Open Access
ARTICLE
Optimizing Biodiesel Production from Karanja and Algae Oil with Nano Catalyst: RSM and ANN Approach
1 Energy Centre, Maulana Azad National Institute of Technology, Bhopal, 462003, India
2 Department of Mechanical Engineering, Maulana Azad National Institute of Technology, Bhopal, 462003, India
* Corresponding Author: Gaurav Dwivedi. Email:
Energy Engineering 2024, 121(9), 2363-2388. https://doi.org/10.32604/ee.2024.052523
Received 04 April 2024; Accepted 12 July 2024; Issue published 19 August 2024
Abstract
This study delves into biodiesel synthesis from non-edible oils and algae oil sources using Response Surface Methodology (RSM) and an Artificial Neural Network (ANN) model to optimize biodiesel yield. Blend of C. vulgaris and Karanja oils is utilized, aiming to reduce free fatty acid content to 1% through single-step transesterification. Optimization reveals peak biodiesel yield conditions: 1% catalyst quantity, 91.47 min reaction time, 56.86°C reaction temperature, and 8.46:1 methanol to oil molar ratio. The ANN model outperforms RSM in yield prediction accuracy. Environmental impact assessment yields an E-factor of 0.0251 at maximum yield, indicating responsible production with minimal waste. Economic analysis reveals significant cost savings: 30%–50% reduction in raw material costs by using non-edible oils, 10%–15% increase in production efficiency, 20% reduction in catalyst costs, and 15%–20% savings in energy consumption. The optimized process reduces waste disposal costs by 10%–15%, enhancing overall economic viability. Overall, the widespread adoption of biodiesel offers economic, environmental, and social benefits to a diverse range of stakeholders, including farmers, producers, consumers, governments, environmental organizations, and the transportation industry. Collaboration among these stakeholders is essential for realizing the full potential of biodiesel as a sustainable energy solution.Keywords
Nomenclature
AV | Acid value |
ANOVA | Analysis of variance |
BBD | Box-Behnke design |
DOE | Design of experiment |
FAME | Fatty acid methyl esters |
GA | Genetic algorithm |
PSO | Particle swarm optimization |
w/w | Weight/weight |
E-factor | Environmental factor |
N₂ | Nitrogen |
cSt | Centistokes |
V/cm | Voltage per centimeter |
CaO | Calcium Oxide |
rpm | Revolution per minute |
V | Voltage |
LPH | Liter per hour |
cSt/s | Centistokes/second |
Kg/m³ | Kilogram per cubic meter |
MJ/kg | Megajoule per kilogram |
EN | European Norm |
Adj SS | Adjusted Sum of Squares |
ANN | Artificial neural network |
ASTM | American Society for Testing and Materials standards |
CCD | Central composite design |
FFA | Free fatty acid |
PM | Particulate matter |
RSM | Response surface methodology |
Mol/mol and m/m | Mole/mole |
SOx | Sulfur Oxide |
CSIR | Council of Science and Industrial Research |
G/cc | Gram per cubic centimeter |
W | Watt |
NaOH | Sodium Hydroxide |
h | Hour |
wt.% | Weight percentage |
KOH | Potassium Hydroxide |
ASTM | American Society for Testing and Materials |
Mm²/s | Millimeter square per second |
v/v | Volume per volume |
DF | Degree of Freedom |
Adj MS | Adjusted Mean Square |
Energy demand increases GHG emissions, causing climate change and air pollution, which kills 6.5 million annually [1–4]. Shifting to renewables is crucial. Biodiesel, a non-toxic, biodegradable, and renewable fuel, reduces pollutants and emissions, offering a sustainable solution for transportation and agriculture sectors heavily reliant on fossil fuels [5–9].
The production of biodiesel involves alcoholysis of oil with base or acid catalysts [10]. Despite similar heating values, using straight oil as diesel fuel is inadvisable due to higher viscosity, lower volatility, and engine carbon deposition. Biodiesel is classified into 1st to 4th generations based on oil availability and feedstock sourcing [11]. Although many feedstocks have been explored, the high cost and limited availability of edible oils hinder large-scale production, particularly in South Asia, where using edible oils for fuel affects food security. Second-generation feedstocks, which are non-edible, require less farming area, are easier to cultivate, and are cheaper, offering a solution to the limitations of edible oils [12]. Thus, exploring alternative inedible sources and algae oil for biodiesel production is essential [11,13–15].
Karanja, a native Indian and Southeast Asian tree, and Chlorella vulgaris microalgae stand out as promising biodiesel candidates. Karanja, known for its N₂ fixation and abundant oilseeds, has evolved from rural lighting and paint production to a biodiesel staple [16]. Meanwhile, Chlorella vulgaris boasts low FFA content and thrives in various conditions, including marginal lands and coastal areas. Researchers at CSIR-National Institute of Oceanography (Goa) have developed a cleaner, cost-effective method for cultivating this microalgae, using fishmeal facility effluents [17]. However, the minimum biodiesel selling price (MBSP) for microalgae biodiesel is approximately $2.17, needing halving to compete with global petroleum prices at $1.09 per liter. Microalgae concentration proves critical, with a range of 20%–40% (wt.%) lowering MBSP from $3.03 to $1.74 per liter. Integrating microalgae biomass with sugar factory waste offers insights for future research and enhances production’s economic feasibility [18].
