Open Access
REVIEW
Hydrogen Energy Storage System: Review on Recent Progress
1 Research Centre for Sustainable Technologies, Faculty of Engineering, Computing and Science, Swinburne University of Technology, Jalan Simpang Tiga, Kuching, Sarawak, 93350, Malaysia
2 College of Engineering, Faculty of Computing, Engineering and the Built Environment, Birmingham City University, Birmingham, West Midlands, England, B4 7XG, UK
* Corresponding Author: Hadi Nabipour Afrouzi. Email:
Energy Engineering 2025, 122(1), 1-39. https://doi.org/10.32604/ee.2024.056707
Received 29 July 2024; Accepted 27 September 2024; Issue published 27 December 2024
Abstract
A hydrogen energy storage system (HESS) is one of the many rising modern green innovations, using excess energy to generate hydrogen and storing it for various purposes. With that, there have been many discussions about commercializing HESS and improving it further. However, the design and sizing process can be overwhelming to comprehend with various sources to examine, and understanding optimal design methodologies is crucial to optimize a HESS design. With that, this review aims to collect and analyse a wide range of HESS studies to summarise recent studies. Two different collections of studies are studied, one was sourced by the main author for preliminary readings, and another was obtained via VOSViewer. The findings from the Web of Science platform were also examined for a more comprehensive understanding. Major findings include the People’s Republic of China has been active in HESS research, as most works and active organizations originate from this country. HESS has been mainly researched to support power generation and balance load demands, with financial analysis being the common scope of analysis. MATLAB is a common tool used for HESS design, modelling, and optimization as it can handle complex calculations. Artificial neural network (ANN) has the potential to be used to model the HESS, but additional review is required as a form of future work. From a commercialization perspective, pressurized hydrogen tanks are ideal for hydrogen storage in a HESS, but other methods can be considered after additional research and development. From this review, it can be implied that modelling works will be the way forward for HESS research, but extensive collaborations and additional review are needed. Overall, this review summarized various takeaways that future research works on HESS can use.Keywords
Nomenclature
AFC | Alkaline Fuel Cell |
ANN | Artificial Neural Network |
ANN-GA | Artificial Neural Network-Genetic Algorithm |
ASEAN | Association of Southeast Asian Countries |
BESS | Battery Energy Storage System |
COE | Cost of Energy |
CA | Cultural Algorithm |
DC | Direct Current |
DMFC | Direct Methanol Fuel Cell |
EMS | Energy Management Systems |
ESS | Energy Storage System |
FC | Fuel Cell |
GA | Genetic Algorithm |
GA-PSO | Genetic Algorithm-Particle Swarm Optimization |
GHG | Greenhouse Gas |
HRES | Hybrid Renewable Energy System |
HESS | Hydrogen Energy Storage System |
ICE | Internal Combustion Engine |
LMS | Least Mean Square |
LCOE | Levelized Cost of Energy |
LOCs | Liquid Organic Carriers |
LPSP | Loss of Power Supply Probability |
MILP | Mixed-Integer Linear Programming |
MCFC | Molten Carbonate Fuel Cell |
MOPSO | Multi-Objective Particle Swarm Optimization |
NSGA-II | Non-Dominated Sorting Genetic Algorithm II |
PSO | Particle Swarm Optimization |
PAFC | Phosphoric Acid Fuel Cell |
P2G | Power-2-Gas |
PtP | Power-to-Power |
RE | Renewable Energy |
SLO | Sea Lion Optimizer |
SOFC | Solid Oxide Fuel Cell |
SMES | Super Conducting Magnetic Energy Storage |
USA | United States of America |
In this modern age, energy generation from renewable sources has become a necessity for major stakeholders to provide clean and sustainable energy. Renewable sources such as solar, hydropower, and biomass have been utilized and commercialized by electrical utility companies. This has led to increased penetration of renewable energy (RE) in grids, as seen in members of the Association of Southeast Asian Countries (ASEAN) expected to increase their respective generation capacities by 2023 and certain European countries increased their respective RE penetration to the grid by more than 50% [1]. With that, an increase in power generation may cause excess or insufficient energy within a power system, especially with intermittent sources such as solar and wind [2]. Excess energy will get wasted if it is not used when it could be used at moments of higher energy demands. Not only that but grid stability can be affected when there is a high penetration of renewable sources, with up to 20% penetration of intermittent renewable sources having the potential to destabilize the grid [3]. Hence an energy storage system (ESS) is crucial to minimize economic losses while addressing possible grid disruptions such as instability [4].
As the name suggests, an ESS stores excess energy and releases it when necessary. The stored energy can be in various forms, depending on the type of ESS used. Storage types can range from hydropower via pumped hydro energy storage, superconducting magnetic energy storage (SMES), batteries, and hydrogen energy [5]. Hydrogen energy is highly favoured as hydrogen itself can be used for various purposes such as electric mobility. A hydrogen energy system would mainly contain a fuel cell (FC) to generate electricity from hydrogen, a hydrogen tank to store excess hydrogen, and a mechanism to generate hydrogen [6]. This mechanism can be either a reformer which takes in gaseous sources [7,8] or electrolysers which perform electrolysis on water to obtain hydrogen [6]. The former may need carbon-heavy sources, hence not favoured in a race for carbon neutrality. This makes the hydrogen energy storage system (HESS) an ideal choice to decarbonise a grid while allowing increased capacity of RE generation. Hydrogen storage can also be further categorized depending on how the hydrogen is stored, such as in the form of metal hydrides [9] or gaseous state [10]. The storage method would depend on the usage of hydrogen as hydrogen can be used in various methods, such as using magnesium hydrides for automotive applications [9] and combustion of hydrogen gas [10]. Besides energy storage and opening wider hydrogen applications, HESS can be used for matters such as power quality management and peak shaving.
Naturally, ESS would have challenges of its own such as low acceptance towards ESS from the industry and financial feasibility [5]. Sizing of ESS is another major challenge as proper sizing of ESS can increase the efficiency of ESS and minimize energy curtailment [4]. However, with a variety of works being done, it can be overwhelming to identify the proper sizing method for an ESS, as there are various uncertainties such as power generation and load demand [8]. With hydrogen becoming an important key for green development, optimal design methods for HESS are necessary for future researchers and designers. On top of this, there has yet to be a review of design methodologies for HESS in recent years.
With that, this review paper investigates the design and analysis methodologies of HESS. Through this review, a better understanding of the recent developments of HESS can be understood from the academic research point of view as well as the necessary technical and supporting knowledge such as the types of hydrogen produced. A literature review would be done, along with preliminary sourcing of recent works for initial understanding and to provide a basis for comparisons with the works that would be sourced from Web of Science via VOSViewer. The results from VOSViewer would then be analysed to provide an answer to each of the objectives of this review.
This review will elaborate deeply on the technical knowledge regarding HESS as well as the technical and economic parameters analysed, hence beneficial for researchers who are new to this topic. The modelling methodologies would also be elaborated and summarized based on sourced materials, further helping those who are new and unfamiliar with HESS. Finally, another unique characteristic of this review is that a comparison between the sourced recent works from the literature review would be compared with the sourced recent works selected from the collected materials by Web of Science, allowing a comparison of what was done and examined by various researchers.
2 Components and Progress of Hydrogen Energy Storage System
Fundamentally, a HESS would need a hydrogen generation system and a hydrogen storage system. Hydrogen is a versatile medium and can be used in various applications. In the context of this review, HESS acts as an energy supplier as well, hence a power generation system such as FC that uses hydrogen as its fuel source is considered as well. On top of these major systems, a balance of systems such as water treatment systems, gas compressors and expansion valves, and energy management systems (EMS) are required for the optimal function of the HESS [11–13]. Regardless, this section will cover the three previously mentioned major systems, which are the FC, hydrogen generation, and hydrogen storage. This section will also cover recent works on HESS, as well as optimization methodologies used to optimize the designed HESS.
An FC is a device that converts chemical energy from hydrogen into electrical energy via an ionization process [6,14]. As stated in a review by Yue et al. [6], when hydrogen is supplied to the FC, ionization occurs, generating electrons which flow within a circuit, hence producing electrical current for the electrical equipment. Electricity generated by the FC is direct current (DC), hence a drop in the DC voltage generated may cause overmodulation between the grid and FC, distorting the output waveform [15]. The ionization process and how electricity is generated from FC can be illustrated via a general diagram of the FC below, Fig. 1 [13,16,17].
Figure 1: General structure of fuel cell. Adapted from References [13,16,17]
Hydrogen is supplied to the anode side of the FC as its fuel source whereas oxygen is supplied to the cathode side of the FC [13,16,17]. When hydrogen reaches the anode, hydrogen releases electrons which travel through the electrical load via an external circuit. The electrons would reach the cathode side of the FC, undergoing a chemical reaction with protons and oxygen to produce water and heat due to the chemical reaction [13,16]. The electrolyte is responsible for enabling the movement of hydrogen ions between the anode and cathode [17]. The chemical equations involved in the functional concept of FC can be summarized in Table 1, as observed below [16].
As technology advances, so too a variety of FCs have been introduced. Examples of FCs include permeable exchange membrane fuel cells (PEMFC), alkaline fuel cells (AFC), solid oxide fuel cells (SOFC), molten carbonate fuel cells (MCFC), and phosphoric acid fuel cells (PAFC) [18]. The type of FC to be used is dependent on what electrolyte is being used. For example, MCFC will have alkali carbonate as its electrolyte whereas SOFC uses solid-non-porous metal oxide [19]. As different FCs have different internal components, it is common to have various studies on them such as heat transfer modelling, system integration, and materials [20]. The different internal components may lead to each FC having unique characteristics, such as a direct methanol fuel cell (DMFC) needing methanol as its input instead of hydrogen and an MCFC releasing carbon dioxide as its reactant [21].
As a component responsible for producing electricity, FC is vital in a HESS should hydrogen be used for a power-to-power (PtP) concept. The overmodulation issue presented by Guo et al. [15] can contribute to harmonics and oscillation which can cascade to the grid system. Guo has presented a possible solution in the form of a rate limiter for active power to prevent overmodulation. Besides this characteristic, its high cost must be addressed to further enable a hydrogen economy [17,19].
