Open Access
ARTICLE
Coordinated Control Strategy of New Energy Power Generation System with Hybrid Energy Storage Unit
1 School of Mechano-Electronic Engineering, Xidian University, Xi’an, 710071, China
2 Electric Power Control Center, State Grid Gansu Electric Power Company, Lanzhou, 730030, China
3 Electric Power Control Center, State Grid Gansu Electric Power Company Zhangye Power Supply Branch, Zhangye, 734000, China
* Corresponding Author: Yun Zhang. Email:
Energy Engineering 2025, 122(1), 167-184. https://doi.org/10.32604/ee.2024.056190
Received 16 July 2024; Accepted 29 October 2024; Issue published 27 December 2024
Abstract
The new energy power generation is becoming increasingly important in the power system. Such as photovoltaic power generation has become a research hotspot, however, due to the characteristics of light radiation changes, photovoltaic power generation is unstable and random, resulting in a low utilization rate and directly affecting the stability of the power grid. To solve this problem, this paper proposes a coordinated control strategy for a new energy power generation system with a hybrid energy storage unit based on the lithium iron phosphate-supercapacitor hybrid energy storage unit. Firstly, the variational mode decomposition algorithm is used to separate the high and low frequencies of the power signal, which is conducive to the rapid and accurate suppression of the power fluctuation of the energy storage system. Secondly, the fuzzy control algorithm is introduced to balance the power between energy storage. In this paper, the actual data is used for simulation, and the simulation results show that the strategy realizes the effective suppression of the bus voltage fluctuation and the accurate control of the internal state of the energy storage unit, effectively avoiding problems such as overshoot and over-discharge, and can significantly improve the stability of the photovoltaic power generation system and the stability of the Direct Current bus. It is of great significance to promote the development of collaborative control technology for photovoltaic hybrid energy storage units.Keywords
Nomenclature
DC | Direct Current |
PV | Photovoltaic System |
ESU | Energy Storage Unit |
HESU | Hybrid Energy Storage Unit |
ESS | Energy Storage System |
HESS | Hybrid Energy Storage System |
WOA | Whale Optimization Algorithm |
MPPT | Maximum Power Point Tracking |
LIPB | Lithium Iron Phosphate Battery |
SC | Supercapacitor |
Ppv | Output power of the PV system |
Pref | Expected power of the system |
PHess | Energy storage system power |
Pbat(t) | Power instructions of LIP |
Psc(t) | Power instructions of SC |
PID | Proportional Integral Derivative |
FFT | Fast Fourier Transformation |
The global economy is in a stage of rapid development, and the process of industrialization is constantly advancing, which leads to the continuous increase of electricity demand in the residential and industrial fields of human society [1]. At the same time, traditional energy resources are facing the threat of depletion, so new energy power generation has received extensive attention and rapid development. However, due to environmental constraints, the generated power of new energy power generation is not smooth. For example, photovoltaic (PV) power generation has problems such as large fluctuations and weak regulation and control capabilities, resulting in unstable power generation [2]. If directly connected to the grid, it will have a significant impact on the stability of microgrids and the quality of electrical energy. To improve the efficiency and stability of power generation, the emergence of Energy Storage Units (ESUs) has changed this situation [3]. By using the difference between the expected power and the photovoltaic output power as input for the ESU, compensation for photovoltaic power fluctuations is achieved, thereby smoothing the fluctuations in photovoltaic power output. The role of ESUs in photovoltaic power generation is becoming increasingly important. Therefore, many scholars both domestically and internationally have conducted extensive research on the application of energy storage systems in the field of photovoltaic technology [4].
Based on the literature review, it can be concluded that the ESU has been widely used in PV power generation, and many researchers have studied and continuously improved it. Vann et al. [5] proposed an energy storage optimization technology for solar photovoltaics to achieve PV penetration into the grating base during the heating process of the power generator. Yuan et al. [6] proposed the use of electricity-ammonia mixed combustion energy storage technology in thermal power units in PV systems. The research results show that electricity-to-ammonia has a good improvement effect on the economy and low-carbon operation of integrated energy systems. Li et al. [7] established a theoretical model of photovoltaic and hydrogen energy storage (HS) systems and proposed a hybrid energy storage system combining HS and battery considering season. Wang et al. [8] incorporated the PV system into the HESS to form a photovoltaic HESS suitable for rail transit networks. Sun et al. [9] designed a PV system based on HS using WOA, considering biological waste and fuel cells. The system takes into account the service life of the project for 20 years. Dong et al. [10] innovatively proposed a hybrid energy storage strategy combining supercapacitors (SCs) and batteries to address the fluctuations in grid-connected photovoltaic power. By precisely managing the energy storage elements, the strategy effectively mitigated the power fluctuations, as thoroughly validated through Simulink simulations.
