell, S. J. Darby, and M. D. McCulloch, “Using peer-to-peer energy-trading platforms to incentivize prosumers to form federated power plants,” Nat. Energy, vol. 3, no. 2, pp. 94–101, Feb. 2018. doi: 10.1038/s41560-017-0075-y. [Google Scholar] [CrossRef]
8. Y. Zhou, J. Z. Wu, C. Long, M. Cheng, and C. H. Zhang, “Performance evaluation of peer-to-peer energy sharing models,” Energy Proced., vol. 143, no. 3, pp. 817–822, Dec. 2017. doi: 10.1016/j.egypro.2017.12.768. [Google Scholar] [CrossRef]
9. W. Tushar, C. Yuen, H. Mohsenian-Rad, T. Saha, H. V. Poor and K. L. Wood, “Transforming energy networks via peer-to-peer energy trading: The potential of game-theoretic approaches,” IEEE Signal Process. Mag., vol. 35, no. 4, pp. 90–111, Jun. 2018. doi: 10.1109/MSP.2018.2818327. [Google Scholar] [CrossRef]
10. N. Kemp, M. S. Siraj, and E. E. Tsiropoulou, “ELEKTRA: Empowering prosumers with distributed prosumer grouping and game-theoretic energy procurement,” Simul. Model. Pract. Theory, vol. 133, no. 1, May 2024, Art. no. 102918. doi: 10.1016/j.simpat.2024.102918. [Google Scholar] [CrossRef]
11. J. Guerrero, A. C. Chapman, and G. Verbic, “Decentralized P2P energy trading under network constraints in a low-voltage network,” IEEE Trans. Smart Grid, vol. 10, no. 5, pp. 5163–5173, Oct. 2018. doi: 10.1109/TSG.2018.2878445. [Google Scholar] [CrossRef]
12. K. Zhang, S. Troitzsch, S. Hanif, and T. Hamacher, “Coordinated market design for peer-to-peer energy trade and ancillary services in distribution grids,” IEEE Trans. Smart Grid, vol. 11, no. 4, pp. 2929–2941, Jan, 2020. doi: 10.1109/TSG.2020.2966216. [Google Scholar] [CrossRef]
13. M. Andoni, V. Robu, D. Flynn, S. Abram, and D. Geach, “Blockchain technology in the energy sector: A systematic review of challenges and opportunities,” Renew. Sustain. Energy Rev., vol. 100, no. 1, pp. 147–174, 2019. doi: 10.1016/j.rser.2018.10.014. [Google Scholar] [CrossRef]
14. G. van Leeuwen, T. AlSkaif, M. Gibescu, and W. van Sark, “An integrated blockchain based energy management platform with bilateral trading for microgrid communities,” Appl. Energy, vol. 263, no. 2, Apr. 2020, Art. no. 114613. doi: 10.1016/j.apenergy.2020.114613. [Google Scholar] [CrossRef]
15. W. Tushar, T. K. Saha, C. Yuen, D. Smith, and H. V. Poor, “Peer-to-peer trading in electricity networks: An overview,” IEEE Trans. Smart Grid, vol. 11, no. 4, pp. 3815–3820, Jan. 2020. doi: 10.1109/TSG.2020.2969657. [Google Scholar] [CrossRef]
16. Y. Zhou, J. Z. Wu, C. Long, and W. L. Ming, “State-of-the-art analysis and perspectives for peer-to-peer energy trading,” Engineering, vol. 6, no. 7, pp. 739–753, Jul. 2020. doi: 10.1016/j.eng.2020.06.002. [Google Scholar] [CrossRef]
17. A. Lüth, J. M. Zepter, P. C. Del Granado, and R. Egging, “Local electricity market designs for peer-to-peer trading: The role of battery flexibility,” Appl. Energy, vol. 229, no. 1, pp. 1233–1243, Nov. 2018. doi: 10.1016/j.apenergy.2018.08.004. [Google Scholar] [CrossRef]
18. W. G. Hou, L. Guo, and Z. L. Ning, “Local electricity storage for blockchain-based energy trading in industrial Internet of Things,” IEEE Trans. Ind. Informat., vol. 15, no. 6, pp. 3610–3619, Feb. 2019. doi: 10.1109/TII.2019.2900401. [Google Scholar] [CrossRef]