Most of the credit towards the invention of FC goes to Welsh chemical physicist Sir William Robert Grove [22,23]. He discovered that an electrical current is generated and flows through an external circuit when two platinum electrodes are immersed in a dilute sulfuric acid solution and there is a chemical reaction between hydrogen and oxygen gases. This “gas battery” model would be named the “Grove Cell” and became the basis of FC today. Even before this discovery came, Humphry Davy contributed to the concept of FC as well in the early 19th century [23].
Splitting of ions via electrolysis has always been the common consideration for hydrogen generation to encourage decarbonization initiatives. However, recent advances have diversified the methods of generating hydrogen on top of pre-existing methodologies. A review of recent technologies for hydrogen generation by Faye et al. [24] identified several methods which can be considered. Reformation into either hydrocarbon or steam can be used if non-renewable sources such as fossil fuel are being used and methods such as gasification are suitable if renewable sources are being used. On top of this, there are methods such as thermolysis and photolysis [25]. Regardless, due to the abundance of water and encouraged decarbonization initiatives, electrolysis on water is still considered a reasonable method.
Depending on the source used and production methodology, the production method can be categorized accordingly, as presented in a review by Ishaq et al. [26]. Blue hydrogen is defined as using carbon-heavy resources to produce hydrogen, such as gas reformation and coal gasification. Purple hydrogen utilizes nuclear energy [26] whereas green hydrogen relies on RE and electrolysis [27]. The other types of hydrogen such as grey and brown hydrogen [16,26–28] can be tabulated in Table 2, as shown below, and their respective brief explanation.
A review by Sarker et al. [25] found that most hydrogen produced is blue hydrogen from natural gas, with the remainder mostly being grey hydrogen produced from coal. Regardless, hydrogen generation with minimal to no emission is still an important element towards sustainable development, and this sentiment is shared in other various reviews [27,28]. With that, green hydrogen has the potential to be an important element to achieve long-term sustainable development.
Due to the need to enable optimal hydrogen production, research on utilizing algorithms to optimize hydrogen production systems was done, specifically with Artificial Neural Network (ANN) [29]. According to a review by Mohammad, ANN models can study and generalize non-linear relationships between inputs and outputs. This makes ANN models adaptive to unclear inputs and produces accurate results. However, the authors did bring up various challenges in using ANN, namely quantity and accuracy of data, misassumptions, poor decision-making, and economic constraints. The authors have also proposed implementing hybrid methodologies, such as combining ANN with other algorithms to improve the results of a study.
After hydrogen is produced, a storage system is required so the installed FC would have an immediate supply for electricity generation when hydrogen consumption needs to be higher than hydrogen production. With that, various storage methods have been developed and investigated, ranging from pressurized tanks to underground caverns.
Compressed hydrogen storage is one of the older techniques, with its usage first documented around 1880 for military purposes, with hydrogen stored at a pressure of 12 MPa in steel cylinders [14]. Technological advancements have enabled compressed hydrogen stored at higher pressure levels, such as up to 70 MPa. This method of storage is deemed the simplest method for hydrogen storage due to no chemical reactions needed, hence went through rapid development and achieved technological maturity [30,31]. Compressed hydrogen storage is characterized by its low cost and energy consumption; however, it presents a few possible safety hazards due to possible hydrogen leaks [27,31]. Depending on the technical requirements and purpose, various steel cylinders have been commercialized; namely Type I, Type II, Type III, and Type IV [30,32]; their respective main characteristics are tabulated as shown in Table 3 below.
Besides the gaseous state, hydrogen can also be stored in a liquid state. Liquid hydrogen storage would involve cryogenic technology, where liquid hydrogen is cooled and kept under a very low temperature of 21.2 K due to the low critical temperature of hydrogen of 33 K [14]. Storing hydrogen at a temperature lower than the critical temperature would prevent hydrogen from returning to its original gaseous state. The liquefaction process of hydrogen is time-consuming and energy-intensive [27,30], but it offers better safety and storage duration than compressed hydrogen storage [14]. A review led by Ma et al. [33] commented that liquid hydrogen storage is very promising as a hydrogen storage method due to its large gravimetric energy density and low volumetric densities. Liquefaction and compressed hydrogen storage are the most established and common methods used as current practices [34].
Another method used to store hydrogen by bonding hydrogen with metals via chemical reactions, creating metal hydrides which make it easier to transport hydrogen. Metal hydrides have been explored since the late 1960s due to the fuel crisis encouraging the development of hydrogen-focused transportation systems [14]. Since then, researchers have explored metal hydrides for a wide range of applications due to their various advantages such as environmentally benign and high volumetric storage [34,35]. The general concept about metal hydrides is that hydrogen separates itself into atoms that would enter the metallic lattice of its attached metal as atoms, diffusing itself through the material and becoming a metal hydride after some modifications to the lattice structure of the metal [14]. Different metal hydrides offer different characteristics, hence a thorough understanding of the numerous metal elements and how they react to hydrogen is crucial.
On top of these methods, there are several other methods researchers have explored for hydrogen storage. Methods including liquid-organic carriers [28], geological storage [33], and natural gas pipelines [33] have been discussed by researchers in both reviews and original research works. The effectiveness and feasibility of these methods would depend on various factors such as climate, available technology, and intention of storing hydrogen.
A review led by Hatem et al. [36] agrees that high-pressure storage is the most mature technology for hydrogen storage, with most of the hydrogen refuelling stations in the world using this as their method of hydrogen storage. Liquefaction technology can also be used by turning hydrogen from its gaseous form into liquid and using cryo-technology to freeze it, but the high energy demand for the liquefaction process and loss of hydrogen when converted back from liquid to gas are the major concerns behind this method. Besides, relying on solid materials to store hydrogen can also be considered. Metal hydrides can bond hydrogen with the metal material via the adsorption process, which can then be used in applications where metal hydrides can be used such as fuel for internal combustion engines (ICE) [10]. Alternatively, hydrogen can be absorbed into materials, producing hydrogen ions which would be assimilated into the lattice structure of a solid. While promising, costs would be high and still mostly being researched before commercialization.
Another review from a team led by Wan et al. [37] also reviewed the methods discussed by Hatem. They agree that high-pressure storage is the more common approach for its direct process. For the liquefaction process, they believe that this process is ideal for large-scale high-density hydrogen storage such as HESS. By turning hydrogen from gaseous form into liquid form, there is a risk of evaporation, hence loss rate of liquid hydrogen would need to be considered. As for solid materials, the energy required for the conversion process would be much lower than the two former suggestions. However, the general mass-energy density of the material involved would be low and metal hydrides are too heavy for transportation. On top of that, metal hydrides could face material poisoning, decreasing their storage capacity.
Reviews done by Tang et al. [28] and Faye et al. [24] have investigated these three methods and shared the same sentiment with the previously discussed works. Tang extended the listed variety of methodologies, adding liquid organic carriers (LOCs) as a possible storage method. Hydrogen is injected into an organic molecule that does not have hydrogen and a reactor would be used to dehydrogenate the LOCs, releasing hydrogen. It is possible to utilize current gas pipelines with this method, as there is research on using gas pipelines to store hydrogen [38]. However, according to a review by Jia et al. [39], embrittlement may occur on the pipelines, potentially causing leakage and affecting safety protocols. On the other hand, Faye explained the possibility of directly cooling hydrogen gas via cryogenics, but the tanks designed to handle such gas have yet to be commercially available. Simultaneously, Jain [10] also shared some similar sentiments such as gaseous hydrogen storage being the most common and simplest technology, and that the hydrogen liquefaction process requires high energy consumption for state conversion from gaseous state to liquid state.
2.4 Recent Reviews on Hydrogen Energy Storage System
RE sources, especially solar and wind, are still deemed the best for a HESS. European countries were found to have high curtailment of RE production due to developments of RE sources being faster than the capabilities of supplying RE power into the grid [6]. Countries such as China have also been found to have massive amounts of energy, which could have been used for hydrogen generation [40]. Dependency on RE sources is also crucial for decarbonization initiatives [41], hence combined with hydrogen being an energy carrier, decreased carbon emissions can be achieved. HESS has been gaining more recognition globally with increasing policies for decarbonization and RE technologies [33]. However, due to current technological limitations, most of the hydrogen produced for the HESS is considered blue hydrogen, as stated by Sarker et al. [25], hence relying on carbon-heavy resources to power the hydrogen production [41]. This is further evident as most countries are focusing their attention on both low-carbon blue hydrogen and green hydrogen for their respective development [30]. Regardless, the review done by Lagioia and the team has also commented that the importance of blue hydrogen could be temporary as it may prepare the future transition from blue hydrogen to green hydrogen. Hydrogen itself still has a lot of advantages such as its versatility as both an energy provider for FC and combustion, as well as manufacturing for industries such as food and chemical [28]. HESS is also superior to other types of ESS such as battery ESS and pumped hydro storage in terms of storage duration and general capacity, with capacities ranging from 10 MWh to 100 GWh for a 12-year lifespan [42]. On top of global policies and encouragement, the large-scale production of green hydrogen and its implementation into a HESS may become a commercialized reality.
One review led by Arsad et al. [43] found that a HESS can be combined with BESS, forming a hybrid ESS that offers higher flexibility and dependability, better bus bar stability, and optimal management on rapid loads and intermittent generation peaks. This does come with a drawback of higher complexity. Choudhury [44] supported this drawback, stating that the control technique for a hybrid ESS is more complex than a single-variant ESS. Factors such as charge and discharge rates, life span, and efficiency must be considered before combining several variants of ESS. On top of this, most of the technologies to support HESS are limited by various factors such as cost [45] and the need for additional research [43]. The disadvantages of HESS and the complexity of hybrid ESS have made commercial development and deployment of grid-connected ESS, especially hybrid ESS, challenging. Regardless, discussions on ESS remain strong as it still brings potential benefits that can see fruition in the future. Hence, an understanding of sizing methodologies is required to ensure future researchers and developers have a better understanding of designing a HESS, regardless of whether it is used in a hybrid ESS or as a single-variant ESS.