However, although the above-referenced studies achieved notable results in the improvement of PV systems by improving the ESU from the aspect of energy storage technology, the energy management control between PV power generation system, ESU, and energy storage will also have a huge impact on the output power of the system. Based on this idea, many researchers have carried out research and experiments. Aiming at the problem of response lag of an ESS when the output power of a PV system fluctuates. Si et al. [11] proposed a HESS control strategy combined with ultra-short-term PV power prediction. This strategy significantly improves the speed of the energy storage system to stabilize fluctuations and effectively alleviates the lag challenge. In the research of Ye et al. [12], the influence of power fluctuation in photovoltaic grid-connected power generation systems on hybrid energy storage devices is considered, especially the safety problems such as overcharge and over-discharge caused by limited capacity of energy storage units. In addition, Nong et al. [13] focused on the stable operation of the optical storage system, and proposed a control strategy considering the fluctuation of the bus voltage. Compared with the traditional control strategy, this new method significantly reduces the fluctuation of the bus voltage, and makes the output power of the battery more stable, thereby prolonging the service life of the battery and improving the reliability and economy of the entire optical storage system. Tian et al. [14] proposed an improved parallel vertical control strategy based on state of charge (SOC) automatic equalization to solve the problems of slow SOC equalization and large bus voltage fluctuation and offset caused by load and PV power changes in independent DC microgrid. The strategy includes main control and secondary control. Deng et al. [15] proposed a control strategy of variable-speed pumped storage plants (VSPSPs) to increase renewable energy penetration. The strategy improved the new energy utilization by reducing the deviation between the predicted and actual output of new energy.
In addition, the first-order filtering control algorithm is currently one of the most widely applied control strategies, making the selection or adjustment of the filter time constant a critical research focus. References [16–20] combined first-order filtering with ramp rate control to ensure compliance with power fluctuation limits. In Reference [21], variable time constant first-order filters are used to control SCs and batteries, with the filter time constants dynamically adjusted based on real-time charge and discharge power, optimized offline by a particle swarm algorithm. Similarly, the sliding average control method, which operates on principles akin to first-order filtering, relies on the sliding window width as its key parameter. Esmaili et al. [22] combined sliding average control with ramp rate control, introducing two evaluation metrics for power fluctuation mitigation: the full compensation time percentage and the energy compensation percentage. Adaptive filtering algorithms such as Kalman filtering and wavelet filtering are advantageous in managing wind power fluctuations. Li [18] employed Kalman filtering to regulate lithium battery energy storage for smoothing wind power fluctuations, incorporating a state-of-charge feedback control based on complex logic. Additionally, model predictive control (MPC), an online optimization heuristic based on process forecasting, is also utilized for wind power fluctuation mitigation. Hong et al. [23] applied MPC to control lithium batteries for this purpose. The advantages, disadvantages, and application situations of various methods are compared in Table 1.
In summary, the photovoltaic hybrid energy storage system, not only takes into account the stable operation of the PV system, the use of energy storage technology to stabilize power fluctuations and other issues, but also considers the energy storage unit’s frequent charge and discharge, overshoot and over-discharge, and other damage to the energy storage device risks. At present, the control strategies proposed by researchers generally have problems such as incomplete consideration and pertinence.
Aiming to solve the problem, this article proposes a two-level control strategy based on VMD and fuzzy control. The difference between the expected power and the generation power of the PV system is used as the input power of the HESU based on lithium iron phosphate battery-SC. The VMD algorithm is used to divide the frequency of the power, which is smoothed by lithium iron phosphate battery (LIPB) and SC, respectively. After the fuzzy control algorithm, the power balance between the energy storages is carried out to control the energy storage SOC, so that the bus power is smoothed while the internal state of the energy storage unit such as the state of charge is considered to avoid overshoot and over-discharge.
This paper puts forward several main contributions and innovation points:
1. The structure of a parallel HESU is designed, and the bidirectional DC converter of the ESS in PV power generation is deeply studied, and a double closed-loop control strategy is proposed to accurately control the bidirectional DC converter.
2. Aiming at the problem of power distribution control between energy storage units, the VMD algorithm is innovatively applied to the power decomposition of HESU, which improves the frequency separation accuracy of the system and realizes the accurate power distribution and stabilization between LIPB and SC.
3. Based on the MATLAB/Simulink platform, the application effect of the VMD algorithm in PV HESS is verified by using the actual data of a station, which realizes the stabilization of DC bus power, significantly improves the power stability of the system, and provides additional evidence for the effectiveness of the control strategy presented in this paper.