19. D. Papadaskalopoulos and G. Strbac, “Decentralized participation of flexible demand in electricity markets-part i: Market mechanism,” IEEE Trans. Power Syst., vol. 28, no. 4, pp. 3658–3666, Apr. 2013. doi: 10.1109/TPWRS.2013.2245686. [Google Scholar] [CrossRef]
20. W. Tushar, T. K. Saha, C. Yuen, T. Morstyn, and M. D. McCulloch, “A motivational game-theoretic approach for peer-to-peer energy trading in the smart grid,” Appl. Energy, vol. 243, no. 10, pp. 10–20, Jun. 2019. doi: 10.1016/j.apenergy.2019.03.111. [Google Scholar] [CrossRef]
21. T. Morstyn, A. Teytelboym, and M. D. McCulloch, “Bilateral contract networks for peer-to-peer energy trading,” IEEE Trans. Smart Grid, vol. 10, no. 2, pp. 2026–2035, Feb. 2018. doi: 10.1109/TSG.2017.2786668. [Google Scholar] [CrossRef]
22. E. Sorin, L. Bobo, and P. Pinson, “Consensus-based approach to peer-to-peer electricity markets with product differentiation,” IEEE Trans. Power Syst., vol. 34, no. 2, pp. 994–1004, Oct. 2018. doi: 10.1109/TPWRS.2018.2872880. [Google Scholar] [CrossRef]
23. J. Guerrero, A. Chapman, and G. Verbic, “A study of energy trading in a low-voltage network: Centralised and distributed approaches,” presented at 2017 Australasian Univ. Pow. Eng. Conf. (AUPECMelbourne, VIC, Australia, Nov. 2017. Accessed: Nov. 11, 2024. doi: 10.1109/AUPEC.2017.8282502. [Google Scholar] [CrossRef]
24. Y. Zhou, J. Z. Wu, and C. Long, “Evaluation of peer-to-peer energy sharing mechanisms based on a multiagent simulation framework,” Appl. Energy, vol. 222, no. 15, pp. 993–1022, Jul. 2018. doi: 10.1016/j.apenergy.2018.02.089. [Google Scholar] [CrossRef]
25. R. Alvaro-Hermana, J. Fraile-Ardanuy, P. J. Zufiria, L. Knapen, and D. Janssens, “Peer to peer energy trading with electric vehicles,” IEEE Intell. Transp. Syst. Mag., vol. 8, no. 3, pp. 33–44, Jul. 2016. doi: 10.1109/MITS.2016.2573178. [Google Scholar] [CrossRef]
26. C. Long, J. Z. Wu, C. H. Zhang, L. Thomas, M. Cheng and N. Jenkins, “Peer-to-peer energy trading in a community microgrid,” presented at IEEE Pow. Energy Soc. General Meet., Chicago, IL, USA, Jul. 2017. doi: 10.1109/PESGM.2017.8274546. [Google Scholar] [CrossRef]
27. T. Morstyn and M. D. McCulloch, “Multiclass energy management for peer-to-peer energy trading driven by prosumer preferences,” IEEE Trans. Power Syst., vol. 34, no. 5, pp. 4005–4014, May 2018. doi: 10.1109/TPWRS.2018.2834472. [Google Scholar] [CrossRef]
28. J. Y. Li, C. R. Zhang, Z. Xu, J. H. Wang, J. Zhao and Y. J. A. Zhang, “Distributed transactive energy trading framework in distribution networks,” IEEE Trans. Power Syst., vol. 33, no. 6, pp. 7215–7227, Jul. 2018. doi: 10.1109/TPWRS.2018.2854649. [Google Scholar] [CrossRef]
29. C. H. Zhang, J. Z. Wu, Y. Zhou, M. Cheng, and C. Long, “Peer-to-peer energy trading in a microgrid,” Appl. Energy, vol. 220, no. 9, pp. 1–12, Jun. 2018. doi: 10.1016/j.apenergy.2018.03.010. [Google Scholar] [CrossRef]
30. Y. Wang, W. Saad, Z. Han, H. V. Poor, and T. Başar, “A game-theoretic approach to energy trading in the smart grid,” IEEE Trans. Smart Grid, vol. 5, no. 3, pp. 1439–1450, Apr. 2014. doi: 10.1109/TSG.2013.2284664. [Google Scholar] [CrossRef]
31. H. Chen, Y. Li, R. H. Y. Louie, and B. Vucetic, “Autonomous demand side management based on energy consumption scheduling and instantaneous load billing: An aggregative game approach,” IEEE Trans. Smart Grid, vol. 5, no. 4, pp. 1744–1754, Jul. 2014. doi: 10.1109/TSG.2014.2311122. [Google Scholar] [CrossRef]