Countries across the globe have taken various measures to develop some form of HESS, either via projects, policies, or active research. Countries such as Germany and Japan established roadmaps for their respective countries to establish themselves as leaders in the global hydrogen economy [33]. People’s Republic of China is actively involved in academic research, as a review found that it has been one of the most active countries in the academic research of hydrogen [46]. Saudi Arabia plans to develop a megacity powered purely by renewable sources [33], but it faces energy storage issues that can only be addressed by HESS [47]. This raises the need for additional feasibility research on HESS to ensure optimal design, development, execution, operation, and maintenance.
2.5 Preliminary Investigations of Scope of Study
Before commencing the bibliographic study via VOSViewer and Web of Science, some preliminary investigations were done to obtain several sources and have an initial understanding of the research scope. This initial understanding was used to compare with the eventual findings from VOSViewer and Web of Science.
Several documents were compiled and examined before determining which were suitable for further preliminary readings. 18 research works from different databases were selected based on their relevance. They were then evaluated thoroughly in various aspects such as keywords, scope of study, and results. These documents are works done respectively by Crespi et al. [48], Wang et al. [49], Kharel et al. [50], Tephiruk et al. [51], Huang et al. [52], Utomo et al. [53], Wen et al. [54], Colbertaldo et al. [55], Teng et al. [56], Zhao et al. [57], Pan et al. [58], Luo et al. [59], Calise et al. [60], Tay et al. [61], Esteban et al. [62], Wu et al. [63], Li [64], and Meng et al. [65]. These studies can be categorized based on their respective country of focus, as observed in Table 4, as observed below. Their respective year of publication are also tabulated.
From Table 4, 9 sources came from the People’s Republic of China, 2 sources came from Italy, and another 2 sources came from Australia. The oldest document came from 2018 while most documents came after 2020. Only 3 works were from before 2020, with 1 from 2018 and 2 from 2019. Besides the general characteristics, technical characteristics were identified from these 18 sources, such as the type of software used and the purpose of introducing HESS. MATLAB is the most common software used, with 11 works relying on MATLAB, and 2 relying on Simulink. HOMER Pro is the second most common software used, albeit only 2 sources were observed. Other software found included GAMS, TRNSYS, and DigSILENT PowerFactory. 2 works did not explicitly state the software used.
There are various purposes to have HESS, as seen in Fig. 2. Some authors such as Esteban et al. [62] tried to minimize dependency on grid imports on a microgrid. On the other hand, teams led by Wu et al. [63] and Wen et al. [54] respectively aimed to further encourage RE generation, mainly solar and wind, by storing excess generation in the form of hydrogen. Balancing load demand and energy generation was another purpose of proposing the HESS, as seen by a team led by Utomo et al. [53] and Colbertaldo et al. [55], respectively. Overall, most of the studies were focused on power generation such as minimizing power curtailment [54,56], or on supporting the grid such as minimizing grid dependency [62]. There are other purposes for investigating HESS such as to balance the load demands [55,57], for comparison with BESS and hybrid ESS [61], and to promote a hydrogen economy [59].
Figure 2: Distribution among the studies on their reasoning for having HESS
Almost all the works examined the performance of HESS with intermittent sources, mainly solar and wind. Huang et al. [52] were the only authors who examined HESS with hydropower as its source of power generation. As for the proposed storage method, Wu et al. [63] proposed salt caverns to store hydrogen, while the rest of the works used hydrogen tanks.
Several modelling and optimization techniques were introduced in these works. Some purely relied on equations and MATLAB to directly model and optimize their proposed system, while others such as Li [64] used algorithms such as Genetic Algorithm (GA) to size their system accordingly. Particle Swarm Optimization (PSO) appears to be a common optimization algorithm used, as seen by teams led by Wang et al. [49], Huang et al. [52], and Wu et al. [63], respectively. Mixed-integer linear programming (MILP) is another approach used, as seen by Crespi et al. [48], Tay et al. [61], and Luo et al. [59] in their respective works.
Finally, there is an apparent similarity in analysing the HESS despite the various methodologies, which can be observed in Fig. 3. Most notably, the cost-effectiveness of the HESS is commonly discussed, especially when compared to hybrid ESS and BESS. Observation was also done on the RE generation sources to encourage RE generation. Some such as Colbertaldo [55] and Pan [58] examined the ideal capacity of the HESS components such as FC, electrolyzer, and hydrogen tank. 9 sources looked at financial matters regarding the HESS, such as payback period [60] and cost of energy (COE) [50], whereas 6 sources investigated how the HESS affects the grid such as connection flexibility [54] and energy flow within the grid [60]. To summarize the Top 3 most common scopes of analysis from the 18 works, the effect of HESS on power generation was evaluated by 5 sources, mainly on mitigating power curtailment.
Figure 3: Scope of analysis from the 18 sources
From the preliminary investigations, GA and PSO algorithms are commonly used in optimizing the sizing of the HESS. This section will elaborate further on these two algorithms, as well as the potential of using the hybrid genetic algorithm-particle swarm optimization (GA-PSO) algorithm in optimization works.
The inspiration behind GA is the crossing-over of genetics between chromosomes. In the case of GA, the chromosomes are the solutions that can solve the objective function [66]. After identifying the objective functions, the solutions are generated and evaluated based on the objective functions. The solutions are chosen based on their fitness, determined by how well they address the objective functions. The fitter solutions are then selected to generate a new population via crossover and mutation functions. The process continues until the termination criterion is satisfied. The flowchart below, Fig. 4, displays the described process of GA.
Figure 4: Flowchart process of genetic algorithm
An improved variant of the GA, the non-dominated sorting genetic algorithm II (NSGA-II), designed to tackle multi-objective problems [67], as seen by Liu in minimizing levelized COE (LCOE) and loss of power supply probability (LPSP) of the proposed system. NSGA-II utilizes a sorting approach that ranks the individuals based on non-dominated sorting and a sorting metric of crowded distance that examines the population density of solutions with similar ranks [68]. The flowchart below, Fig. 5, elaborates on the procedures and process in the NSGA-II algorithm, as well as pinpoints where the said sorting function is done [68].
Figure 5: Flowchart process of NSGA-II
Similar to GA, a group of individuals is created, and their fitness is assessed. The fitter individuals are chosen as parents, where crossover and mutation operations are done to produce offspring. If the number of offspring required has not been reached, additional offspring would be produced. Both parents and offspring solutions are ranked based on non-dominated sorting [68], and should the algorithm not reach maximum generation yet, new individuals are chosen to repeat the process until the maximum generations have been obtained.
GA and its variants share a similar trait, whereby it is suitable for optimizations having discrete variables, but it is slow [69]. Hence, researchers adopted hybrid strategies to address the weaknesses of individual algorithms. Further explanations can be found in other sub-sections.
2.6.2 Particle Swarm Optimization Algorithm
PSO is a swarm-based algorithm that takes inspiration from the social behaviour of animals moving in large groups, or a “swarm” [70]. Each solution is treated as a particle moving at a certain velocity that then meets another moving particle. These particles exchange information to determine their next movement within the search space [71]. This process will repeat itself until they have reached their optimal result, or optimal. The process will also end if the algorithm has reached sufficient iterations. The flowchart below, Fig. 6, graphically illustrates the said explanation.
Figure 6: Flowchart process of particle swarm optimization
Just like GA, PSO has a multi-objective variant, which is the Multi-Objective PSO (MOPSO). MOPSO maintains the capabilities of PSO and extends it by adding Pareto dominance to select a set of leaders to lead the movement of particles to the optimal solutions [68]. A repository is used to store the solutions, and a global leader is chosen based on the score of hypercubes via the roulette wheel selection method. Fig. 7 below is a flowchart that illustrates MOPSO based on the explanation.
Figure 7: Flowchart of MOPSO
Ibanez-Rioja [72] used PSO to optimize an off-grid solar-BESS-HESS system and achieved similar results to GA but at a faster rate than GA. The optimization was restricted by minimizing the cost of hydrogen production. This finding is consistent with the statement made by Khan [69], that PSO has a fast convergence speed. However, PSO may suffer from early convergence, hence the particles may be trapped in a local optimum and not generate optimized results [71]. Hence, like GA, PSO is used with other algorithms to cover its weaknesses, forming hybrid algorithms.
As stated in the previous sections, each algorithm has strengths and weaknesses. PSO may suffer from early convergence and be trapped in a local optimum, whereas GA has a slower convergence speed. Hence, researchers considered combining these two algorithms to compensate for each other’s weaknesses and produce a more optimal result. A review done by Dziwinski et al. [73] elaborated several strategies on how multiple optimization algorithms can work together in a hybrid algorithm, such as one algorithm is used to initialize the other algorithm, multiple algorithms operate at the same time, the algorithms operate sequentially, and solutions of one algorithm are modified by the operators of another algorithm.
For reference, a team led by Ghorbani et al. [74] used both GA and PSO to minimize total NPC while addressing minimal LPSP. They executed both GA and PSO, then compared which of the two algorithms produced a more optimal result, in which the better result is remembered and used for subsequent iterations until the termination criterion is satisfied. They then compared the GA-PSO combination with MOPSO and found that the GA-PSO approach produced the most ideal optimization. It must be noted that the GA-PSO algorithm is a single-objective algorithm, hence LPSP is converted to cost by considering its weighted cost multiplied by penalty factor.
On the other hand, work done by Lei et al. [75] combined PSO and NSGA-II to optimize a wind-PV-HESS system. PSO is used to optimize the parameters for NSGA-II, which is then used to address multiple objectives, which are to minimize economic cost, maximize wind and PV power consumption, and minimize LPSP. This strategy was found to have better performance than the NSGA-II approach, where the hybrid algorithm achieved a better crossover ratio and higher probability of variation, hence achieving better results.
As hybrid algorithms can compensate for the weaknesses of individual algorithms, hybrid algorithms are the way forward for future optimization works, as observed by Memon et al. [76]. Hybrid algorithms are also seen in works outside of ESS, such as cloud computing [77] and demand management of a smart grid [78]. Hence, hybrid algorithms should be more emphasized in discussions for optimization works.
2.6.4 Artificial Neural Networks
As shared by Abdelkareem et al. [29], ANN is an excellent tool for optimization. ANN takes inspiration from how the human brain processes thoughts [79]. Just as neurons carry small pieces of information and share it with other neurons via synaptic connections, the ANN fundamentally consists of nodes grouped into layers for information sharing. The ANN model would need some training data sets to “learn” how it should “think”, but once its training is complete, it can be used to perform several arithmetic processes such as data classification, recognition, optimization, data association, and predictions [80].