This chapter mainly elaborates on the research background of cooperative control of photovoltaic HESS, introduces the research status of HESS and its control strategy, and leads to the research direction of this paper. Section 2 introduces the topology of the photovoltaic HESS and the overall control strategy. In Section 3, the variational problem in the hybrid system is discussed, and the VMD algorithm is used to solve the problem. At the same time, the effectiveness of the VMD algorithm is verified by the data of an actual station. In Section 4, through the system collaborative control simulation based on VMD algorithm, the simulation results are analyzed in depth, and the corresponding conclusions are finally drawn. Section 5 provides a summary of the control strategy introduced in this paper and the conclusions drawn from the simulation experiments.
2 System Structure and Control Strategy
A typical photovoltaic hybrid energy storage unit consists of a PV array, a LIPB-SC hybrid energy storage unit, and a grid-connected inverter system [24], The structure of the system is shown in Fig. 1.
Figure 1: System structure diagram
In Fig. 1, the PV system initially employs an MPPT algorithm to ensure optimal grid output, maximizing solar energy utilization and minimizing waste. It then calculates the difference between the bus power demand and the actual PV generation, which serves as the output power for the HESU. Utilizing a precise power allocation algorithm, the system intelligently controls the LIPB and SC to flexibly adjust the HESU’s output. This design aims to quickly mitigate power fluctuations from sudden changes in generation or load, ensuring stable and efficient power system operation [25].
Under the topology that is shown in Fig. 1, if there is no ESU, due to the power generation characteristics of PV, the grid voltage and power are bound to fluctuate violently, therefore, the power quality of the ESU grid plays a decisive role, and the core of the HESU lies in the power control algorithm. At present, the simple mechanical strategy of peak shaving and valley filling [26]. Compared with PV power generation in a complex environment, both accuracy and response speed can no longer be satisfied. The control strategy of the HESU proposed in this paper adopts a two-level control strategy, and the control structure is shown in Fig. 2, wherein, Ppv is the generation power of the PV system, Pref is the expected power of the system, PHess is the fluctuating power to be stabilized, that is, the overall power instruction of the fusion ESS.
Figure 2: The overall control strategy of the system
The control policy abandons the traditional first-order low-pass filter, using a more advanced variational mode decomposition (VMD) algorithm, The power to be flattened is decomposed into high-frequency and low-frequency signals, according to the different energy storage characteristics of LIPB and SC devices. LIPBs are energy-based devices with high energy storage efficiency but low response speed to mutations, while SC are power-based devices with high power density and fast response speed. Therefore, the low-frequency signal is sent to the LIPB. The high-frequency signal is sent to the SC for stabilization. secondly, to extend the cycle life of the system, in phosphoric acid Iron lithium with super. The fuzzy control algorithm is introduced between the capacitors to correct the power instructions. Avoid irreversible damage to the energy storage system when the system is in extreme conditions [27].
The LIPB is connected in parallel with the SC, and the control of its Buck-Boost converter adopts a double closed-loop PID control strategy, as Fig. 3 shows.
Figure 3: Dual-closed-loop PID control strategy based on VMD decomposition
The reference voltage is compared with the actual voltage and then adjusted by PID, and then the reference current of iron phosphate and SC is obtained by decomposition of VMD, and then the PID is used to adjust after comparing with the actual current, and finally, the PWM is generated to generate the control signal, and the converter of the hybrid energy storage unit is controlled to realize the power distribution control [28].
Set the discharge depth of LIPB and SC to 10%–90%, set up a fuzzy controller, and start the fuzzy controller to adjust according to the current SOC status of each energy storage unit and the next energy storage output power, and output the adjustment parameters k(t).
When the control instructions are corrected by using the fuzzy control algorithm, and k(t)>0, it is stated that a part of the power instructions of the SC needs to be transferred to LIPB. The power instructions of LIPB and SC are respectively Pbat(t),Psc(t):
Psc(t)=k(t)×P∗sc(t)(1)
Pbat(t)=P∗bat(t)+[1−k(t)]×P∗sc(t)(2)
When k(t)<0, it is stated that a part of the power command of LIPB is transferred to the SC, and the LIPB is corrected. The power instructions of LIPB and SC are respectively Pbat(t), Psc(t) [29]:
Pbat(t)=k(t)×P∗bat(t)(3)
Psc(t)=P∗sc(t)+[1−k(t)]×P∗bat(t)(4)
3 Variational Modal Decomposition
VMD is an adaptive and completely non-recursive approach to modal variation and signal processing [30]. Its advantage lies in the ability to adaptively determine the number of modal decompositions based on the actual situation. The fundamental concept of VMD is to establish and solve variational problems.