While production of biodiesel from single oil feedstocks has advantages, it is not without limitations, including impoverished oxidation resistance and cold flow characteristics. Furthermore, high FFA feedstocks can result in saponification during transesterification. To deal with these challenges, investigators have recommended numerous approaches, incorporating the usage of two or more raw materials for bio propellant, to enhance biodiesel quality, reduce raw material costs, and mitigate availability issues, ultimately lowering production costs. This study primarily focuses on the blending of two naturally compatible oils, Karanja (Pongamia pinnata) and Chlorella vulgaris oil, both exhibiting similar fatty acid composition, FFA content, acid value, and other physicochemical characteristics in their natural state. The commercialization of biodiesel as a fuel has faced certain limitations, such as higher costs and quality standards compared to diesel fuel. Notable research endeavors have addressed these challenges, exemplified by Singh Pali et al. who successfully derived excellent biodiesel from Kusum oil using base-catalyzed transesterification. The optimal conditions for Kusum biodiesel synthesis included a methanol to oil molar ratio of 7.5:1, a reaction temperature of 63.3°C, and a reaction time of 93 min, resulting in biodiesel with a kinetic viscosity of 5.15 cSt, a density of 0.88 g/cc, and a yield of 95% [19]. Karimi et al. achieved production of biofuel from the inedible seed oil of copiously accessible wild Azadirachta at room temperature. The study reported a maximum biodiesel yield of 80% using the catalyst, with process parameters including methyl alcohol to oil molar ratios (spanning from 5:1 to 20:1), a response time of 13 h, and voltage (spanning from 5 to 15 V/cm) as determined by Response Surface Methodology based on Central Composite Design (CCD) [20]. Table 1 in this study outlines the optimization of various feedstocks using various simulation tools, including RSM, Genetic Algorithms (GA), and ANN, among others. It also presents the range of process parameters and optimized values derived from the research of various investigators. In Table 2, we explore the blending of diverse comestible and inedible oils to produce biodiesel from hybrid oil sources. The impending sections of this research endeavour will delve further into the intricacies of optimizing biodiesel production, specifically concentrating on the fusion blend of Chlorella Vulgaris and Karanja oils. This investigation employs sophisticated techniques, such as RSM and ANN modelling, to anticipate and enhance biodiesel output, thereby contributing to the promotion of sustainable energy production and environmental responsibility. The pursuit of efficient biodiesel production from various feedstocks has led to notable contributions in recent research endeavours. Attari et al. focused on Waste Cooking Oil (WCO) and employed Response Surface Methodology (RSM) to optimize the key process parameters. The parameters included reaction time (ranging from 20 to 40 min), ultrasonic power (varying from 150 to 300 W), methanol to oil ratio (ranging from 6:1 to 12:1 m/m), and catalyst loading (varying from 6% to 12% w/w). Their study culminated in the achievement of an impressive biodiesel yield of 99%, realized under the following optimized conditions: a reaction time of 39.84 min, ultrasonic power of 299.66 W, a methanol to oil molar ratio of 8.33 m/m, and a catalyst loading of 6% w/w [21].
Ajala et al. innovated biodiesel production using solid catalysts, with waste lard as the feedstock. They optimized biodiesel yield employing Genetic Algorithm (GA), Particle Swarm Optimization (PSO) Response Surface Methodology (RSM) and Firefly Algorithm (FA). Optimization parameters included catalyst type (Cat900, Cat700, Cat500), response time (1–4 h), operating temperature (50°C–60°C), and methyl alcohol to oil ratio. The study achieved a remarkable 97% biodiesel yield under optimal conditions: Cat500 catalyst at 5% (w/w), 1-h reaction time, 59.97°C operating temperature, and 12:1 methyl alcohol to oil ratio [22]. In another significant study, Singh et al. employed Genetic Algorithm (GA) and Response Surface Methodology (RSM) to maximize biodiesel yield. They utilized supercritical methanol transesterification under ideal conditions: 287°C temperature, 123 bar pressure, and 23-min reaction time. These innovative approaches signify the evolving landscape of biodiesel research, offering considerable potential for enhancing production efficiency and sustainability [23].
Biodiesel production efficiency hinges on various factors like methanol to oil ratio, operating temperature, time, stir speed, and catalyst content. Response Surface Methodology (RSM) offers a comprehensive analysis, considering individual and interaction effects to cut operational costs and maximize yield [34,35]. RSM employs quantitative approaches like Doehlert matrix, Box-Behnken Design (BBD), and Central Composite Design (CCD), each with distinct advantages. Artificial Neural Networks (ANN) also optimize processes, modeling, and prediction, mimicking brain processes [36–38]. Agu et al. integrated ANN and RSM for biodiesel optimization from Anacardium occidentale and AOKO. Comparatively, fossil fuel prices are volatile due to geopolitical events, while biodiesel prices tend to be more stable due to localized feedstock production. Currently, B20 costs $3.83/gallon, petrol $3.06/gallon, diesel $3.94/gallon, B100 biodiesel $4.69/gallon, and natural gas (CNG) $2.95/gallon, indicating biofuels’ potential as future energy alternatives [39].
Biodiesel production enhances energy security by reducing reliance on imported fossil fuels and diversifying energy sources. Fossil fuel dependence exposes economies to supply disruptions, geopolitical risks, and price volatility. Advancements in both biodiesel and fossil fuel industries target efficiency, cost reduction, and environmental impact mitigation, including greenhouse gas emissions and pollution. Innovation in biodiesel production processes and conversion technologies boosts economic competitiveness compared to fossil fuels. Despite upfront investments and feedstock challenges, biodiesel offers price stability, environmental benefits, and potential incentives. Addressing knowledge gaps, this investigation blends high FFA Karanja oil with low FFA Algae oil for biodiesel production, optimizing parameters via Response Surface Methodology and predicting yield with Artificial Neural Networks. These findings can enhance commercial biodiesel production and guide optimal oil blending.
The selection of Karanja and Algae oil for biodiesel production in this experiment is underpinned by their unique characteristics and suitability for the optimization of process parameters. Karanja oil, chosen for its non-edibility, easy availability, lower density, higher cetane number, and superior calorific value, offers a promising source for synthesis of biodiesel. The saturated fatty acid profile of Algae and Karanja oil is enlisted in Table 3. However, it is essential to note that the FFA concentration in Karanja oil is initially measured at 2%, which exceeds the permissible limit when employing a base catalyst. An FFA concentration above 1% can trigger saponification process, leading to the formation of soap during biodiesel production. Consequently, the FFA content in Karanja oil must be reduced to less than 1% to ensure a smooth transesterification process. In contrast, Algae oil presents a more favourable FFA concentration of only 0.54%. This lower FFA concentration positions it as an excellent candidate for blending with Karanja oil to achieve the desired ultimate FFA amount of under 1%. In this experiment, the oils are unified in a proportional volume of 75:25, offering the advantage of maintaining a suitable FFA level for efficient biodiesel production. This innovative blend leverages the strengths of each oil source while mitigating the challenges associated with high FFA content in Karanja oil, ultimately contributing to the optimization of the biodiesel production process. Fig. 1 shows the schematic diagram of biodiesel production methodology.