Abdelkareem et al. [29] has also shared that ANN can be combined with other algorithms to improve results and minimize errors. One review [29] compiled several studies and found a few of them opted for a hybrid combination with both ANN and other optimization algorithms such as GA. Yilmaz et al. [80] have also used the artificial neural network-genetic algorithm (ANN-GA) combination to optimize an integrated geothermal-solar-HESS system. ANN was used to do the initial modelling of the system, while GA was used for optimization. The same strategy was used by Izadi et al. [81] to optimize an HRES consisting of PV, wind turbines, and HESS for a building.
The main method for this review would be collecting academic sources from various reputable databases via the Web of Science and filtering out the documents based on the determined criteria. The criteria for the selection are as follows:
1. The academic works must be published at most 9 years ago from the time of writing, which was in 2023, hence works from 2015 up to September 2023 are considered.
2. The documents must be in English and not translated from their original language.
3. The sources must be review papers or journal articles categorized under the electrical and electronics engineering field.
As part of the selection process, several keywords are identified. Table 5 below shows the main keywords used and their respective alternative forms.
There is a limitation to the possible number of exported documents. A total of 205,319 works were identified from the search on the Web of Science platform. However, due to the time constraints in exporting the documents, only the first 30,000 documents with the highest relevance were chosen for the bibliographic study. After obtaining the documents, they were then analysed in VOSViewer. Various settings in VOSViewer were adjusted such as type of analysis, counting method, and unit of analysis to observe a variety of graphics and categorizations.
The output from VOSViewer can be observed in the screenshot below, Fig. 8. The initial output is based on the keywords of the documents sourced.
Figure 8: Network visualization of results based on keywords
By observation, several keywords can be grouped in one cluster. The collection of clusters indicates how related the keywords are to each other. Another observation that can be made is that some keywords have a more significant presence amongst the pool of documents over other keywords. These observations can be further presented in the subsequent sub-sections. Simultaneously, several documents from the output can be examined for further understanding and this examination will also be elaborated in a subsection.
Some adjustments were made to present the keywords in an overlay visualization based on the year of publication, as seen in Fig. 9. The keywords “classification” and “voltage control” are found to be different from the other keywords. On average, they are present in publications earlier than 2021, with “classification” being more significant in late 2017 and “voltage control” in early 2019. Generally, modelling-related keywords such as “neural networks” and “data models” are more significant in the later years, mainly from 2020 onwards.
Figure 9: Overlay visualization based on keywords and average year of publication
There are 5 clusters in the network shown in Fig. 8. Each of these clusters contains keywords that are associated with each other. These clusters can be tabulated as shown in below, Table 6.
Cluster 1 focused on electrical engineering aspects in designing a HESS, with terms such as “voltage control” and “harmonic analysis”. On the other hand, Clusters 2, 3, 4, and 5 grouped the various terms related to data science and constructing models. Hence, terms such as “neural network”, “convolution”, “data models” and “computational modelling” are present. Despite the apparent similarity, the four latter clusters have differences.
Cluster 2 identified terms related to extracting information from a set of data, hence terms such as “task analysis” and “convolution” are grouped. On the other hand, Cluster 3 focused on terms related to building a model, based on terms such as “deep neural networks” and “deep learning”. Next, Cluster 4 delved deeper into neural networks, with terms such as “convolutional neural networks”, “training”, and “computational modelling” grouped. Finally, Cluster 5 grouped terms that allow researchers to have informative insights into their data, such as “principal component analysis” and “natural language processing”.
4.2 Characteristics of Keywords
Occurrences and total link strength are the characteristics that define the importance of the keywords obtained. Occurrences are defined as how frequently the keywords appear whereas total link strength indicates how strongly connected they are with the sample size. Hence, the tables below display keywords with the highest total link strength and highest occurrences, Tables 7 and 8, respectively.
From the two tables above, the general approach towards designing HESS can be inferred. The keywords “task analysis”, “feature extraction”, “training”, “deep learning”, and “computational modelling” are the Top 5 highest total link strengths whereas “task analysis”, “deep learning”, “feature extraction”, “optimization”, and “machine learning” are the Top 5 highest occurrences. Other noticeable keywords include “data models”, and “computational modeling”.
4.3 Summary of Selected Studies from Pool of Documents
Of the 205,319 documents, some documents were selected for additional reading. These documents are used as a basis for comparison with previously examined documents. A total of 17 documents were randomly selected and examined and their summary can be tabulated as such below. The table below, Table 9, also categorizes the documents based on their country of focus. It must be mentioned that these documents are the respective works done by Tazay et al. [82], Jing et al. [83], Abdelsalam et al. [84], Suresh et al. [85], Khezzane et al.[86], Azoug et al. [87], de Oliveira et al. [88], Zahedi et al. [89], Nguyen et al. [90], Wang et al. [91], Hussain et al. [92], Mehdi et al. [93], Monforti et al. [94], Bouabdallah et al. [95], Seward et al. [96], Saib et al. [97], and You et al. [98].
From the 17 documents, several observations can be obtained. For example, Hussain et al. [92] investigated from the perspective of hydrogen production and refuelling facilities whereas Seward et al. [96] designed an off-grid HRES. Investigations on HESS were done on a pressurized hydrogen tank design. 2 of the selected documents do not cover HESS [70,88], but matters related to the topic which is sizing of ESS via using algorithms such as GA [86] and PSO [97]. The characteristics of the other 15 documents are summarized in the following paragraphs.
From Table 9, despite the variety of works presented, most of them use similar software to each other such as MATLAB and GAMS. Other software such as TRNSYS was found, as seen in the work led by Monforti et al. [94]. The usage of algorithms such as NSGA-II [95], GA [86] and PSO [82,97] was observed in these works as well. The reasoning for including HESS in their respective works can be visually recorded in the graph below.
From Fig. 10, most documents justified using HESS in the system mainly due to either power generation, balancing load demands, or supporting the grid. Each category contained 4 documents, whereas 1 document focused on supporting the hydrogen economy [92] and another compared the performance of HESS with BESS [96]. Besides the reasoning for including HESS, the scope of analysis for these works can be observed and similarly, visually represented. Hence, the graph below shows the areas analysed from these works.
Figure 10: Distribution among selected documents on their reasoning for having HESS
When analysing the HESS and graphically displaying the results in Fig. 11, most of the selected documents examined the ideal size of the HESS, followed by its financial matters. Works by authors such as Nguyen et al. [90] combined some of the other scopes into their main scope. For the case of Nguyen, the ideal size of the HESS is identified based on the system’s dependency on grid imports and LCOE. Like the initial readings, some of the works had multiple angles of analysis for the HESS. For example, Gabriel et al. [88] examined the electrical characteristics and financial impact of the proposed HESS and compared them with the proposed BESS for a grid-connected energy system.
Figure 11: Scope of analysis from the 15 selected documents
There are two types of geographic findings: one is based on the country of origin of the publications while the other is their respective organizations. This section will categorize the results based on these two types.
4.4.1 Findings Based on Countries
Several tables are used to tabulate different categories, namely highest number of documents, highest number of citations, and highest total link strength. These tables are as shown below; Table 10 for the number of documents, Table 11 for the number of citations, and Table 12 for total link strength.
From the tables, the major contributors to the sample pool are the People’s Republic of China and the United States of America (USA), as they take the Top 2 positions in all three categories. India is another major contributor as it is third in two of the categories, and fourth highest total link strength. Other contributing countries included Australia, Italy, Canada, France, Iran, England, South Korea, and Germany, indicating a mix of mostly Asian and European countries. Besides these values, a network visualization can be observed, as seen below, in Fig. 12.
Figure 12: Network visualization of results based on countries
The different colours represent different clusters, and the varying sizes represent how many documents each country has. Reflective on the tabulated results, the People’s Republic of China, the USA, and India have significantly larger circles than the other countries as they have the most publications. These three countries are also seen collaborating with a wide variety of countries. However, some countries are significantly further away from the centre cluster such as Mauritius, Philippines, and Argentina. Mauritius and Kenya are among the countries that have no connecting lines to any other countries.
4.4.2 Findings Based on Organizations
Besides countries, the major contributing organizations can also be identified. Similar to the categorization by countries, the organizations can be arranged in terms of the number of documents, the highest number of citations, and the highest total link strength. With that, the tables below, Tables 13–15, show the results of the top-performing organizations based on these criteria respectively.
From Tables 13–15, the Chinese Academy of Sciences is the most contributive organization, as it has the largest pool of documents, most citations, and the highest total link strength. Other major contributing organizations originate from the People’s Republic of China, such as Tsinghua University and the University of Electronic Science and Technology of China. There are non-Chinese organizations that contributed majorly to the collected sample pool, such as the University of California, Berkeley from the USA and Islamic Azad University from Iran.
The network visualization of results based on organization can be observed in Fig. 13.
Figure 13: Network visualization of results based on organizations
Similar to the countries-based network, some organizations do not have any connecting lines to any other organizations. The weight of visualization is based on the number of published documents; hence Chinese Academy of Sciences has the largest circle in this network.
An interesting observation is that the organizations generally collaborate with other organizations that are geographically close to them. For example, as seen in Fig. 14, Nanyang Technology University from Singapore have collaborations with other organizations from Singapore such as the National University Singapore and Agency Science Technology and Research. Nanyang Technology University has collaborations with organizations such as Auckland University Technology from New Zealand, The University of Sydney from Australia, and Zhejiang University from the People’s Republic of China, all of which are geographically closer to Singapore as opposed to countries such as Iran, England, and USA which are geographically further.
Figure 14: Nanyang technology university and its collaborative partners
From the collected resources, MATLAB appears to be the common software used to model and simulate the HESS. As sizing and optimization of HESS are considered complex calculations, high computational power is required, and MATLAB fulfils this requirement. Other software such as HOMER Pro, GAMS, and TRNSYS were found, but there are insufficient sources to justify them besides MATLAB. Hence, as both sizing and optimization are required, MATLAB is deemed the ideal choice. MATLAB also has access to relevant optimization toolboxes, especially for GA and PSO, hence enhancing the advantages of using MATLAB for HESS modelling research works.