32. A. M. Jadhav and N. R. Patne, “A novel approach to neighborhood fair energy trading in a distribution network of multiple microgrid clusters,” IEEE Trans. Ind. Electron., vol. 66, no. 2, pp. 1520–1531, Mar. 2018. doi: 10.1109/TIE.2018.2815945. [Google Scholar] [CrossRef]
33. Y. Chen, W. Wei, H. Wang, and Q. Zhou, “An energy sharing mechanism achieving the same flexibility as centralized dispatch,” IEEE Trans. Smart Grid, vol. 12, no. 4, pp. 3379–3389, Feb. 2021. doi: 10.1109/TSG.2021.3060380. [Google Scholar] [CrossRef]
34. S. W. Mei, F. Liu, and W. Wei, Fundamentals of Engineering Game Theory and Applications in Power Systems. Beijing, China: Science Press, 2016. [Google Scholar]
35. J. Lee, J. Guo, J. K. Choi, and M. Zukerman, “Distributed energy trading in microgrids: A game-theoretic model and its equilibrium analysis,” IEEE Trans. Ind. Electron., vol. 62, no. 6, pp. 3524–3533, Jan. 2015. doi: 10.1109/TIE.2014.2387340. [Google Scholar] [CrossRef]
36. A. M. Jadhav and N. R. Patne, “Priority-based energy scheduling in a smart distributed network with multiple microgrids,” IEEE Trans. Ind. Informat., vol. 13, no. 6, pp. 3134–3143, Feb. 2017. doi: 10.1109/TII.2017.2671923. [Google Scholar] [CrossRef]
37. N. Liu, X. H. Yu, C. Wang, C. J. Li, L. Ma and J. Y. Lei, “Energy-sharing model with price-based demand response for microgrids of peer-to-peer prosumers,” IEEE Trans. Ind. Electron, vol. 32, no. 5, pp. 3569–3583, Jan. 2017. doi: 10.1109/TPWRS.2017.2649558. [Google Scholar] [CrossRef]
38. K. Anoh, S. Maharjan, A. Ikpehai, Y. Zhang, and B. Adebisi, “Energy peer-to-peer trading in virtual microgrids in smart grids: A game-theoretic approach,” IEEE Trans. Smart Grid, vol. 11, no. 2, pp. 1264–1275, Aug. 2019. doi: 10.1109/TSG.2019.2934830. [Google Scholar] [CrossRef]
39. Z. Li, L. Chen, and G. Nan, “Small-scale renewable energy source trading: A contract theory approach,” IEEE Trans. Ind. Informat., vol. 14, no. 4, pp. 1491–1500, Nov. 2017. doi: 10.1109/TII.2017.2776241. [Google Scholar] [CrossRef]
40. J. Li, Y. J. Ye, and G. Strbac, “Stabilizing peer-to-peer energy trading in prosumer coalition through computational efficient pricing,” Electr. Power Syst. Res., vol. 189, no. 6, Dec. 2020, Art. no. 106764. doi: 10.1016/j.epsr.2020.106764. [Google Scholar] [CrossRef]
41. C. S. Feng, F. S. Wen, S. You, Z. Li, F. Shahnia and M. Shahidehpour, “Coalitional game based transactive energy management in local energy communities,” IEEE Trans. Power Syst., vol. 35, no. 3, pp. 1729–1740, Dec. 2020. doi: 10.1109/TPWRS.2019.2957537. [Google Scholar] [CrossRef]
42. L. Y. Han, T. Morstyn, and M. McCulloch, “Incentivizing prosumer coalitions with energy management using cooperative game theory,” IEEE Trans. Power Syst., vol. 34, no. 1, pp. 303–313, Jul. 2018. doi: 10.1109/TPWRS.2018.2858540. [Google Scholar] [CrossRef]
43. W. Tushar, T. K. Saha, C. Yuen, P. Liddell, R. Bean and H. V. Poor, “Peer-to-peer energy trading with sustainable user participation: A game theoretic approach,” IEEE Access, vol. 6, pp. 62932–62943, Oct. 2018. doi: 10.1109/ACCESS.2018.2875405. [Google Scholar] [CrossRef]