Figure 1: Schematic diagram of biodiesel production methodology
2.2.1 API (American Petroleum Institute) Gravity
In this study, essential oil characteristics, including specific gravity, API gravity, and viscosity, were determined through established procedures and standardized methods. To calculate the specific gravity of the oil, the weight of the oil was divided by the weight of an equivalent volume of water, employing a specific gravity bottle. The viscosity of the oil was assessed using a viscometer, while the API gravity was calculated using Eq. (1) [40]. These measurements and calculations provide vital insights into the properties of the oils, which are critical for understanding their suitability and performance in the biodiesel production process.
Here
The acid value is an indicator of the FFA content of the oil. The use of a homogeneous alkali catalyst with oil with an acid value greater than 2 results in an unintended saponification reaction. One gram of individual sample was dissolved into 25 mL of ethanol to measure the acid value. The solution was heated to 70°C for 10 min then cooled at the room temperature. Using a phenolphthalein indicator, it was then titrated with 0.1N KOH solution. The transition to pink was the tipping moment. The AV was obtained using Eq. (2).
where AV = acid value, N = KOH normality (0.1N), V = titrate value (mL), W = weight of biodiesel (gram).
The determination of density of the oil was done by using the general density equation and measured in compliance with the ASTM D 4052-96 standard. This standardized method ensures the accurate and consistent measurement of oil density, providing valuable data for the biodiesel production process.
The following IS 15607 specifications are followed and computed using the formula:
where C = viscometer calibration constant (0.0336 cSt/s), and t = flow of time.
The ratio of the oil blend was determined by summing the API gravity of each oil to calculate the total API gravity. Subsequently, the API gravity of each individual oil was divided by the total API gravity, providing the respective proportions in the blend. Prior to the characterization of the oil blend’s properties, it was heated to 40°C using a magnetic stirrer. The properties of propellant so produced by blend of Karanja, Algal oil, and their combination are presented in Table 4. For a detailed understanding of the measurement methodology for these attributes, reference can be made to previous works by Karimi et al. [20] and Li et al. [41].
2.3 Transesterification Process
The oil blend for this experiment consisted of a 75% Karanja oil and 25% Algae oil ratio by taking 750 mL of Karanja and 250 mL of algae oil making total quantity of hybrid oil to be 1000 mL. The process was repeated thrice and thus we had 3 liters of concoction of hybrid oil. From this mixture we took 100 mL in a separate beaker of 250 mL and added 20 mL of methanol to it along with 1 gram of CaO catalyst and then positioned the beaker on the magnetic stirrer to ensure optimal homogeneity. The transesterification process unfolds on a electric hotplate endowed with a precisely modulated magnetic stirrer and a temperature sensor for meticulous control. This blended oil combination served as the feedstock for the transesterification process, aimed at producing biodiesel. The hybrid oil undergoes a meticulous preheating process at a temperature of 40°C to expunge any surplus water content. Under these process parameters the entire process involving 27 individual tests conducted within a magnetic stirrer at a constant stirring speed, facilitated by a hot plate stirrer was implemented:
a) Predetermined methanol-to-oil volumetric ratio ranging from 20% to 60% v/v,
b) Reaction time (variating between 60 and 180 min),
c) Reaction temperature (within the range of 30°C up until 90°C) and
d) Concentration of Catalyst (NaOH) (spanning from 0% to 2% w/w).
A Fractionating funnel was utilized to separate the fatty acid methyl ester (FAME) and glycerol layers, ultimately yielding pure biodiesel. FAME was found in the upper lamina of the assorted product, while 1,2,3-propanetriol accumulated in the bottom layer. Subsequently, the bio propellant phase went through a water rinsing process to eliminate adulterants up until the wastewater attained a neutral pH level. The ultimate product was scalded to 80°C to facilitate the evaporation of any unconsumed methyl alcohol. The yield of biodiesel can be determined by employing Eq. (5).
To ensure the quality and compliance of the produced biodiesel with industry standards, a physicochemical characterization was carried out in compliance with ASTM D6751 specifications [40]. The analysis necessitates the entailing of transesterification process for the blend of Karanja oil and Algal oil by utilizing the meticulously engineered Response Surface Methodology (RSM) with subsequent implementation of the Box-Behnken Design (BBD) at a consistent reaction speed. The acquired data was subjected to comprehensive statistical analysis and optimization procedures, facilitated through the use of the Minitab software, to ascertain the most favourable conditions for this biodiesel synthesis.
2.4 Response Surface Methodology (RSM)
Experimental design, often abbreviated as DOE (Design of Experiments), is an amalgamation of analytical techniques employed to create a model or systematically study a problem. The primary objective is to investigate standardized parameters concerning the enhancement of the intended reaction in relation to the process variables. Response Surface Methodology (RSM), a robust tool for commencing new processes, enhancing existing ones, designing new products, and overall process optimization, is one of the most extensively employed DOE techniques for constructing mathematical models [42]. RSM offers substantial advantages over conventional methods as it necessitates fewer experimental runs, facilitating a more rapid and cost-effective assessment of process parameters. Additionally, it allows for the exploration of interdependencies between various factors and the development of models to predict predefined responses [32].