As for the storage method, a pressurized hydrogen tank is a commonly used approach to store hydrogen for the HESS among the 15 observed works. This observation is in line with the reviews observed in the literature review [26,28,36], where a hydrogen tank is a common method for its high maturity. Other methods such as salt caverns can be considered, but they have yet to reach an age where it is commercially possible to implement it. Hence, hydrogen tanks are the common choice of investigation for HESS for their practicality. Regardless, other forms of hydrogen storage have potential, but they require more intensive research to understand their respective technical and economic feasibility. Hence, methods such as liquefaction and metal hydrides should not be completely ruled out from large-scale commercial applications.
When it comes to the usage of algorithms, a variety of algorithms have been identified such as PSO [82,97], GA [86], and NSGA-II [95]. GA and PSO were also found in the initial literature readings. GA is an algorithm whereby genetic operations such as selection, mutation, and genetic crossover are applied to some data to obtain an optimized result. Simultaneously, the PSO algorithm iteratively moves a group of potential solutions through a search space for a set number of iterations or until a convergence is achieved. Both algorithms are commonly found in both the literature documents and obtained documents, hence proving their popularity in optimizing the size of the HESS. The sizing of HESS can be done directly via equations, but optimizations are to be done with the assistance of equations, especially in works that aim to investigate the ideal size of the HESS. Usage of data analysis methods is also apparent from the pool of keywords, where keywords such as “neural networks”, “deep learning”, and “convolution” are observed.
From the results obtained via VOSViewer, neural network-related keywords have significant appearances. Some studies were identified to be using ANN for modelling purposes, followed by using GA to optimize the results [80,81]. Due to how the ANN algorithm functions, using ANN for modelling purposes is promising. However, ANN was not mentioned as often as GA and PSO from the sampled works in this context, hence this may suggest additional reviews on ANN modelling for HESS and hybrid ANN modelling would be needed in future work.
5.2 Inference from Observed Keywords
By observing the keywords obtained, neural networks are commonly discussed, as terms such as “training” and “deep learning” are associated with the development of neural networks. A neural network is a machine learning model analogous to the nature of the human brain and its interconnected nodes. In a neural network, each neuron is organized into layers via weighted connections, and eventually, an outer layer produces the result. To produce the outer layer, a training process is done, whereby the neural network adjusts the weights of the connections based on mathematical errors between the prediction and actual outcome. From this understanding and the presented keywords, neural networks are essential to the development and study of HESS. Neural network modelling is evident as most keyword clusters are directed to mathematical modelling. Combined with most modelling-related keywords having consistent appearances from 2020 onwards, modelling-focused HESS studies are envisioned to be more significant in the future.
Another observable phenomenon is the term “convolution” and its potential connection with neural networks via “convolutional neural networks”. In the context of data modelling, convolution is a mathematical operation that combines two sets of information to generate a third set of information. Hence, in a convolutional neural network, layers are combined to capture significant features. The capture is done via kernels on the incoming layer to filter the input data. Hence, convolution is a technique that can be used to develop neural networks and achieve optimal results.
Finally, an inference can be made whereby the design and optimization of HESS requires strong computation power, as seen with the term “computational modelling”. Referencing the literature and selected documents, MATLAB is the most used software. This would further solidify that HESS modelling and optimization require high computational power, and MATLAB can fulfil this requirement along with its available optimization toolboxes.
5.3 Analysis and Objective on Proposed Systems
Both the literature review and results have observed similar reasons in implementing HESS. Both categories of documents have listed power generation, system support, and balancing demand to be the major reasons in adding the HESS. This reasoning is similar to non-HESS studies, whereby Saib implemented BESS to support HRES in selling electricity to the grid [97] and Khezzane implemented the BESS to store excess electricity from the HRES [86]. With that, the analysis of HESS would not be too different from the analysis of BESS under similar circumstances.
Besides the three major reasons, minimizing power curtailment is another major reason for implementing HESS. Referencing back to high RE power curtailment issue [6], it is possible to link this challenge with the capabilities of HESS using the excess energy to produce hydrogen and then relying on FC to generate electrical energy from the produced hydrogen, especially given that high amounts of energy is required to produce hydrogen. Mainly, intermittent sources such as solar and wind were used as sources for the HESS as they are more susceptible to energy wastage. There are not many studies on using controlled sources such as hydropower to operate HESS, hence it will be insightful to investigate such a combination.
Regarding the scope of analysis on the HESS, financial matters is the major scope from both the literature documents and obtained documents. This scope is resonant to one of major issues raised in commercializing HESS, which is its high costs [45]. With that, optimizations were done to minimize the costs of HESS, such as the work by Wu et al. [63] where the PSO algorithm optimized the system to be more cost-effective. Similarly, Nguyen et al. [90] performed iterative calculations to ensure that the system meets the criteria of minimal LCOE and high system reliability. Hence, economic analysis should be considered, and its results are related to technical specifications.
5.4 Geographic Characteristics
From the collected sample pool, the People’s Republic of China is the dominant country in HESS research and modelling. Similarly, from the preliminary readings, the People’s Republic of China was found to be a popular country for HESS research, implying sources for future literature reviews on HESS would most likely originate from this country. Most contributing organizations such as the Chinese Academy of Sciences and Tsinghua University originate from this country, further reinforcing the People’s Republic of China as a leader in the research of HESS modelling. The review by Ozsari [46] has also identified that the People’s Republic of China is one of the most contributive towards academic research on HESS. Other countries such as Iran and England are also actively involved in researching and developing HESS.
In the bigger picture, some countries and organizations have not collaborated with any external party. Organizations collaborate with partners that are geographically close to them. This may limit knowledge sharing which can affect the speed of HESS research and development. These organizations should encourage a wider range of collaborations regardless of geographical factors so that researchers can learn from each other and adopt various approaches and practices that can advance the promising development of HESS. The major leading countries for HESS research and modelling, namely the People’s Republic of China, the USA, and India, have the potential to encourage wider collaborations as they have the activeness and resources.
Meanwhile, other growing countries involved in HESS research such as Iran, Malaysia, and England should try to collaborate with countries with little collaborative work. This can expedite knowledge sharing and open opportunities for more and varied collaborations. The same can be said for organizations and they should not limit themselves to collaborative work with peers within their geographic network.
6 Conclusion, Recommendations, and Future Work
This bibliographic review aimed to shed light on the design methodologies of HESS. With that, initial readings were done to provide initial understanding while VOSViewer was used to examine extracted documents from Web of Science based on set parameters. Results include a pool of academic works, their common keywords and their respective interconnections. Analysis was done by examining the relationship between the keywords and comparisons between some of the extracted documents and preliminary readings. The country and organization of origin of these documents were also studied. Data modelling is an essential skill for researching HESS, as ANN is found to have a connection with HESS modelling. On top of this, HESS models are commonly optimized by GA and PSO algorithms, where either one is used or combined as a hybrid optimization algorithm. With that, MATLAB is generally used for HESS modelling and optimization studies. HESS is investigated to generate power for a system, supporting a generating system to take in excess power and balance the load and generation demands. Like any ESS, HESS is considered to minimize power curtailment, especially on intermittent sources such as solar and wind. From a geographic point of view, the People’s Republic of China is a major contributor to the research on HESS modelling. Finally, economic analysis is commonly investigated to examine the financial feasibility of the proposed HESS. As technical specifications directly affect financial results, technical and economic analysis were often discussed. This review can be expanded with possible future work such as additional bibliographic review tools, a review of potential applications of the various physical states of hydrogen, usage of ANN, and an in-depth discussion on current hydrogen projects from industry players and global leaders. With that, this review successfully answered the methodologies to design and optimize HESS models. It is hoped that this review shared updated insights on sizing methodologies and analysis of HESS, contributing to future research.
Acknowledgement: The authors would like to thank and acknowledge the Research Center of Sustainable Technologies of Swinburne University of Technology (Sarawak Campus).
Funding Statement: The authors received no specific funding for this study.
Author Contributions: The authors confirm contribution to the paper as follows: Conception, data collection, analysis, writing—draft and editing: Millenium Wong; Writing—editing and advisor: Hadi Nabipour Afrouzi. All authors reviewed the results and approved the final version of the manuscript.
Availability of Data and Materials: The authors confirm that the data used in this study is available on request.
Ethics Approval: Not applicable.
Conflicts of Interest: The authors declare that they have no conflicts of interest to report regarding the present study.