44. J. Li, Y. Ye, D. Papadaskalopoulos, and G. Strbac, “Computationally efficient pricing and benefit distribution mechanisms for incentivizing stable peer-to-peer energy trading,” IEEE Internet Things J., vol. 8, no. 2, pp. 734–749, Jul. 2020. doi: 10.1109/JIOT.2020.3007196. [Google Scholar] [CrossRef]
45. W. Gan, M. Yan, W. Yao, and J. Wen, “Peer to peer transactive energy for multiple energy hub with the penetration of high-level renewable energy,” Appl. Energy, vol. 295, no. 2, pp. 734–749, Aug. 2021. doi: 10.1016/j.apenergy.2021.117027. [Google Scholar] [CrossRef]
46. W. Tushar et al., “A coalition formation game framework for peer-to-peer energy trading,” Appl. Energy, vol. 261, no. 10, Mar. 2020, Art. no. 114436. doi: 10.1016/j.apenergy.2019.114436. [Google Scholar] [CrossRef]
47. W. F. Zhong, S. L. Xie, K. Xie, Q. Y. Yang, and L. H. Xie, “Cooperative P2P energy trading in active distribution networks: An MILP-based nash bargaining solution,” IEEE Trans. Smart Grid, vol. 12, no. 2, pp. 1264–1276, Oct. 2020. doi: 10.1109/TSG.2020.3031013. [Google Scholar] [CrossRef]
48. J. Wang, N. C. Zhou, Q. G. Wang, and P. Wang, “Electricity direct transaction mode and strategy in microgrid based on blockchain and continuous double auction mechanism,” Proc. CSEE, vol. 38, no. 17, pp. 5072–5084, Sep. 2018. doi: 10.13334/J.0258-8013.PCSEE.171955. [Google Scholar] [CrossRef]
49. D. Han, C. Z. H. Zhang, J. Ping, and Z. Yan, “Smart contract architecture for decentralized energy trading and management based on blockchains,” Energy, vol. 199, no. 3, May 2020, Art. no. 117417. doi: 10.1016/j.energy.2020.117417. [Google Scholar] [CrossRef]
50. Z. B. Wang, X. D. Yu, and Y. F. Mu, “A distributed peer-to-peer energy transaction method for diversified prosumers in urban community microgrid system,” Appl. Energy, vol. 260, no. 4, Feb. 2020, Art. no. 114327. doi: 10.1016/j.apenergy.2019.114327. [Google Scholar] [CrossRef]
51. J. W. Kang, R. Yu, X. M. Huang, S. Maharjan, Y. Zhang and E. Hossain, “Enabling localized peer-to-peer electricity trading among plug-in hybrid electric vehicles using consortium blockchains,” IEEE Trans. Ind. Informat., vol. 13, no. 6, pp. 3154–3164, May 2017. doi: 10.1109/TII.2017.2709784. [Google Scholar] [CrossRef]
52. D. Wang et al., “Integrated demand response in district electricity heating network considering double auction retail energy market based on demand-side energy stations,” Appl. Energy, vol. 248, no. 1, pp. 656–678, Aug. 2019. doi: 10.1016/j.apenergy.2019.04.050. [Google Scholar] [CrossRef]
53. H. J. Gao, F. Zhang, and J. Y. Liu, “Design of P2P transaction mechanism considering differentiation characteristics of multiple prosumers in community microgrid system,” Proc. CSEE, vol. 42, no. 4, pp. 1455–1470, 2022. [Google Scholar]
54. K. X. Chen, J. Lin, and Y. H. Song, “Trading strategy optimization for a prosumer in continuous double auction-based peer-to-peer market: A prediction-integration model,” Appl. Energy, vol. 42, no. 4, pp. 1121–1133, May 2019. doi: 10.1016/j.apenergy.2019.03.094. [Google Scholar] [CrossRef]
55. S. Nguyen, W. Peng, P. Sokolowski, D. Alahakoon, and X. Yu, “Optimizing rooftop photovoltaic distributed generation with battery storage for peer-to-peer energy trading,” Appl. Energy, vol. 228, no. 5, pp. 2567–2580, Oct. 2018. doi: 10.1016/j.apenergy.2018.07.042. [Google Scholar] [CrossRef]