Within RSM, the BBD is a prevalent and highly effective design method for creating experimental data suitable for quadratic modelling. BBD systematically explores the effectiveness of numerous elements spanning throughout a comprehensive range of distinct design points, while also consistently assessing curvature to accumulate a significant database for examining incongruity [33]. Response Surface Methodology is a DOE technique that constructs a framework in accordance to the correlation betwixt a response and multiple supervised independent elements, enabling multifaceted estimation and refinement. Eqs. (6) and (7) provide the fundamental frameworks grounded on primary and quadratic polynomial expressions that are often utilized in RSM, respectively. These models are invaluable for understanding and optimizing complex processes, offering a powerful tool for scientific inquiry and process improvement.
where
2.5 Artificial Neural Network (ANN)
Artificial Neural Network (ANN) is a widely recognized machine learning technique that has garnered significant attention in various engineering disciplines. It operates by transforming an input dataset into an output dataset. A prevalent type of neural network is the Multilayer Perceptron (MLP) network, which utilizes a feed-forward back-propagation (BP) approach. ANN offers a flexible simulation method that learns from the process by adjusting the network’s weight. In contrast to Response Surface Methodology (RSM), which is limited to variables within its control, ANN can accommodate a broader range of variables.
In this research, the ANN approach is employed to optimize the process variables. ANN is essentially a computer model inspired by the information processing mechanism of the human brain. Each layer of the neural network consists of a multitude of tiny entities called neurons, primarily dedicated to processing specific components. For this study, the artificial neural network analyzed 27 data points derived from independent factors, including temperature, time, catalyst concentration, and methanol-to-oil ratio. An MLP configuration, encompassing three layers comprising the input layer, hidden layer, and output layer, was utilized to facilitate the optimization process. This approach provides a powerful tool for exploring the interplay of variables and predicting optimal process conditions.
The perturbation plot in Fig. 2 illustrates the sensitivity of biodiesel yield to factors like methanol-to-oil ratio (A), temperature (B), time (C), and catalyst concentration (D). Notably, temperature (B) shows the most significant impact, particularly between 30°C and 60°C. Parameters C, D, and A also influence yield, albeit to a lesser extent. Understanding these sensitivities is crucial for optimizing biodiesel production [42].
Figure 2: Perturbation graph of biodiesel yield
Fig. 2 emphasizes the significant influence of factor B (reaction temperature) on biodiesel yield, supported by its pronounced slope and higher regression coefficients. The temperature range tested (30°C to 90°C) aligns with optimal yield conditions found in literature, with peak yields (>80%) observed around 55°C to 60°C. However, exceeding 60°C leads to reduced yield due to methanol evaporation.
In Fig. 2, biodiesel yield increases with catalyst concentration up to 1% (w/w), but then sharply declines [41]. Higher concentrations create a cavitation barrier, hindering transesterification, and increase soap formation. This leads to emulsification of glycerol and biodiesel, causing separation difficulties and yield decrease. These insights aid biodiesel production optimization [20,42].
The relationship between biodiesel yield (Y) and reaction time (C) is depicted in Fig. 2. It’s evident that biodiesel yield increases with the reaction time up to 100 min, after which it experiences a slight deterioration. This drop-in yield beyond 100 min could be attributed to the combination of high-temperature conditions and the potential saponification of triglycerides, which might occur over an extended reaction time [40].
Factor A, represented by the methanol-to-oil ratio, displayed a less pronounced slope compared to the other factors, indicating that this particular factor is less sensitive to all the responses. The experiment’s range for the methanol-to-oil ratio was selected to vary from a minimum of 6:1 to a maximum of 12:1 (mol/mol). An increase in biodiesel yield was observed as the methanol-to-oil ratio increased. However, beyond a certain threshold, increasing the methanol-to-oil ratio resulted in a significant decrease in density. This reduction in density suggests a decrease in the early collapse of cavitation bubbles and cavitation intensity, ultimately leading to reduced biodiesel yield [40].
Moreover, the decline in biodiesel yield with an increasing methanol-to-oil ratio can also be attributed to the solubility of methanol with glycerol, biodiesel, and other reactants. This increased solubility makes the separation of the products more challenging and contributes to emulsification [40]. Additionally, a larger amount of excess methanol at the end of the reaction can increase the cost associated with methanol separation [20]. These findings highlight the complex interplay of factors influencing biodiesel yield and underscore the importance of optimizing these variables for efficient production.
ANOVA, i.e., Analysis of variance was incorporated to evaluate the applicability and computational significance of the mathematical model. As depicted in Table 5, ANOVA helps identify the independent influences of each trait and their associations with biodiesel yield [44]. The importance of each independent variable is determined through the collected data, and the Fisher’s F-test (F-value) and probability value (p-value) play a crucial role in this assessment. A higher F-value and a lower p-value indicate the substantial effectiveness of an autonomous factors [45,46]. These constants are instrumental in gauging the meaningfulness of individual regression coefficients and the likelihood of anomaly.
The regression model’s F-value of 11.87 and a p-value less than 0.0001 affirm the model’s reliability within the experimental design.
Fig. 3 depicts the actual (experimental) data vs. the predicted data of biodiesel yield. As can be seen, the actual data were constantly distributed around a straight line (y = x), with a decent correlation (R2 = 95%) between such values.
Figure 3: Actual vs. predicted biodiesel yield
3.3 Artificial Neuron Network (ANN)
In ANN modeling, the configuration of network size, hidden layers, and neurons is vital for forecasting experimental results. Additionally, ANN can be employed in second-order polynomial regression, based on the RSM model. This study investigates an ANN model with one output, ten hidden, and four input neuron layers (1-10-4), representing variables like reaction time, temperature, methanol-to-oil ratio, and catalyst concentration, with biodiesel yield as the output.
The experimental yield dataset is split into training (70%), testing (15%), and validation (15%) sets to prevent overfitting and enhance model reliability. Regression coefficients for training, validation, and test networks are 0.99, 0.94, and 0.95, respectively, indicating high modeling accuracy. Linear regression of target plots vs. validation outputs yields a strong correlation (Output = 0.95 * Target + 3.9). Notably, the data distribution around a 45° angle signifies promising agreement between ANN predictions and experimental results.