References
1. O. Erixno, N. A. Rahim, F. Ramadhani, and N. N. Adzman, “Energy management of renewable energy-based combined heat and power systems: A review,” Sustain. Energy Technol. Assess., vol. 51, 2022. doi: 10.1016/j.seta.2021.101944. [Google Scholar] [CrossRef]
2. M. Frankowska, A. Rzeczycki, M. Sowa, and W. Drożdż, “Functional model of power grid stabilization in the green hydrogen supply chain system—Conceptual assumptions,” Energies, vol. 16, no. 1, 2023, Art. no. 154. doi: 10.3390/en16010154. [Google Scholar] [CrossRef]
3. M. Y. Worku, “Recent advances in energy storage systems for renewable source grid integration: A comprehensive review,” Sustainability, vol. 14, no. 10, 2022. doi: 10.3390/su14105985. [Google Scholar] [CrossRef]
4. K. M. Tan, T. B. Sudhakar, V. K. Ramachandaramurthy, P. Kasinathan, S. G. Solanki and S. K. Raveendran, “Empowering smart grid: A comprehensive review of energy storage technology and application with renewable energy integration,” J. Energy Storage, vol. 39, no. 18, 2021, doi: 10.1016/j.est.2021.102591. [Google Scholar] [CrossRef]
5. B. Pinnangudi, M. Kuykendal, and S. Bhadra, “4—Smart grid energy storage,” in The Power Grid: Smart, Secure, Green and Reliable, B. W. D’Andrade (Ed.Washington, DC, USA: Elsevier, 2017, pp. 93–135. [Google Scholar]
6. M. Yue, H. Lambert, E. Pahon, R. Roche, S. Jemei and D. Hissel, “Hydrogen energy systems: A critical review of technologies, applications, trends and challenges,” Renew. Sustain. Energy Rev., vol. 146, no. 34, 2021. doi: 10.1016/j.rser.2021.111180. [Google Scholar] [CrossRef]
7. F. Ramadhani, M. A. Hussain, and H. Mokhlis, “A comprehensive review and technical guideline for optimal design and operations of fuel cell-based cogeneration systems,” Processes, vol. 7, no. 12, 2019. doi: 10.3390/pr7120950. [Google Scholar] [CrossRef]
8. M. A. Hannan, M. Faisal, J. K. Pin, R. A. Begum, Z. Y. Dong and C. Zhang, “Review of optimal methods and algorithms for sizing energy storage systems to achieve decarbonization in microgrid applications,” Renew. Sustain. Energy Rev., vol. 131, 2020. doi: 10.1016/j.rser.2020.110022. [Google Scholar] [CrossRef]
9. I. P. Jain, C. Lal, and A. Jain, “Hydrogen storage in Mg: A most promising material,” Int. J. Hydrog. Energy, vol. 35, no. 10, pp. 5133–5144, 2009. doi: 10.1016/j.ijhydene.2009.08.088. [Google Scholar] [CrossRef]
10. I. P. Jain, “Hydrogen the fuel for 21st century,” Int. J. Hydrog. Energy, vol. 34, no. 17, pp. 7368–7378, 2009. doi: 10.1016/j.ijhydene.2009.05.093. [Google Scholar] [CrossRef]
11. A. Bavo, N. Poli, A. Trobo, G. Marini, and M. Guarnieri, “Hydrogen energy storage system in a multi‒technology microgrid: Technical features and performance,” Int. J. Hydrog. Energy, vol. 48, no. 32, pp. 12072–12088, 2023. doi: 10.1016/j.ijhydene.2022.11.346. [Google Scholar] [CrossRef]
12. M. Lamagna, A. F. Monforti, D. G. Astiaso, S. Mcphail, and G. Comodi, “Reversible solid oxide cell coupled to an offshore wind turbine as a poly-generation energy system for auxiliary backup generation and hydrogen production,” Energy Rep., vol. 8, no. 19, pp. 14259–14273, 2022. doi: 10.1016/j.egyr.2022.10.355. [Google Scholar] [CrossRef]
13. Y. Wang, X. Yang, Z. Sun, and Z. Chen, “A systematic review of system modeling and control strategy of proton exchange membrane fuel cell,” Energy Reviews, vol. 3, no. 1, 2023. doi: 10.1016/j.enrev.2023.100054. [Google Scholar] [CrossRef]
14. M. K. Sarmah, T. P. Singh, P. Kalita, and A. Dewan, “Sustainable hydrogen generation and storage—A review,” RSC Adv., vol. 13, no. 36, pp. 25253–25275, 2023. doi: 10.1039/D3RA04148D. [Google Scholar] [PubMed] [CrossRef]
15. R. Guo, Q. Li, and N. Zhao, “An overview of grid-connected fuel cell system for grid support,” Energy Rep., vol. 8, 10, pp. 884–892, 2022. doi: 10.1016/j.egyr.2022.05.211. [Google Scholar] [CrossRef]
16. J. Li, T. Wu, C. Cheng, J. Li, and K. Zhou, “A review of the research progress and application of key components in the hydrogen fuel cell system,” Processes, vol. 12, no. 2, 2024. doi: 10.3390/pr12020249. [Google Scholar] [CrossRef]
17. T. L. Thanh et al., “Fueling the future: A comprehensive review of hydrogen energy systems and their challenges,” Int. J. Hydrog. Energy, vol. 54, pp. 791–816, 2023. doi: 10.1016/j.ijhydene.2023.08.044. [Google Scholar] [CrossRef]
18. L. Fan, Z. Tu, and S. H. Chan, “Recent development of hydrogen and fuel cell technologies: A review,” Energy Rep., vol. 7, no. 2, pp. 8421–8446, 2021. doi: 10.1016/j.egyr.2021.08.003. [Google Scholar] [CrossRef]
19. M. K. Singla, P. Nijhawan, and A. S. Oberoi, “Hydrogen fuel and fuel cell technology for cleaner future: A review,” Environ. Sci. Pollut. Res., vol. 28, no. 13, pp. 15607–15626, 2021. doi: 10.1007/s11356-020-12231-8. [Google Scholar] [PubMed] [CrossRef]
20. N. Sazali, W. N. W. Salleh, A. S. Jamaludin, and M. N. M. Razali, “New perspectives on fuel cell technology: A brief review,” Membranes, vol. 10, no. 5, 2020, Art. no. 99. doi: 10.3390/membranes10050099. [Google Scholar] [PubMed] [CrossRef]
21. A. T. Haseeb, S. A. A. Kazmi, H. Muhammad, S. A. A. Muhammed, and M. Anwar, “Analysis of fuel cell integration with hybrid microgrid systems for clean energy: A comparative review,” Int. J. Hydrog. Energy, vol. 52, pp. 1005–1034, 2023. doi: 10.1016/j.ijhydene.2023.07.238. [Google Scholar] [CrossRef]
22. E. I. Ortiz-Rivera, A. L. Reyes-Hernandez, and R. A. Febo, “Understanding the history of fuel cells,” presented at the 2007 IEEE Conf. His. Elec. Pow., Newark, NJ, USA, 2008. [Google Scholar]
23. A. Ozturk, R. A. Gultekin, S. Erkan, and A. Y. Bayrakceken, “Chapter 1—Introduction to fuel cells,” in Direct Liquid Fuel Cells Fundamentals, Advances and Future, UK: Academic Press, 2021, pp. 1–47. [Google Scholar]
24. O. Faye, J. Szpunar, and U. Eduok, “A critical review on the current technologies for the generation, storage, and transportation of hydrogen,” Int. J. Hydrog. Energy, vol. 47, no. 29, pp. 13771–13802, 2022. doi: 10.1016/j.ijhydene.2022.02.112. [Google Scholar] [CrossRef]
25. A. K. Sarker, A. K. Azad, M. G. Rasul, and A. T. Doppalapudi, “Prospect of green hydrogen generation from hybrid renewable energy sources: A review,” Energies, vol. 16, no. 3, 2023, Art. no. 1556. doi: 10.3390/en16031556. [Google Scholar] [CrossRef]
26. H. Ishaq, I. Dincer, and C. Crawford, “A review on hydrogen production and utilization: Challenges and opportunities,” Int. J. Hydrog. Energy, vol. 47, no. 62, pp. 26238–26264, 2022. doi: 10.1016/j.ijhydene.2021.11.149. [Google Scholar] [CrossRef]
27. L. Ge et al., “A review of hydrogen generation, storage, and applications in power system,” J. Energy Storage, vol. 75, 2023, doi: 10.1016/j.est.2023.109307. [Google Scholar] [CrossRef]
28. D. Tang et al., “State-of-the-art hydrogen generation techniques and storage methods: A critical review,” J. Energy Storage, vol. 64, 2023. doi: 10.1016/j.est.2023.107196. [Google Scholar] [CrossRef]
29. M. A. Abdelkareem et al., “Progress of artificial neural networks applications in hydrogen production,” Chem. Eng. Res. Des., vol. 182, pp. 66–86, 2022. doi: 10.1016/j.cherd.2022.03.030. [Google Scholar] [CrossRef]
30. P. Muthukumar et al., “Review on large-scale hydrogen storage systems for better sustainability,” Int. J. Hydrog. Energy, vol. 48, no. 85, pp. 33223–33259, 2023. doi: 10.1016/j.ijhydene.2023.04.304. [Google Scholar] [CrossRef]
31. E. Kalihonda, E. Igbineweka, and S. Chowdhury, “Integrating hydrogen as an energy storage for renewable energy systems: A comprehensive review,” presented at the 2024 32nd Sout. Afri. Univ. Pow. Engin. Conf., Stellenbosch, South Africa, 2024. [Google Scholar]
32. B. T. -L. Chukwudi and S. N. Godfrey, “Hydrogen production, distribution, storage and power conversion in a hydrogen economy—A technology review,” Chem. Eng. J. Adv., vol. 8, no. 2, 2021, doi: 10.1016/j.ceja.2021.100172. [Google Scholar] [CrossRef]
33. N. Ma, W. Zhao, W. Wang, X. Li, and H. Zhou, “Large scale of green hydrogen storage: Opportunities and challenges,” Int. J. Hydrog. Energy, vol. 50, no. 1, pp. 379–396, 2023. doi: 10.1016/j.ijhydene.2023.09.021. [Google Scholar] [CrossRef]
34. W. F. Ekpotu, J. Akintola, M. C. Obialor, and U. Philemon, “Historical review of hydrogen energy storage technology,” World J. Eng. Technol., vol. 11, no. 3, pp. 454–475, 2023. doi: 10.4236/wjet.2023.113033. [Google Scholar] [CrossRef]
35. N. Klopcic, I. Grimmer, F. Winkler, M. Sartory, and A. Trattner, “A review on metal hydride materials for hydrogen storage,” J. Energy Storage, vol. 72, 2023. doi: 10.1016/j.est.2023.108456. [Google Scholar] [CrossRef]
36. Z. R. Hatem and S. Rajakaruna, “A review on hydrogen storage techniques and their applications in novel initiatives in australia,” presented at the 2022 IEEE Sus. Pow. and Ener. Conf., Perth., Australia, 2022. [Google Scholar]