3.1 Construct the Variational Problem of ESS
Suppose that in the PV ESS, the energy storage power signal PHess(t) is decomposed into K variables:
PHess(t)=∑Ki=1pi(t)(5)
where PHess(t) is the original energy storage power; pi(t) is the component.
The VMD constrained variational model is as follows [31]:
min{pi,ωi}{∑i‖∂t[(δ(t)+jπt)×pi(t)]e−jωit‖22}(6)
s.t.∑ipi(t)=PHess(t)(7)
where pi={p1,p2,…,pi} is the function of each modality; ωi={ω1,ω2,…,ωi} is the center frequency of each modality is described.
3.2 Variational Modal Algorithm
The VMD method simultaneously calculates all modal waveforms and their center frequencies. It is a variational problem that searches for pi(t) and ωi(t) minimization constraints. In order to obtain pi(t) and ωi(t), the IMF in the frequency domain is calculated, and X(ω)=DFT{X(t)} is reconstructed in the form of pi(ω)=DFT{pi(t)}. In order to eliminate the edge effect, the signal is extended by half the length of the mirror signal on both sides. The optimal solution of augmented Lagrangian is introduced in the optimization process [32]:
{L(pi(t),wi,λ(t))=(i)+(ii)+(iii)(i)=α∑Ki=1‖ddt[(δ(t)+jπt)×pi(t)]e−j2πwit‖22(ii)=x(t)−∑Ki=1pi(t)22(iii)=λ(t),x(t)−∑Ki=1pi(t)(8)
where the inner product ⟨p(t),q(t)⟩=∫+∞−∞p(t)q(t)dt; 2 − norm p(t)22=p(t),p(t). (i) and (ii) terms enforce constraints x(t)=∑Ki=1pi(t), by applying a quadratic penalty in combination with a Lagrange multiplier. This Lagrangian multiplier has a Fourier transform Λ(ω).
The steps of the VMD decomposition algorithm are as follows:
1) Constructing the variational problem
The variational problem of hybrid energy storage in this paper is shown in Eqs. (6) and (7).
2) Travers the K modalities of the signal
(a) Calculate the waveform for each modality
Pn+1i(ω)=PHess(ω)−∑m<iPn+1m(ω)−∑m>iPnm(ω)+Λn21+2α{2π(ω−ωni)}2(9)
where pi(ω) is the Fourier transform of the ith mode computed in the (n+1) iterations; Λ is Λ(ω), the Fourier transform that represents the Lagrange multiplier.
(b) Calculate the center frequency of the first modality i
ωn+1i=∫∞0|Pn+1i(ω)|2ωdω∫∞0|Pn+1I(ω)|2dω≈∑ω|Pn+1i(ω)|2∑|Pn+1i(ω)|2(10)
3) Use Eq. (10) to updated Lagrange multiplier
Λn+1(ω)=Λn(ω)+τ(PHess(ω)−∑iPn+1i(ω))(11)
where τ is the Lagrange multiplier update rate.
4) Determine the stop condition
Judge pi(ω): If the stop condition is not met, repeat Steps 2) and 3); If the stop condition is met, the algorithm is terminated and pi(ω) is output.
The k modes after VMD decomposition can be directly added to obtain an approximate signal of the original signal. Combining the contents of Sections 3.1 and 3.2, the complete process of using VMD to decompose a signal is shown in Fig. 4.
Figure 4: VMD signal flow chart
4.1 VMD Is Applied to Hybrid Energy Storage Units
In this paper, the actual output power data of a 200 MW photovoltaic farm on a certain day is used, and the sampling time is 15 min, as Fig. 5 shows. Directly call the FFT function in the MATLAB toolbox to perform a fast Fourier transform on the data to obtain the amplitude-frequency characteristic curve, such as Fig. 6 shows.
Figure 5: Actual output power
Figure 6: Amplitude-frequency characteristic curve
According to Fig. 5, the analysis shows that from the perspective of the time domain, the energy of photovoltaic field output power is mainly concentrated in the daytime period (7:00–17:00), which is consistent with the light characteristics. As shown in Fig. 6, the analysis shows that from the perspective of the frequency domain, the energy of the output power of the photovoltaic electric field is mainly concentrated in the low-frequency part (from the amplification part, the low-frequency part is mainly 0~4 Hz), and the high-frequency part has low energy. This is consistent with the light variation characteristic, where the light amplitude is small for high-frequency variations and large for low-frequency variations. The LIPB is an energy-based energy storage unit, which is suitable for absorbing and releasing high-density energy, while the SC is suitable for undertaking high-power and low-energy work, so the low-frequency power signal is taken as the expected power value of LIPB, and the high-frequency power signal is smoothed by the SC. Therefore, in this paper, the VMD method is used to decompose the power to be leveled into high-frequency signals and low-frequency signals.