Fig. 4 illustrates the ANN regression findings for general, validation, test, and training data, showing reasonable correlation coefficients across all datasets. Overall, the study concludes that the ANN model effectively optimizes significant parameters for biodiesel production.
Figure 4: Regression plots for (a) training, (b) validation, (c) test, and (d) overall prediction
Table 6 presents the iterations and allied reaction data points of produced biodiesel from RSM and ANN, respectively. The empirical statistics was fit with a quadratic polynomial model, and the resultant quadratic function was employed to illustrate the bio fuel productivity rooted in the categorical variables, as highlighted in Eq. (8).
where A, B, C, and D represent the coded forms of molar ratio, reaction temperature, time and catalyst concentration. The occurrence of a positive symbol in front of the terms implies a collaborative ramification, whereas the negative symbol suggests an inconsistence implication [21].
3.4 Outcome of Process Parameters on Conversion Rate of Biodiesel
The study investigated the significant effects of various working factors on biodiesel yield, employing a homogenous transesterification technique to derive biodiesel from a novel oil amalgamation of C. vulgaris and Karanja. The examination of alterations in reactions with each criterion variation, while clutching onto other components consistent at zero level values, shed light on the primary effects of the reaction parameters. Notably, all linear and quadratic terms were determined to be significant (p < 0.05) by ANOVA analysis, prompting an exploration of the main effects of all parameters to understand their influence on biodiesel yield.
3.4.1 Interval of Reaction and Methyl Alcohol to Oil Ratio
Surface and contour plots illustrated the impact of response time and methyl alcohol to oil molar ratio on amalgamated biodiesel yield. Optimal conditions involving a balanced reaction time and methanol to oil ratio were found to maximize biodiesel productivity, with excessive ratios leading to lessened productivity due to enhanced methyl alcohol solvency, complicating separation. These findings align with previous studies by Helmi et al. [47] and Elkelawy et al. [48], highlighting the importance of optimizing these parameters to achieve optimal biodiesel yield [20].
3.4.2 Reaction Temperature/Methanol to Oil Ratio
The study revealed a complex interplay between reaction temperature and methanol to oil molar ratio, with lower ratios and higher temperatures resulting in decreased yield due to reduced methanol vaporization loss [30,48]. Optimal conditions were found to gradually increase biodiesel yield up to a threshold, beyond which further increases led to diminishing returns [49]. These findings corroborate studies by Bai et al. [28] and Yusuff et al. [50], emphasizing the critical role of temperature and methanol to oil ratio in biodiesel production optimization.
3.4.3 Catalyst Concentration/Methanol to Oil Ratio
Analysis of catalyst concentration and methanol to oil ratio interactions revealed that lower concentrations and excessive ratios reduced catalyst and alcohol availability, leading to lower biodiesel yield [51]. Conversely, higher concentrations and optimal ratios enhanced yield, facilitating the transesterification process. These results are consistent with observations by Ghasemzadeh et al. [52] and Dutta et al. [53], highlighting the importance of optimizing these parameters to maximize biodiesel yield.
3.4.4 Catalyst Concentration/ Reaction Time
In the study of concentration of catalyst and response time, a 60-min reaction time coupled with a catalyst concentration of 1–1.2 wt.% yields optimal biodiesel output. Limited time and methoxy ions availability during the reaction contribute to reduced yield when both parameters are decreased. Conversely, lower catalyst concentration and longer reaction time lead to diminished yield. Industrial applications emphasize the critical role of catalyst concentration, affecting triglyceride conversion to FAME by enhancing active site availability. While increasing catalyst concentration improves yield, exceeding 1 wt.% leads to a significant drop. The interplay of reactants and catalyst greatly influences reaction yield. Athar et al. [54] and Maleki et al. [55] support these findings in their studies on Jatropha oil and canola oil optimization, respectively, while Ghasemzadeh et al. underscore the importance of reaction time in biodiesel yield [52].
3.4.5 Reaction Time/Reaction Temperature
Surface and contour plots demonstrated that optimal reaction time and temperature combinations promoted efficient methanol and oil diffusion, maximizing biodiesel yield [35]. However, temperatures exceeding optimal thresholds led to reduced yield due to increased methanol vapor pressure [23,48]. These findings echo previous studies by Cholapandian et al. [51] and Athar et al. [54], underscoring the significance of temperature and reaction time optimization in biodiesel production.
3.4.6 Reaction Temperature/Catalyst Concentration
Interactions between reaction temperature and catalyst concentration revealed peaks in yield, with optimal conditions maximizing biodiesel production. However, excessive concentrations beyond optimal thresholds led to negative consequences, primarily due to oil saponification. These findings are in line with insights put forth by Ajala et al. [22] and Rajendran et al. [56], emphasizing the importance of balancing concentration of catalyst and temperature for optimal biodiesel productivity.
3.5 Social, Environmental, and Economic Benefits of Adopting Biodiesel
Farmers and Agricultural Producers: Farmers benefit from biodiesel production by diversifying their revenue streams through the sale of oilseed crops used as feedstock. Increased demand for oilseed crops can lead to higher prices and improved market opportunities for farmers. Biodiesel production provides an additional market for surplus or low-quality crops, reducing waste and increasing overall agricultural productivity.
Biodiesel Producers: Biodiesel producers benefit from the growing demand for renewable fuels driven by environmental regulations, energy security concerns, and consumer preferences. Government incentives and subsidies for biodiesel production can improve the profitability and competitiveness of biodiesel manufacturing facilities. Technological advancements and process optimization contribute to cost reduction and increased efficiency in biodiesel production.
Consumers: Consumers benefit from biodiesel through reduced air pollution and improved air quality, leading to potential health benefits and lower healthcare costs. Biodiesel use in transportation can contribute to the mitigation of climate change by reducing greenhouse gas emissions compared to fossil fuels. The availability of biodiesel as an alternative fuel option provides consumers with choice and supports energy independence and security.
