Open Access
ARTICLE
Method for Estimating the State of Health of Lithium-ion Batteries Based on Differential Thermal Voltammetry and Sparrow Search Algorithm-Elman Neural Network
School of Electrical and Electronic Engineering, Hubei University of Technology, Wuhan, 430068, China
* Corresponding Author: Daoyu Zhang. Email:
(This article belongs to the Special Issue: Advanced Modelling, Operation, Management and Diagnosis of Lithium Batteries)
Energy Engineering 2025, 122(1), 203-220. https://doi.org/10.32604/ee.2024.056244
Received 17 July 2024; Accepted 25 October 2024; Issue published 27 December 2024
Abstract
Precisely estimating the state of health (SOH) of lithium-ion batteries is essential for battery management systems (BMS), as it plays a key role in ensuring the safe and reliable operation of battery systems. However, current SOH estimation methods often overlook the valuable temperature information that can effectively characterize battery aging during capacity degradation. Additionally, the Elman neural network, which is commonly employed for SOH estimation, exhibits several drawbacks, including slow training speed, a tendency to become trapped in local minima, and the initialization of weights and thresholds using pseudo-random numbers, leading to unstable model performance. To address these issues, this study addresses the challenge of precise and effective SOH detection by proposing a method for estimating the SOH of lithium-ion batteries based on differential thermal voltammetry (DTV) and an SSA-Elman neural network. Firstly, two health features (HFs) considering temperature factors and battery voltage are extracted from the differential thermal voltammetry curves and incremental capacity curves. Next, the Sparrow Search Algorithm (SSA) is employed to optimize the initial weights and thresholds of the Elman neural network, forming the SSA-Elman neural network model. To validate the performance, various neural networks, including the proposed SSA-Elman network, are tested using the Oxford battery aging dataset. The experimental results demonstrate that the method developed in this study achieves superior accuracy and robustness, with a mean absolute error (MAE) of less than 0.9% and a root mean square error (RMSE) below 1.4%.Keywords
Cite This Article
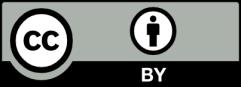
This work is licensed under a Creative Commons Attribution 4.0 International License , which permits unrestricted use, distribution, and reproduction in any medium, provided the original work is properly cited.