In this paper, the expected output power of the photovoltaic farm is set to 120 MW, and calculate energy storage power by Eq. (12):
PHess=Pref−Ppv(12)
where PHess is the energy storage power, Pref is the expected power, and Ppv is the photovoltaic output power.
The energy storage power curve is shown in Fig. 6.
In Fig. 7, the energy storage power curve greater than zero represents the absorbed power of the energy storage, and less than the zero part represents the power to be output by the energy storage.
Figure 7: Energy storage power curve
After the energy storage power is obtained, the VMD decomposition algorithm is used to decompose the energy storage power at high and low frequencies. The decompose results for VMD are shown in Fig. 8.
Figure 8: VMD decomposition results
According to Fig. 8, it can be seen that the VMD decomposition algorithm can decompose the signal well for high and low frequency, and the low-frequency signal shows an overall energy change trend while the high-frequency energy is not high, but the change frequency is fast, which is in line with the practical application. In Fig. 8c, it can be seen that VMD decomposition cannot ensure that the original signal can be fully distributed to the high-frequency signal or low-frequency signal and there will be residuals, and this part also represents the relatively extremely high-frequency signal, which is the part of the output power that cannot be flattened and cannot be used by the energy storage system.
To further analyze the decomposition effect, and whether the low frequency can represent the overall change trend of energy, the low-frequency signal is compared with the original signal, as Fig. 9 shows.
Figure 9: Low frequency vs. raw signal
In Fig. 9, it can be seen that the low-frequency signal is almost the outline of the original signal, and there is no burr signal, which makes the LIPB not need to be charged and discharged frequently, but stable output, dominate the energy change of the system, and continue to supply/absorb energy, which is in line with the actual application, which also shows that the decomposition algorithm is effective.
To observe the difference between high and low frequency signals, the high and low frequency signals are shown in Fig. 10.
Figure 10: Comparison of high-frequency and low-frequency
As shown in Fig. 10, the high and low frequency signals have huge differences in amplitude and frequency, which are in line with the goals and expectations of LIPB energy supply and fast response of SC, and the decomposition effect of VMD decomposition algorithm is a good, which can effectively distinguish high and low frequency signals, and can be applied to actual systems, which has certain engineering significance.
4.2 Operating Control Simulation Experiments
To make the simulation experiment more real and reliable, this paper uses the simulation method combining actual data analysis and simulation experiments to experiment, that is, this paper uses the actual light of a photovoltaic farm on a certain day, such as Fig. 11 as shown, a simulation test model is built, and the parameters of the simulated photovoltaic array are used as follows Table 2 to perform a simulation experiment. The system simulation model is shown in Fig. 12.
Figure 11: Actual lighting curve
Figure 12: System simulation model
The photovoltaic output power and the DC bus power are obtained through simulation, are shown in Fig. 13.
Figure 13: Comparison chart of PV power generation and DC bus power
The simulation results show that due to the drastic change of illumination, the output power of the PV system will fluctuate greatly, and it cannot be directly connected to the power grid, after the hybrid energy storage unit is stabilized, it can be seen that the power on the DC bus is stable as a whole except for the sudden change in some places, and the expected power is maintained for output. The effectiveness of the proposed operation control strategy is verified.
To verify the superiority of the control strategy proposed in this paper compared with other methods, the proposed method was compared with other methods, and the comparison results are shown in Table 3.
As shown in Table 3, it is evident that the traditional first-order low-pass filter results in significant bus power fluctuations during coordinated operation, with almost no stabilization, reaching a fluctuation range as high as 68.8%, which would still exert considerable impact on the grid. Although the EMD algorithm significantly reduces the fluctuation range, it remains suboptimal. In contrast, the VMD algorithm proposed in this paper achieves a more stable DC bus during the coordinated operation of the hybrid energy storage system, with a significantly reduced fluctuation range, maintaining it below 3%. Therefore, the control strategy proposed in this paper demonstrates superior performance compared to other control methods, offering better control effectiveness and significant practical engineering implications.
In this paper, a coordinated control strategy of a new energy power generation system with a HESU is proposed, to solve the problem that the randomness of the PV power generation system leads to large fluctuation of PV output power so that PV output power cannot be directly connected to the power grid. The system model was developed and simulated using MATLAB/Simulink. Based on the simulation results, the following conclusions were drawn:
1. The parallel energy storage system structure adopted in this study meets the operational requirements, and the dual-loop control strategy, after parameter tuning, enables the bidirectional DC-DC converter to be controlled quickly and effectively.