Government and Regulatory Agencies: Governments benefit from promoting biodiesel production and use through reduced dependence on imported fossil fuels, improved energy security, and environmental stewardship. Biodiesel blending mandates and tax incentives support the achievement of renewable energy targets, greenhouse gas reduction goals, and air quality standards. Investment in biodiesel infrastructure and research and development fosters economic growth, job creation, and technological innovation.
Environmental Organizations and Advocacy Groups: Environmental organizations advocate for biodiesel as a cleaner alternative to fossil fuels, promoting its use to mitigate climate change, reduce air pollution, and protect natural ecosystems. Increased adoption of biodiesel aligns with sustainability goals and supports the transition to a low-carbon economy, enhancing biodiversity and ecosystem resilience.
Transportation and Logistics Industry: The transportation and logistics sector benefits from biodiesel use as a renewable and domestically produced fuel that can help meet emissions reduction targets and regulatory requirements. Biodiesel-compatible vehicles and infrastructure support the integration of renewable fuels into existing transportation systems, reducing reliance on fossil fuels and improving air quality in urban areas.
The environmental (E)-factor is often employed to evaluate environmental sustainability of a chemical process. If the amount of E is large, it shows that the process produces a lot of waste, which is harmful to the environment. The subsequent Sheldon Eq. (9) was employed to estimate the value of E-factor [57].
The E-factor is calculated assuming that glycerol is a waste and biodiesel is the product. Furthermore, Eq. (9) eliminates the amount of water in total waste since addition of water greatly increases the value of E-factor which is not useful for comparing findings. The optimal value of E-factor should be zero because higher value implies, the process creates additional leftover and adverse environmental consequences. During the calculation of E-factor, biodiesel is treated as a product, methanol and catalyst are treated as reagents, and hybrid blend of Karanja and C. vulgaris oil is treated as raw material [58]. The quantity of E-factor for the biodiesel manufacturing process from hybrid oil of Karanja and C. vulgaris oil homogeneous catalyst is estimated to be 0.975 utilising Eq. (5). The methanol extracted from the biodiesel phase in the biodiesel preparation process may be reutilized in the transesterification process, and the by-product glycerol can be recycled in the production of commercial glycerol, hence Eq. (9) is revised as follows:
The amount of the E-factor was determined to be 0.0251 using Eq. (10). A low E-factor means that the biodiesel process produces less waste, is environmentally friendly, and may be used as a biodegradable fuel instead of fossil fuels originating from oil.
3.5.3 Economic Viability of Bio Propellant
Production Cost: Biodiesel research must focus on reducing production costs to unlock its potential. High costs impede widespread adoption and economic viability, making the research futile without affordable alternatives. Competitive production costs are essential for biodiesel to rival traditional fuels and support sustainable energy transitions.
Feedstock Availability and Price Stability: The availability and price stability of feedstocks are crucial factors in the economic feasibility of biodiesel production. Unlike fossil fuels, which are subject to global market fluctuations, biodiesel feedstocks can sometimes be locally sourced and may offer more stable pricing over time. However, competition for feedstocks between biodiesel production and other industries (such as food production) can impact availability and prices.
Government Incentives and Regulations: Many governments offer incentives and subsidies for biodiesel production. Regulatory requirements, such as blending mandates and tax incentives for biodiesel use, can create market demand and support the economic viability of biodiesel production.
Environmental Benefits: The potential for carbon credits and other environmental incentives can enhance the economic attractiveness of biodiesel production.
3.6 Optimal Conditions of the Biodiesel Yield and Validation of Modal
The ideal values of each of the variables influencing the reaction were found using the regression equation generated from RSM followed by BBD model to optimize the effectiveness production of biodiesel during the transesterification reaction. The ideal reaction conditions were found by modifying the independent variables in the experimental range and maximizing the efficiency of biodiesel yield with the greatest degree of relevance (+5), simultaneously reducing the standard deviation in the optimization portion of the program with a moderate level of significance (+3).
The most favorable indicators confirmed from the RSM for the transesterification of blends of C. vulgaris oil and Karanja oil to engender bio propellant are displayed in Fig. 5. The maximum 98% yield of biodiesel was achieved with desirability 94% at the ideal operational temperature of 56.86°C, amount of catalyst being at 1% (w/w), with timespan of reaction being at 91.47 min, and Methyl alcohol to oil molar ratio of 8.46:1 as indicated in the Fig. 6.
Figure 5: Depicts effect of various process parameters on biodiesel yield figure (a) reaction time and methanol to oil ratio, (b) reaction temperature/methanol to oil ratio, (c) catalyst concentration/methanol to oil ratio, (d) catalyst concentration/reaction time, (e) reaction time/reaction temperature and (f) reaction temperature/catalyst concentration
Figure 6: Optimized process parameter and biodiesel yield
3.6.1 Characteristics of Biofuel
The physical, chemical and propellant-related attributes of the produced bio propellant were rigorously evaluated leveraging established bio propellant factors. These assessments are crucial for determining the feasibility of utilizing this biofuel in internal combustion (I.C.) engines. The evaluation was conducted in accordance with the standard techniques recommended by the AOAC in 1997. Notably, the results obtained from these analyses were found to be in accordance with the established biodiesel standards, including EN 14214 and ASTM D6751, confirming the quality and suitability of the produced biofuel for use as an automotive fuel.
The obtained biodiesel was evaluated and scrutinized after scouring and decontaminating to ascertain its attributes. The revelations implied that the viscosity of bio propellant, is primary characteristics properties of biodiesel, was enclosed within the spectrum validated by EN 14214 and ASTM D6751, with a value of 4.21 cst (mm2/s) at 40°C. Table 7 covers attributes of biod fuel acquired from composite oil. One downside of camelina oil biodiesel was the high amount of unsaturated fatty acids C18:2 and C18:3, which increased the iodine value.