37. L. Wan, W. Zhang, and Z. Xu, “Overview of key technologies and applications of hydrogen energy storage in integrated energy systems,” presented at the 2020 12th IEEE PES Asia-Pacific Power Energy Eng. Conf., Nanjing, China, 2020. [Google Scholar]
38. J. J. Brey, “Use of hydrogen as a seasonal energy storage system to manage renewable power deployment in Spain by 2030,” Int. J. Hydrog. Energy, vol. 46, no. 33, pp. 17447–17457, 2021. doi: 10.1016/j.ijhydene.2020.04.089. [Google Scholar] [CrossRef]
39. G. Jia et al., “Hydrogen embrittlement in hydrogen-blended natural gas transportation systems: A review,” Int. J. Hydrog. Energy, vol. 48, no. 82, pp. 32137–32157, 2023. doi: 10.1016/j.ijhydene.2023.04.266. [Google Scholar] [CrossRef]
40. S. J. Wang, Z. Y. Zhang, Y. Tan, K. X. Liang, and S. H. Zhang, “Review on the characteristics of existing hydrogen energy storage technologies,” Energy Sources, Part A: Recov., Util., Environ. Effects, vol. 45, no. 1, pp. 985–1006, 2022. doi: 10.1080/15567036.2023.2175938. [Google Scholar] [CrossRef]
41. G. Lagioia, M. S. Pia, and V. Amicarelli, “Blue and green hydrogen energy to meet European Union decarbonisation objectives. An overview of perspectives and the current state of affairs,” Int. J. Hydrog. Energy, vol. 48, no. 4, pp. 1304–1322, 2023. doi: 10.1016/j.ijhydene.2022.10.044. [Google Scholar] [CrossRef]
42. E. Venugopal, P. Sivakumar, A. Raghul, R. Priyadharshini, C. Indhumathi and E. Parthasarathy, “A review of electrical energy storage system,” presented at the 2023 9th Int. Conf. Adv. Comp. Comm. Sys., Coimbatore, India, 2023. [Google Scholar]
43. A. Z. Arsad et al., “Hydrogen energy storage integrated hybrid renewable energy systems: A review analysis for future research directions,” Int. J. Hydrog. Energy, vol. 47, no. 39, pp. 17285–17312, 2022. doi: 10.1016/j.ijhydene.2022.03.208. [Google Scholar] [CrossRef]
44. S. Choudhury, “Review of energy storage system technologies integration to microgrid: Types, control strategies, issues, and future prospects,” J. Energy Storage, vol. 48, 2022. doi: 10.1016/j.est.2022.103966. [Google Scholar] [CrossRef]
45. T. Egeland-Eriksen, A. Hajizadeh, and S. Sartori, “Hydrogen-based systems for integration of renewable energy in power systems: Achievements and perspectives,” Int. J. Hydrog. Energy, vol. 46, no. 63, pp. 31963–31983, 2021. doi: 10.1016/j.ijhydene.2021.06.218. [Google Scholar] [CrossRef]
46. I. Ozsari, “Trend analysis and evaluation of hydrogen energy and hydrogen storage research,” Energy Storage, vol. 5, no. 6, pp. 46, 2023. doi: 10.1002/est2.471. [Google Scholar] [CrossRef]
47. A. Boretti, “A market opportunity in power generation for hydrogen energy storage systems,” Int. J. Hydrog. Energy, vol. 49, no. 33, pp. 166–172, 2023. doi: 10.1016/j.ijhydene.2023.07.162. [Google Scholar] [CrossRef]
48. E. Crespi, P. Colbertaldo, G. Guandalini, and S. Campanari, “Design of hybrid power-to-power systems for continuous clean PV-based energy supply,” Int. J. Hydrog. Energy, vol. 46, no. 26, pp. 13691–13708, 2021. doi: 10.1016/j.ijhydene.2020.09.152. [Google Scholar] [CrossRef]
49. Y. Wang, Y. G. Li, X. Sun, and S. Zhao, “Research on high proportion renewable energy of hybrid energy storage containing hydrogen storage,” presented at the IECON 2021—47th Ann. Conf. IEEE Indus. Elec. Soc., Toronto, ON, Canada, 2021. [Google Scholar]
50. S. Kharel and B. Shabani, “Hydrogen as a long-term large-scale energy storage solution to support renewables,” Energies, vol. 11, no. 10, 2018, Art. no. 2825. doi: 10.3390/en11102825. [Google Scholar] [CrossRef]
51. N. Tephiruk, P. Jamjang, A. Taweesap, and K. Hongesombut, “Hybrid energy storage system to enhance efficiency of renewable energy usage,” presented at the 2022 19th Int. Conf. Elec. Eng./Electron., Comput., Telecommun., Inf. Tech., Prachuap Khiri Khan, Thailand, 2022. [Google Scholar]
52. J. Huang, W. Li, X. Wu, and Z. Gu, “A bi-level capacity planning approach of combined hydropower hydrogen system,” J. Clean. Prod., vol. 327, no. 6054, 2021, doi: 10.1016/j.jclepro.2021.129414. [Google Scholar] [CrossRef]
53. O. Utomo, M. Abeysekera, and C. E. Ugalde-Loo, “Optimal operation of a hydrogen storage and fuel cell coupled integrated energy system,” Sustainability, vol. 13, no. 6, 2021, doi: 10.3390/su13063525. [Google Scholar] [CrossRef]
54. T. Wen, Z. Zhang, X. Lin, Z. Li, C. Chen and Z. Wang, “Research on modeling and the operation strategy of a hydrogen-battery hybrid energy storage system for flexible wind farm grid-connection,” IEEE Access, vol. 8, pp. 79347–79356, 2020. doi: 10.1109/ACCESS.2020.2990581. [Google Scholar] [CrossRef]
55. P. Colbertaldo, S. B. Agustin, S. Campanari, and B. Jack, “Impact of hydrogen energy storage on California electric power system: Towards 100% renewable electricity,” Int. J. Hydrog. Energy, vol. 44, no. 19, pp. 9558–9576, 2019. doi: 10.1016/j.ijhydene.2018.11.062. [Google Scholar] [CrossRef]
56. Y. Teng, Z. Wang, Y. Li, Q. Ma, Q. Hui and S. Li, “Multi-energy storage system model based on electricity heat and hydrogen coordinated optimization for power grid flexibility,” CSEE J. Power Energy Syst., vol. 5, no. 22019. doi: 10.17775/CSEEJPES.2019.00190. [Google Scholar] [CrossRef]
57. P. Zhao, W. Xu, A. Liu, W. Wu, J. Wang and X. Wang, “Assessment the hydrogen-electric coupled energy storage system based on hydrogen-fueled CAES and power-to-gas-to-power device considering multiple time-scale effect and actual operation constraints,” Int. J. Hydrog. Energy, vol. 48, no. 25, pp. 9198–9218, 2023. doi: 10.1016/j.ijhydene.2022.12.097. [Google Scholar] [CrossRef]
58. J. Pan, J. Lei, A. Chen, Y. Cao, Z. Zhao and H. Zhang, “Optimal proportion of wind, PV, hydrogen and storage capacity based on time sequence simulation,” presented at the 2022 5th Int. Conf Pow. Energy Appl., Guangzhou, China, 2022. [Google Scholar]
59. W. Luo, J. Wu, J. Cai, Y. Mao, and S. Chen, “Capacity allocation optimization framework for hydrogen integrated energy system considering hydrogen trading and long-term hydrogen storage,” IEEE Access, vol. 11, pp. 15772–15787, 2022. doi: 10.1109/ACCESS.2022.3228014. [Google Scholar] [CrossRef]
60. F. Calise, F. L. Cappiello, L. Cimmino, M. D. A. Dentice, and M. Vicidomini, “Dynamic simulation and thermoeconomic analysis of a hybrid renewable system based on PV and fuel cell coupled with hydrogen storage,” Energies, vol. 14, no. 22, 2021. doi: 10.3390/en14227657. [Google Scholar] [CrossRef]
61. S. L. Tay, N. N. Tuan, D. -K. Bui, and D. N. Tuan, “Optimal sizing of renewable energy storage: A techno-economic analysis of hydrogen, battery and hybrid systems considering degradation and seasonal storage,” Appl. Energy, vol. 336, no. 9–10, 2023, doi: 10.1016/j.apenergy.2023.120817. [Google Scholar] [CrossRef]
62. J. V. L. Esteban, A. Mazza, E. Pons, H. Lok, and E. Bompard, “Modelling and control of a grid-connected RES-hydrogen hybrid microgrid,” Energies, vol. 14, no. 6, 2021. doi: 10.3390/en14061540. [Google Scholar] [CrossRef]
63. F. Wu, R. Gao, C. Li, and J. Liu, “A comprehensive evaluation of wind-PV-salt cavern-hydrogen energy storage and utilization system: A case study in Qianjiang salt cavern, China,” Energy Convers. Manag., vol. 277, 2023, doi: 10.1016/j.enconman.2022.116633. [Google Scholar] [CrossRef]
64. B. Li, “Build 100% renewable energy based power station and microgrid clusters through hydrogen-centric storage systems,” presented at the 2020 4th Int. Conf. HVDC, Xi’an, China, 2020. [Google Scholar]
65. Z. Meng, Q. He, X. Shi, D. Cao, and D. Du, “Research on energy utilization of wind-hydrogen coupled energy storage power generation system,” Sep. Purif. Technol., vol. 313, no. 6, 2023, Art. no. 123439. doi: 10.1016/j.seppur.2023.123439. [Google Scholar] [CrossRef]
66. A. Slowik and H. Kwasnicka, “Evolutionary algorithms and their applications to engineering problems,” Neural Comput Appl., vol. 32, no. 16, pp. 12363–12379, 2020. doi: 10.1007/s00521-020-04832-8. [Google Scholar] [CrossRef]
67. T. Liu, Z. Yang, Y. Duan, and S. Hu, “Techno-economic assessment of hydrogen integrated into electrical/thermal energy storage in PV+ Wind system devoting to high reliability,” Energy Convers. Manag., vol. 268, 2022, doi: 10.1016/j.enconman.2022.116067. [Google Scholar] [CrossRef]
68. I. E. Atawi, A. Abuelrub, A. Q. Al-Shetwi, and O. H. Albalawi, “Design of a wind-PV system integrated with a hybrid energy storage system considering economic and reliability assessment,” J. Energy Storage, vol. 81, 2024, doi: 10.1016/j.est.2023.110405. [Google Scholar] [CrossRef]
