Open Access
ARTICLE
Joint Estimation of SOH and RUL for Lithium-Ion Batteries Based on Improved Twin Support Vector Machineh
1 School of Electrical and Information Engineering, Beijing University of Civil Engineering and Architecture, Beijing, 100044, China
2 Institute of Distributed Energy Storage Safety Big Data, Beijing, 100044, China
3 Beijing Key Laboratory of Intelligent Processing for Building Big Data, Beijing, 100044, China
* Corresponding Author: Hongyan Ma. Email:
(This article belongs to the Special Issue: Advanced Modelling, Operation, Management and Diagnosis of Lithium Batteries)
Energy Engineering 2025, 122(1), 243-264. https://doi.org/10.32604/ee.2024.057500
Received 19 August 2024; Accepted 29 October 2024; Issue published 27 December 2024
Abstract
Accurately estimating the State of Health (SOH) and Remaining Useful Life (RUL) of lithium-ion batteries (LIBs) is crucial for the continuous and stable operation of battery management systems. However, due to the complex internal chemical systems of LIBs and the nonlinear degradation of their performance, direct measurement of SOH and RUL is challenging. To address these issues, the Twin Support Vector Machine (TWSVM) method is proposed to predict SOH and RUL. Initially, the constant current charging time of the lithium battery is extracted as a health indicator (HI), decomposed using Variational Modal Decomposition (VMD), and feature correlations are computed using Importance of Random Forest Features (RF) to maximize the extraction of critical factors influencing battery performance degradation. Furthermore, to enhance the global search capability of the Convolution Optimization Algorithm (COA), improvements are made using Good Point Set theory and the Differential Evolution method. The Improved Convolution Optimization Algorithm (ICOA) is employed to optimize TWSVM parameters for constructing SOH and RUL prediction models. Finally, the proposed models are validated using NASA and CALCE lithium-ion battery datasets. Experimental results demonstrate that the proposed models achieve an RMSE not exceeding 0.007 and an MAPE not exceeding 0.0082 for SOH and RUL prediction, with a relative error in RUL prediction within the range of [−1.8%, 2%]. Compared to other models, the proposed model not only exhibits superior fitting capability but also demonstrates robust performance.Keywords
Cite This Article
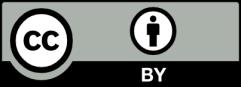
This work is licensed under a Creative Commons Attribution 4.0 International License , which permits unrestricted use, distribution, and reproduction in any medium, provided the original work is properly cited.