2. The VMD algorithm effectively and accurately decomposes the power signal, achieving the separation of high- and low-frequency signals. The low-frequency signal, handled by the LIPB, is maintained at a relatively low power frequency, while the high-frequency component is effectively smoothed by the SC, achieving power distribution control among the energy storage units.
3. The system’s coordinated control simulation results, combined with actual field data, indicate that photovoltaic power fluctuations can be smoothed. With the integration of the hybrid energy storage system, the DC bus power remains relatively stable, validating the effectiveness of the control strategy proposed in this paper.
Through in-depth research and simulation experiments, certain achievements have been made in this study. However, some limitations remain:
1. During the decomposition process using the VMD algorithm, residuals may occur, particularly in the very high-frequency components of the power signal. The exclusion of these residuals can lead to minor fluctuations in the DC bus. Future research should focus on compensating for this component to mitigate its impact.
2. The control strategy’s effectiveness was verified using data from a single station, which may not fully eliminate the possibility of random occurrences. In future research, the scope of data collection will be expanded to include multiple locations to comprehensively validate the effectiveness of the control strategy.
In summary, in this paper, the efficacy of the proposed control strategy is validated through a comprehensive approach combining actual data analysis and simulation experiments. This validation methodology holds practical significance for the relevant engineering projects.
Acknowledgement: Not applicable.
Funding Statement: This work was supported by the State Grid Corporation of China Science and Technology Project, grant number 52270723000900K.
Author Contributions: The authors confirm contribution to the paper as follows: study conception and design: Yun Zhang, Biao Tian; data collection: Zifen Han, Ning Chen, Yi Fan; analysis and interpretation of results: Yun Zhang, Zifen Han, Biao Tian; draft manuscript preparation: Yun Zhang, Biao Tian. All authors reviewed the results and approved the final version of the manuscript.
Availability of Data and Materials: Data will be made available on request.
Ethics Approval: Not applicable.
Conflicts of Interest: The authors declare that they have no conflicts of interest to report regarding the present study.
References
1. R. Gugulothu, B. Nagu, D. Pullaguram, and B. C. Babu, “Optimal coordinated energy management strategy for standalone solar photovoltaic system with hybrid energy storage,” J. Energy Storage, vol. 67, no. 6, Sep. 2023, Art. no. 107628. doi: 10.1016/j.est.2023.107628. [Google Scholar] [CrossRef]
2. L. Ye, Y. W. Zhong, and C. W. Tang, “Sizing optimization of a photovoltaic hybrid energy storage system based on long time-series simulation considering battery life,” Appl. Sci., vol. 13, no. 15, Jul. 2023, Art. no. 8693. doi: 10.3390/app13158693. [Google Scholar] [CrossRef]
3. G. Boroumandfar, A. Khajehzadeh, M. Eslami, and R. B. Y. Syah, “Information gap decision theory with risk aversion strategy for robust planning of hybrid photovoltaic/wind/battery storage system in distribution networks considering uncertainty,” Energy, vol. 278, no. 4, Sept. 2023, Art. no. 127778. doi: 10.1016/j.energy.2023.127778. [Google Scholar] [CrossRef]
4. K. C. Liu, L. Chen, D. Y. Xiao, and L. P. Liu, “Analysis and modeling of time output characteristics for distributed photovoltaic and energy storage,” Energy Eng.: J. Assoc. Energy Eng., vol. 124, no. 4, pp. 933–949, Mar. 2024. doi: 10.32604/ee.2023.043658. [Google Scholar] [CrossRef]
5. S. Vann, H. Zhu, C. Chen, and D. Zhang, “Solar photovoltaic penetration into the grid based on energy storage optimization technology,” in Proc. 2023 Int. Conf. Wirel. Power Transf. (ICWPT2023), Weihai, China, Oct. 2024, pp. 554–562. [Google Scholar]