69. T. Khan, M. Yu, and M. Waseem, “Review on recent optimization strategies for hybrid renewable energy system with hydrogen technologies: State of the art, trends and future directions,” Int. J. Hydrog. Energy, vol. 47, no. 60, pp. 25155–25201, 2022. doi: 10.1016/j.ijhydene.2022.05.263. [Google Scholar] [CrossRef]
70. T. M. Shami, A. A. El-Saleh, M. Alswaitti, Q. Al-Tashi, M. S. Amen and S. Mirjalili, “Particle swarm optimization: A comprehensive survey,” IEEE Access, vol. 10, no. 4, pp. 10031–10061, 2022. doi: 10.1109/ACCESS.2022.3142859. [Google Scholar] [CrossRef]
71. A. G. Gad, “Particle swarm optimization algorithm and its applications: A systematic review,” Arch. Computat. Methods Eng., vol. 29, no. 5, pp. 2531–2561, 2022. doi: 10.1007/s11831-021-09694-4. [Google Scholar] [CrossRef]
72. A. Ibáñez-Rioja et al., “Simulation methodology for an off-grid solar-battery–water electrolyzer plant: Simultaneous optimization of component capacities and system control,” Appl. Energ., vol. 307, 2022. doi: 10.1016/j.apenergy.2021.118157. [Google Scholar] [CrossRef]
73. P. Dziwinski and L. Bartczuk, “A new hybrid particle swarm optimization and genetic algorithm method controlled by fuzzy logic,” IEEE Trans. Fuzzy Syst., vol. 28, no. 6, pp. 1140–1154, 2019. doi: 10.1109/TFUZZ.2019.2957263. [Google Scholar] [CrossRef]
74. N. Ghorbani, A. Kasaeian, A. Toopshekan, L. Bahrami, and A. Maghami, “Optimizing a hybrid wind-PV-battery system using GA-PSO and MOPSO for reducing cost and increasing reliability,” Energy, vol. 154, no. 4, pp. 581–591, 2018. doi: 10.1016/j.energy.2017.12.057. [Google Scholar] [CrossRef]
75. L. Xing and Y. Liu, “An optimization capacity design method of wind/photovoltaic/hydrogen storage power system based on PSO-NSGA-II,” Energ. Eng., vol. 120, no. 4, pp. 1023–1043, 2022. doi: 10.32604/ee.2023.025335. [Google Scholar] [CrossRef]
76. S. A. Memon and R. N. Patel, “An overview of optimization techniques used for sizing of hybrid renewable energy systems,” Renew. Energy Focus, vol. 39, pp. 1–26, 2021. doi: 10.1016/j.ref.2021.07.007. [Google Scholar] [CrossRef]
77. A. M. K. Senthil, K. Parthiban, and S. S. Shankar, “An efficient task scheduling in a cloud computing environment using hybrid Genetic Algorithm-Particle Swarm Optimization (GA-PSO) algorithm,” presented at the 2019 Int. Conf. Intell. Sustain. Syst., Palladam, India, 2019. [Google Scholar]
78. C. Roy and D. K. Das, “A hybrid genetic algorithm (GA)–particle swarm optimization (PSO) algorithm for demand side management in smart grid considering wind power for cost optimization,” Sādhanā, vol. 46, 2021, Art. no. 101. doi: 10.1007/s12046-021-01626-z. [Google Scholar] [CrossRef]
79. A. S. Ramesh et al., “Artificial intelligence driven hydrogen and battery technologies—A review,” Fuel, vol. 337, 2023. doi: 10.1016/j.fuel.2022.126862. [Google Scholar] [CrossRef]
80. C. Yilmaz and O. Sen, “Thermoeconomic analysis and artificial neural network based genetic algorithm optimization of geothermal and solar energy assisted hydrogen and power generation,” Int. J. Hydrog. Energy, vol. 47, no. 37, pp. 16424–16439, 2022. doi: 10.1016/j.ijhydene.2022.03.140. [Google Scholar] [CrossRef]
81. A. Izadi, M. Shahafve, and P. Ahmadi, “Neural network genetic algorithm optimization of a transient hybrid renewable energy system with solar/wind and hydrogen storage system for zero energy buildings at various climate conditions,” Energy Convers. Manag., vol. 260, 2022. doi: 10.1016/j.enconman.2022.115593. [Google Scholar] [CrossRef]
82. A. F. Tazay, M. M. Samy, and S. Barakat, “A techno‐economic feasibility analysis of an autonomous hybrid renewable energy sources for university building at Saudi Arabia,” J. Electr. Eng. Technol., vol. 15, no. 6, pp. 2519–2527, 2020. doi: 10.1007/s42835-020-00539-x. [Google Scholar] [CrossRef]
83. K. Jing, C. Liu, X. Cao, H. Sun, and Y. Dong, “Analysis, modeling and control of a non-grid-connected source-load collaboration wind-hydrogen system,” J. Electr. Eng. Technol., vol. 16, no. 5, pp. 2355–2365, 2021. doi: 10.1007/s42835-021-00774-w. [Google Scholar] [CrossRef]
84. R. A. Abdelsalam, Y. S. Abdallah, N. M. Shabar, O. A. Afifi, M. H. Fouda, and A. A. El-Deib, “Energy management and techno-economic optimization of an isolated hybrid AC/DC microgrid with green hydrogen storage system,” presented at the 2023 IEEE Conf. Power Electron. Renew. Energy, Luxor, Egypt, 2023. [Google Scholar]
85. S. K. Suresh, N. C. M. Mukundan, and P. Jayaprakash, “Modified LMS control for a grid interactive PV-fuel cell-electrolyzer hybrid system with power dispatch to the grid,” IEEE Trans. Ind. Appl., vol. 58, no. 6, pp. 7907–7918, 2022. doi: 10.1109/TIA.2022.3200926. [Google Scholar] [CrossRef]
86. K. Khezzane, M. D. Lamine, and F. Khoucha, “Multi-objective sizing of hybrid generation energy system for remote area using genetic algorithm,” presented at the 2021 Sixteenth Int. Conf. Ecol. Veh. Renew. Energy, Monte-Carlo, Monaco, 2021. [Google Scholar]
87. H. Azoug, H. Belmili, B. Fekkak, and S. Harrouni, “New optimized sizing based on the techno-economic analysis of PV system with hybrid storage H2-battery,” presented at the 2021 9th Int. Renew. Sustain. Energy Conf., Morocco, 2021. [Google Scholar]
88. R. G. de Oliveira, S. B. Leal, F. Pradelle, E. S. Torres, and C. L. S. V. Coutinho, “Numerical simulation of an on-grid natural gas PEMFC—Solar photovoltaic micro CHP unit: Analysis of the energy, economic and environmental impacts for residential and industrial applications,” Technol. Econ. Smart Grids Sustain. Energy, vol. 7, 2022, Art. no. 5. doi: 10.1007/s40866-022-00124-3. [Google Scholar] [CrossRef]
89. R. Zahedi and M. Moeini-Aghtaie, “Operational strategy optimization of a hybrid green power system based on fuzzy logic controller with considering for optimal sizing and analysis of different priorities for energy storage,” Sustain. Energy, Grids Netw., vol. 32, 2022. doi: 10.1016/j.segan.2022.100809. [Google Scholar] [CrossRef]
90. T. H. T. Nguyen, T. Nakayama, and M. Ishida, “Optimal capacity design of battery and hydrogen system for the DC grid with photovoltaic power generation based on the rapid estimation of grid dependency,” Int. J. Electr. Power & Energy Syst., vol. 89, no. 2, pp. 27–39, 2017. doi: 10.1016/j.ijepes.2016.12.012. [Google Scholar] [CrossRef]
91. H. Wang, N. N. B. Mansor, H. B. Mokhlis, and A. Mashhadi, “Optimal size selection of combined diesel generator/fuel cell/photovoltaic system components using a multi-objective strategy and sea lion optimization algorithm,” IET Renew. Power Gen., 2023. doi: 10.1049/rpg2.12795. [Google Scholar] [CrossRef]
92. S. Hussain, S. D. Vahdati, H. Zareipour, D. Layzell, and A. Khan, “Optimal sizing of a stand-alone renewable-powered hydrogen fueling station,” presented at the 2022 IEEE Int. Aut. Meet. Power, Electron., Comput., Ixtapa, Mexico, 2022. [Google Scholar]
93. S. H. Mehdi, A. Hasankhani, M. Shafie-khah, M. Lotfi, and J. P. S. Catalão, “Optimal sizing of renewable energy systems in a Microgrid considering electricity market interaction and reliability analysis,” Electr. Power Syst. Res., vol. 203, 2022. doi: 10.1016/j.epsr.2021.107678. [Google Scholar] [CrossRef]
94. A. F. Monforti, C. Amoruso, R. R. Villafáfila, L. D. Zotto, E. Bocci and G. Comodi, “Power-to-Gas from curtailed RES electricity in Spain: Potential and applications,” presented at the 2020 IEEE Int. Conf. Environ. Electr. Eng. 2020 IEEE Indust. Commer. Power Syst. Eur., Madrid, Spain, 2020. [Google Scholar]
95. A. Bouabdallah, J. -C. Olivier, S. Bourguet, A. Houari, and M. Machmoum, “Robust sizing of a stand-alone multi-sources power system,” presented at the 2016 IEEE Int. Conf. Indust. Technol., Taipei, Taiwan, 2016. [Google Scholar]
96. W. Seward, L. Chi, M. Qadrdan, A. Allahham, and K. Alawasa, “Sizing, economic, and reliability analysis of photovoltaics and energy storage for an off-grid power system in Jordan,” IET Energy Syst. Integr., vol. 5, no. 4, pp. 393–404, 2023. doi: 10.1049/esi2.12108. [Google Scholar] [CrossRef]
97. S. Saib, A. Gherbi, A. Kaabeche, and R. Bayindir, “Techno-economic optimization of a grid-connected hybrid energy system considering voltage fluctuation,” J. Electr. Eng. Technol., vol. 13, no. 2, pp. 659–668, 2018. doi: 10.5370/JEET.2018.13.2.659. [Google Scholar] [CrossRef]
98. S. You, J. Hu, Y. Zong, and J. Lin, “Value assessment of hydrogen-based electrical energy storage in view of electricity spot market,” J. Mod. Power Syst. Clean Energy, vol. 4, no. 4, pp. 626–635, 2016. doi: 10.1007/s40565-016-0246-z. [Google Scholar] [CrossRef]
Cite This Article
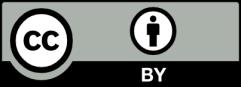
This work is licensed under a Creative Commons Attribution 4.0 International License , which permits unrestricted use, distribution, and reproduction in any medium, provided the original work is properly cited.