In this study, biodiesel was derived from a novel blend of Karanja oil and C. vulgaris using a homogenous transesterification technique. The research aimed to establish a framework for the transesterification of a blend consisting of low FFA (0.54%) microalgal oil and high FFA (2%) Karanja oil, resulting in a hybrid oil with 1% free fatty acid content, suitable for a single-step transesterification process.
Key conclusions drawn from this research include:
1. Optimization and Yield: Using Response Surface Methodology (RSM), we achieved a maximum biodiesel yield of 98% under optimal conditions (56.86°C reaction temperature, 91.47 min of reaction time, 8.46:1 methanol to oil ratio, and 1.09 weight% catalyst concentration). This high yield highlights the efficiency of the process.
2. Environmental Impact: The Environmental factor (E-factor) was calculated to be 0.0251, indicating minimal waste generation. This low E-factor underscores the environmental benefits of the process, demonstrating its potential as a sustainable alternative to fossil fuel-based diesel.
3. Fuel Quality: The biodiesel produced meets ASTM standards, with notable improvements in fuel properties such as lower density (852.25 kg/m3) and viscosity (3.82 mm2/s) compared to Karanja biodiesel (viscosity-5.59 mm2/s and density-860 kg/m3), and higher calorific value (41.52 MJ/kg) and cetane number (54.34) compared to C. vulgaris biodiesel (Gross Calorific value (42.3 KJ/kg), Cetane value (55.56)). These properties make it highly suitable for diesel engines.
4. Cost Efficiency: The use of a blended oil system significantly reduces the required reaction temperature and catalyst concentration, thereby lowering the overall production costs. This presents a cost-effective method for biodiesel production.
5. Industrial and Environmental Relevance: The findings suggest that incorporating algal oil can effectively reduce the FFA content of high FFA oils like Karanja oil, enhancing the input parameters for biodiesel production. This approach offers a viable pathway for utilizing diverse oil sources.
6. Future Research and Applications: Beyond the current methods, future studies could explore alternative oil blends and advanced optimization techniques, such as artificial intelligence algorithms or evolutionary algorithms. Additionally, the by-products of biodiesel production, such as glycerol, can be valorized for use in the cosmetic industry or converted into other high-value chemicals, adding economic value to the production process.
This research provides a significant step forward in biodiesel production, offering an economically viable, environmentally friendly, and high-quality alternative fuel. The insights gained from this study have the potential to expand the horizons of biodiesel production technology and its industrial applications.
Acknowledgement: The authors would like to express their deepest gratitude to Energy Centre, MANIT, Bhopal for their unwavering support and M.P. Council of Science and Technology for the financial support provided for this research project entitled “Enhancement of Cold Flow Properties of Waste Cooking Biodiesel and Diesel” under the File Number A/RD/RP-2/345 for the above publication.
Funding Statement: We would like to thank M.P. Council of Science and Technology for the financial support provided for this research project entitled “Enhancement of Cold Flow Properties of Waste Cooking Biodiesel and Diesel” under the File Number A/RD/RP-2/345 for the above publication.
Author Contributions: Sujeet Kesharvani: Conceptualization, Data curation, Formal analysis, Writing—original draft. Sakhi Katre: Writing—original draft, Data curation, Methodology, Software, Validation, Writing—review & editing. Suyasha Pandey: Writing—original draft, Data curation, Methodology, Writing—review & editing. Gaurav Dwivedi: Methodology, Validation, Visualization & Supervision. Tikendra Nath Verma: Methodology, Validation, Visualization & Supervision. Prashant Baredar: Supervision. All authors reviewed the results and approved the final version of the manuscript.
Availability of Data and Materials: No data was used for the research described in the article.
Conflicts of Interest: The authors declare that they have no conflicts of interest to report regarding the present study.
References
1. H. Kamyab, A. Naderipour, M. Jahannoush, A. Abdullah, and M. H. Marzbali, “Potential effect of SARS-CoV-2 on solar energy generation: Environmental dynamics and implications,” Sustain. Energy Technol. Assessments, vol. 52, no. 4, pp. 102027, Aug. 2022. doi: 10.1016/j.seta.2022.102027. [Google Scholar] [CrossRef]
2. M. S. Mastoi et al., “A critical analysis of the impact of pandemic on China’s electricity usage patterns and the global development of renewable energy,” Int. J. Environ. Res. Public Heal, vol. 19, no. 8, pp. 4608, 2022. doi: 10.3390/ijerph19084608. [Google Scholar] [PubMed] [CrossRef]
3. Y. M. Oo, A. Legwiriyakul, J. Thawornprasert, and K. Somnuk, “Production of diesel-biodiesel-water fuel nanoemulsions using three-dimensional printed rotor-stator hydrodynamic cavitation,” Fuel, vol. 317, no. 3, pp. 123445, Jun. 2022. doi: 10.1016/j.fuel.2022.123445. [Google Scholar] [CrossRef]
4. M. U. Qadeer et al., “Review of biodiesel synthesis technologies, current trends, yield influencing factors and economical analysis of supercritical process,” J. Clean. Prod., vol. 309, no. 5, pp. 127388, Aug. 2021. doi: 10.1016/j.jclepro.2021.127388. [Google Scholar] [CrossRef]