6. W. T. Yuan, L. Chen, C. B. Wang, and Z. R. Wang, “Bi-level optimal scheduling of power-to-ammonia coupling wind-photovoltaic-thermal integrated energy system based on ammonia energy storage technology,” (in Chinese), Proc. CSEE, vol. 43, no. 18, pp. 6992–7002, Sep. 2023. doi: 10.13334/j.0258-8013.pcsee.223152. [Google Scholar] [CrossRef]
7. Y. Li, J. Zhao, X. Yang, and Y. Y. Wang, “Research on scheduling strategy of flexible interconnection distribution network considering distributed photovoltaic and hydrogen energy storage,” Energy Eng., vol. 121, no. 5, pp. 1263–1289, Apr. 2024. doi: 10.32604/ee.2024.046784. [Google Scholar] [CrossRef]
8. X. Wang, X. Wang, and B. Qin, “Improved multi-objective differential evolution algorithm and its application in the capacity configuration of urban rail photovoltaic hybrid energy storage systems,” J. Energy Storage, vol. 98, Sep. 2024, Art. no. 113115. doi: 10.1016/j.est.2024.113155. [Google Scholar] [CrossRef]
9. H. Y. Sun, A. G. Ebadi, M. Toughani, S. A. Nowdeh, A. Naderipour and A. Abdullah, “Designing framework of hybrid photovoltaic-biowaste energy system with hydrogen storage considering economic and technical indices using whale optimization algorithm,” Energy, vol. 238, no. 4, Jan. 2022, Art. no. 121555. doi: 10.1016/j.energy.2021.121555. [Google Scholar] [CrossRef]
10. X. Dong and H. L. Cen, “Hybrid energy storage strategy for suppressing power grid fluctuation of photovoltaic power generation system,” in 2020 IEEE 9th Int. Power Electron. Motion Control Conf. (IPEMC2020-ECCE Asia), Nanjing, China, 2020, pp. 3196–3203. [Google Scholar]
11. M. J. Si, K. Y. Zhao, K. J. Chen, and G. H. Lin, “Hybrid energy storage control strategy based on ultra-short-term photovoltaic power prediction,” (in Chinese), J. Hunan Univ. Eng. (Nat. Sci. Ed.), vol. 33, no. 2, pp. 22–29, Jun. 2023. doi: 10.15987/j.cnki.hgbjbz.2023.02.009. [Google Scholar] [CrossRef]
12. Y. Ye and L. J. Zhu, “Hybrid energy storage power distribution control strategy for photovoltaic power generation system,” (in Chinese), Electr. Technol., no. 20, pp. 45–48+52, Oct. 2023. doi: 10.19768/j.cnki.dgjs.2023.20.014. [Google Scholar] [CrossRef]
13. B. X. Nong, C. Yang, Q. Yang, and Y. Xiao, “Photovoltaic hybrid energy storage control strategy considering bus voltage fluctuation,” (in Chinese), Comput. Simul., vol. 41, no. 1, pp. 91–96, Jan. 2024. [Google Scholar]
14. J. Tian, S. Mo, F. Zhao, and X. Q. Chen, “Automatic SOC equalization strategy of energy storage units with DC microgrid bus voltage support,” Energy Eng.: J. Assoc. Energy Eng., vol. 121, no. 2, pp. 439–459, Jan. 2024. doi: 10.32604/ee.2023.029956. [Google Scholar] [CrossRef]
15. Y. W. Deng et al., “Dynamic analysis of variable-speed pumped storage plants for mitigating effects of excess wind power generation,” Int. J. Electr. Power Energy Syst., vol. 135, Feb. 2022, Art. no. 107453. doi: 10.1016/j.ijepes.2021.107453. [Google Scholar] [CrossRef]
16. T. Tanabe, T. Sato, R. Tanikawa, I. Aoki, T. Funabashi and R. Yokoyama, “Generation scheduling for wind power generation by storage battery system and meteorological forecast,” in 2008 IEEE Power Energy Soc. Gen. Meet.-Convers. Deliv. Electr. Energy 21st Century, Pittsburgh, PA, USA, 2008, pp. 1–7. [Google Scholar]
17. X. J. Li, D. Hui, L. Wu, and X. K. Lai, “Control strategy of battery state of charge for wind/battery hybrid power system,” in 2010 IEEE Int. Symp. Ind. Electron., Bari, Italy, 2010, pp. 2723–2726. [Google Scholar]
18. X. Li, “Fuzzy adaptive Kalman filter for wind power output smoothing with battery energy storage system,” IET Renew. Power Gener., vol. 6, no. 5, pp. 340–347, Sep. 2012. doi: 10.1049/iet-rpg.2011.0177. [Google Scholar] [CrossRef]