56. M. R. Alam, M. St-Hilaire, and T. Kunz, “Peer-to-peer energy trading among smart homes,” Appl. Energy, vol. 238, no. 3, pp. 1434–1443, Mar. 2019. doi: 10.1016/j.apenergy.2019.01.091. [Google Scholar] [CrossRef]
57. R. Jing, M. N. Xie, and F. X. Wang, “Fair P2P energy trading between residential and commercial multi-energy systems enabling integrated demand-side management,” Appl. Energy, vol. 262, no. 6, Mar. 2020, Art. no. 114551. doi: 10.1016/j.apenergy.2020.114551. [Google Scholar] [CrossRef]
58. Z. Zhang, R. Li, and F. R. Li, “A novel peer-to-peer local electricity market for joint trading of energy and uncertainty,” IEEE Trans. Smart Grid, vol. 11, no. 2, pp. 1205–1215, Aug. 2019. doi: 10.1109/TSG.2019.2933574. [Google Scholar] [CrossRef]
59. H. Rashidizadeh-Kermani, M. Vahedipour-Dahraie, M. Shafie-khah, and P. Siano, “Optimal bidding of profit-seeking virtual associations of smart prosumers considering peer to peer energy sharing strategy,” Int. J. Electr. Power Energy Syst., vol. 132, no. 5, Nov. 2021, Art. no. 107175. doi: 10.1016/j.ijepes.2021.107175. [Google Scholar] [CrossRef]
60. T. Morstyn, A. Teytelboym, C. Hepburn, and M. D. McCulloch, “Integrating P2P energy trading with probabilistic distribution locational marginal pricing,” IEEE Trans. Smart Grid, vol. 11, no. 4, pp. 3095–3106, Dec. 2019. doi: 10.1109/TSG.2019.2963238. [Google Scholar] [CrossRef]
61. K. H. Chung and D. Hur, “Hur,Towards the design of P2P energy trading scheme based on optimal energy scheduling for prosumers,” Energies, vol. 13, no. 19, Oct. 2020, Art. no. 5177. doi: 10.3390/en13195177. [Google Scholar] [CrossRef]
62. T. Baroche, P. Pinson, R. L. G. Latimier, and H. B. Ahmed, “Exogenous cost allocation in peer-to-peer electricity markets,” IEEE Trans. Power Syst., vol. 34, no. 4, pp. 2553–2564, Jan. 2019. doi: 10.1109/TPWRS.2019.2896654. [Google Scholar] [CrossRef]
63. H. Almasalma, S. Claeys, and G. Deconinck, “Peer-to-peer-based integrated grid voltage support function for smart photovoltaic inverters,” Appl. Energy, vol. 239, no. 2, pp. 1037–1048, 2019. doi: 10.1016/j.apenergy.2019.01.249. [Google Scholar] [CrossRef]
64. Y. Xu, H. Sun, and W. Gu, “Gu,A novel discounted min-consensus algorithm for optimal electrical power trading in grid-connected DC microgrids,” IEEE Trans. Ind. Electron., vol. 66, no. 11, pp. 8474–8484, Jan. 2019. doi: 10.1109/TIE.2019.2891445. [Google Scholar] [CrossRef]
65. D. L. Rodrigues, X. Ye, X. Xia, and B. Zhu, “Battery energy storage sizing optimization for different ownership structures in a peer-to-peer energy sharing community,” Appl. Energy, vol. 262, no. 3, Mar. 2020, Art. no. 114498. doi: 10.1016/j.apenergy.2020.114498. [Google Scholar] [CrossRef]
66. C. Long, J. Z. Wu, Y. Zhou, and N. Jenkins, “Peer-to-peer energy sharing through a two-stage aggregated battery control in a community Microgrid,” Appl. Energy, vol. 226, no. 4, pp. 261–276, Jan. 2019. doi: 10.1016/j.apenergy.2018.05.097. [Google Scholar] [CrossRef]
67. D. Wang, B. Liu, and H. J. Jia, “Peer-to-peer energy transaction decision of prosumers based on reinforcement learning,” Autom. Electr. Power Syst., vol. 45, no. 3, pp. 139–147, Feb. 2021. doi: 10.7500/AEPS20200515005. [Google Scholar] [CrossRef]