19. Q. Jiang and H. Wang, “Two-time-scale coordination control for a battery energy storage system to mitigate wind power fluctuations,” IEEE Trans. Energy Convers., vol. 28, no. 1, pp. 52–61, Mar. 2013. doi: 10.1109/TEC.2012.2226463. [Google Scholar] [CrossRef]
20. Q. Y. Jiang, Y. Z. Gong, and H. J. Wang, “A battery energy storage system dual-layer control strategy for mitigating wind farm fluctuations,” IEEE Trans. Power Syst., vol. 28, no. 3, pp. 3263–3273, Aug. 2013. doi: 10.1109/TPWRS.2013.2244925. [Google Scholar] [CrossRef]
21. X. Liu and Q. Y. Jiang, “An optimal coordination control of hybrid wind/photovoltaic/energy storage system,” Autom. Electr. Power Syst., vol. 36, no. 14, pp. 95–100, Jul. 2012. doi: 10.3969/j.issn.1000-1026.2012.14.019. [Google Scholar] [CrossRef]
22. A. Esmaili, B. Novakovic, A. Nasiri, and O. Abdel-Baqi, “A hybrid system of Li-ion capacitors and flow battery for dynamic wind energy support,” IEEE Trans. Ind. Appl., vol. 49, no. 4, pp. 1649–1657, Jul.–Aug. 2013. doi: 10.1109/TIA.2013.2255112. [Google Scholar] [CrossRef]
23. H. S. Hong, Q. Y. Jiang, and Y. Yan, “An optimization control method of battery energy storage system with wind power fluctuations smoothed in real time,” Autom. Electr. Power Syst., vol. 37, no. 1, pp. 103–109, Jan. 2013. doi: 10.7500/AEPS201206177. [Google Scholar] [CrossRef]
24. J. W. Gao, Y. P. Wang, F. J. Guo, and J. Y. Chen, “A two-stage decision framework for GIS-based site selection of wind-photovoltaic-hybrid energy storage project using LSGDM method,” Renew. Energy, vol. 222, no. 60, Feb. 2024, Art. no. 119912. doi: 10.1016/j.renene.2023.119912. [Google Scholar] [CrossRef]
25. C. Mukul, H. Ikhlaq, and A. Aijaz, “Seamless control of grid-tied PV-hybrid energy storage system,” Int. J. Emerg. Electric Power Syst., vol. 22, no. 5, pp. 569–582, Jun. 2021. doi: 10.1515/ijeeps-2021-0090. [Google Scholar] [CrossRef]
26. M. Chankaya, A. Ahmad, and I. Hussain, “Path-finder optimization based control of grid-tied PV hybrid energy storage system,” IETE J. Res., vol. 69, no. 8, pp. 5289–5306, Aug. 2023. doi: 10.1080/03772063.2021.1963335. [Google Scholar] [CrossRef]
27. O. P. Jaga and S. G. Choudhuri, “Seamless transition between grid-connected and islanded operation modes for hybrid PV-BESS combination used in single-phase, critical load applications,” in 2021 Int. Conf. Sustain. Energy Future Electr. Transport. (SEFET), Hyderabad, India, 2021, pp. 1–6. [Google Scholar]
28. C. J. Jena and P. K. Ray, “Power management in three-phase grid-integrated PV system with hybrid energy storage system,” Energies, vol. 16, no. 4, 2023, Art. no. 2030. doi: 10.3390/en16042030. [Google Scholar] [CrossRef]
29. I. Bhogaraju, J. N. Forestieri, M. Malisoff, and M. Farasat, “Delay-compensating stabilizing feedback controller for a grid-connected PV/hybrid energy storage system,” IEEE Trans. Control Syst. Technol., vol. 31, no. 4, pp. 1875–1883, Jul. 2023. doi: 10.1109/TCST.2022.3227501. [Google Scholar] [CrossRef]
30. W. Qiu et al., “Cyber-attack identification of synchrophasor data via VMD and multifusion SVM,” IEEE Trans. Ind. Appl., vol. 58, no. 2, pp. 1456–1465, Mar.–Apr. 2022. doi: 10.1109/TIA.2022.3144381. [Google Scholar] [CrossRef]
31. Y. Zhang, L. Xiao, H. Zhou, and G. H. Zhao, “Control strategy of wind power smooth grid connection based on adaptive VMD and hybrid energy storage,” J. Renew. Sustain. Energy, vol. 14, no. 2, Mar. 2022, Art. no. 023306. doi: 10.1063/5.0077754. [Google Scholar] [CrossRef]
32. X. Zhang, B. Wang, D. Gamage, and A. Ukil, “Model predictive and iterative learning control based hybrid control method for hybrid energy storage system,” IEEE Trans. Sustain. Energy, vol. 12, no. 4, pp. 2146–2158, Oct. 2021. doi: 10.1109/TSTE.2021.3083902. [Google Scholar] [CrossRef]
Cite This Article
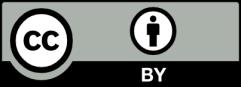
This work is licensed under a Creative Commons Attribution 4.0 International License , which permits unrestricted use, distribution, and reproduction in any medium, provided the original work is properly cited.