68. T. Chen and W. C. Su, “Local energy trading behavior modeling with deep reinforcement learning,” IEEE Access, vol. 6, pp. 2169–3536, Oct. 2018. doi: 10.1109/ACCESS.2018.2876652. [Google Scholar] [CrossRef]
69. H. Nehrir, K. Dehghanpour, J. W. Sheppard, and N. Kelly, “Agent-based modeling of retail electrical energy markets with demand response,” IEEE Trans. Smart Grid, vol. 9, no. 4, pp. 3465–3475, Nov. 2016. doi: 10.1109/TSG.2016.2631453. [Google Scholar] [CrossRef]
70. T. Y. Chen and S. R. Bu, “Realistic peer-to-peer energy trading model for microgrids using deep reinforcement learning,” presented at 2019 IEEE PES Innov. Smart Grid Technol. Europe (ISGT-EuropeBucharest, Romania, Sep. 2019. doi: 10.1109/ISGTEurope.2019.8905731. [Google Scholar] [CrossRef]
71. S. Vandael, B. Claessens, D. Ernst, T. Holvoet, and G. Deconinck, “Reinforcement learning of heuristic EV fleet charging in a day-ahead electricity market,” IEEE Trans. Smart Grid, vol. 6, no. 4, pp. 1795–1805, Mar. 2015. doi: 10.1109/TSG.2015.2393059. [Google Scholar] [CrossRef]
72. C. Samende, J. Cao, and Z. Fan, “Multi-agent deep deterministic policy gradient algorithm for peer-to-peer energy trading considering distribution network constraints,” Appl. Energy, vol. 317, no. 7, Jul. 2022, Art. no. 119123. doi: 10.1016/j.apenergy.2022.119123. [Google Scholar] [CrossRef]
73. C. I. Dick and A. PraktiknjoDick, “Blockchain technology and electricity wholesale markets: Expert Insights on potentials and challenges for OTC trading in Europe,” Energies, vol. 12, no. 5, Mar. 2019, Art. no. 832. doi: 10.3390/en12050832. [Google Scholar] [CrossRef]
74. Y. Li and B. Hu, “A consortium blockchain-enabled secure and privacy-preserving optimized charging and discharging trading scheme for electric vehicles,” IEEE Trans. Ind. Informat., vol. 17, no. 3, pp. 1968–1977, Apr. 2020. doi: 10.1109/TII.2020.2990732. [Google Scholar] [CrossRef]
75. Z. Li, J. Kang, R. Yu, D. Ye, Q. Deng and Y. Zhang, “Consortium blockchain for secure energy trading in industrial Internet of things,” IEEE Trans. Ind. Informat., vol. 14, no. 8, pp. 3690–3700, Dec. 2017. doi: 10.1109/TII.2017.2786307. [Google Scholar] [CrossRef]
76. J. Ping, S. J. Chen, N. Zhang, Z. Yan, and L. Z. Yao, “Decentralized transactive mechanism in distribution network based on smart contract,” Proc. CSEE, vol. 37, no. 13, pp. 878–889, Dec. 2017. doi: 10.13334/j.0258-8013.pcsee.170374. [Google Scholar] [CrossRef]
77. M. Shahidehpour, M. Yan, P. Shikhar, S. Bahramirad, and A. Paaso, “Blockchain for peer-to-peer transactive energy trading in networked microgrids: Providing an effective and decentralized strategy,” IEEE Electrific. Mag., vol. 8, no. 4, pp. 80–90, Dec. 2020. doi: 10.1109/MELE.2020.3026444. [Google Scholar] [CrossRef]
78. A. Hahn, R. Singh, C. C. Liu, and S. J. Chen, “Smart contract-based campus demonstration of decentralized transactive energy auctions,” presented at 2017 IEEE Pow. Energy Soc. Innov. Smart Grid Technol. Conf. (ISGTWashington, DC, USA, Apr. 2017. doi: 10.1109/ISGT.2017.8086092. [Google Scholar] [CrossRef]
Cite This Article
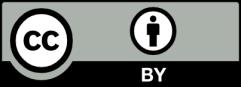
This work is licensed under a Creative Commons Attribution 4.0 International License , which permits unrestricted use, distribution, and reproduction in any medium, provided the original work is properly cited.