5. M. A. Bashir, S. Wu, J. Zhu, A. Krosuri, M. U. Khan and R. J. Ndeddy Aka, “Recent development of advanced processing technologies for biodiesel production: A critical review,” Fuel Process. Technol., vol. 227, no. 3, pp. 107120, Mar. 2022. doi: 10.1016/j.fuproc.2021.107120. [Google Scholar] [CrossRef]
6. S. Brahma et al., “Biodiesel production from mixed oils: A sustainable approach towards industrial biofuel production,” Chem. Eng. J. Adv., vol. 10, no. 4, pp. 100284, May 2022. doi: 10.1016/j.ceja.2022.100284. [Google Scholar] [CrossRef]
7. S. Parida, M. Singh, and S. Pradhan, “Biomass wastes: A potential catalyst source for biodiesel production,” Bioresour Technol. Reports, vol. 18, no. 7, pp. 101081, Jun. 2022. doi: 10.1016/j.biteb.2022.101081. [Google Scholar] [CrossRef]
8. M. Munir et al., “Production of high quality biodiesel from novel non-edible Raphnus raphanistrum L. seed oil using copper modified montmorillonite clay catalyst,” Environ. Res., vol. 193, no. 1, pp. 110398, Feb. 2021. doi: 10.1016/j.envres.2020.110398. [Google Scholar] [PubMed] [CrossRef]
9. Y. Guo, X. Gou, Z. Xu, and M. Skare, “Carbon pricing mechanism for the energy industry: A bibliometric study of optimal pricing policies,” Acta Montan. Slovaca, vol. 27, no. 1, pp. 49–69, 2022. doi: 10.46544/AMS.v27i1.05. [Google Scholar] [CrossRef]
10. P. Maheshwari et al., “A review on latest trends in cleaner biodiesel production: Role of feedstock, production methods, and catalysts,” J. Clean. Prod., vol. 355, no. 14, pp. 131588, Jun. 2022. doi: 10.1016/j.jclepro.2022.131588. [Google Scholar] [CrossRef]
11. D. Singh et al., “A comprehensive review of physicochemical properties, production process, performance and emissions characteristics of 2nd generation biodiesel feedstock: Jatropha curcas,” Fuel, vol. 285, no. 082208, pp. 119110, Feb. 2021. doi: 10.1016/j.fuel.2020.119110. [Google Scholar] [CrossRef]
12. M. Anwar, “Biodiesel feedstocks selection strategies based on economic, technical, and sustainable aspects,” Fuel, vol. 283, no. 1, pp. 119204, Jan. 2021. doi: 10.1016/j.fuel.2020.119204. [Google Scholar] [CrossRef]
13. M. Kumar, Y. Sun, R. Rathour, A. Pandey, I. S. Thakur and D. C. W. Tsang, “Algae as potential feedstock for the production of biofuels and value-added products: Opportunities and challenges,” Sci. Total Environ., vol. 716, no. 1, pp. 137116, May 2020. doi: 10.1016/j.scitotenv.2020.137116. [Google Scholar] [PubMed] [CrossRef]
14. N. Hossain, M. H. Hasan, T. M. I. Mahlia, A. H. Shamsuddin, and A. S. Silitonga, “Feasibility of microalgae as feedstock for alternative fuel in Malaysia: A review,” Energy Strateg. Rev., vol. 32, no. 2, pp. 100536, Nov. 2020. doi: 10.1016/j.esr.2020.100536. [Google Scholar] [CrossRef]
15. J. Maroušek, B. Gavurová, O. Strunecký, A. Maroušková, M. Sekar and V. Marek, “Techno-economic identification of production factors threatening the competitiveness of algae biodiesel,” Fuel, vol. 344, no. 1, pp. 128056, Jul. 2023. doi: 10.1016/j.fuel.2023.128056. [Google Scholar] [CrossRef]
16. B. Karmakar, S. Pal, K. Gopikrishna, O. N. Tiwari, and G. Halder, “Injection of superheated C1 and C3 alcohols in non-edible Pongamia pinnata oil for semi-continuous uncatalyzed biodiesel synthesis,” Renew Energy, vol. 185, no. 5, pp. 850–861, Feb. 2022. doi: 10.1016/j.renene.2021.12.109. [Google Scholar] [CrossRef]
17. P. Moradi and M. Saidi, “Biodiesel production from Chlorella Vulgaris microalgal-derived oil via electrochemical and thermal processes,” Fuel Process. Technol., vol. 228, no. 2, pp. 107158, Apr. 2022. doi: 10.1016/J.FUPROC.2021.107158. [Google Scholar] [CrossRef]
18. D. Jain et al., “CO2 fixation and production of biodiesel by Chlorella vulgaris NIOCCV under mixotrophic cultivation,” Bioresour. Technol., vol. 273, pp. 672–676, Nov. 2018. doi: 10.1016/j.biortech.2018.09.148. [Google Scholar] [CrossRef]
19. H. Singh Pali, A. Sharma, N. Kumar, and Y. Singh, “Biodiesel yield and properties optimization from Kusum oil by RSM,” Fuel, vol. 291, pp. 120218, May 2021. doi: 10.1016/j.fuel.2021.120218. [Google Scholar] [CrossRef]
20. S. Karimi and M. Saidi, “Biodiesel production from Azadirachta India-derived oil by electrolysis technique: Process optimization using response surface methodology (RSM),” Fuel Process. Technol., vol. 234, pp. 107337, Sep. 2022. doi: 10.1016/j.fuproc.2022.107337. [Google Scholar] [CrossRef]
21. A. Attari, A. Abbaszadeh-Mayvan, and A. Taghizadeh-Alisaraie, “Process optimization of ultrasonic-assisted biodiesel production from waste cooking oil using waste chicken eggshell-derived CaO as a green heterogeneous catalyst,” Biomass Bioenergy, vol. 158, pp. 106357, Mar. 2022. doi: 10.1016/j.biombioe.2022.106357. [Google Scholar] [CrossRef]
22. E. O. Ajala, A. B. Ehinmowo, M. A. Ajala, O. A. Ohiro, F. A. Aderibigbe and A. O. Ajao, “Optimisation of CaO-Al2O3-SiO2-CaSO4-based catalysts performance for methanolysis of waste lard for biodiesel production using response surface methodology and meta-heuristic algorithms,” Fuel Process. Technol., vol. 226, no. 4, pp. 107066, Feb. 2022. doi: 10.1016/j.fuproc.2021.107066. [Google Scholar] [CrossRef]
23. N. K. Singh, Y. Singh, and A. Sharma, “Optimization of biodiesel synthesis from Jojoba oil via supercritical methanol: A response surface methodology approach coupled with genetic algorithm,” Biomass Bioenergy, vol. 156, no. 7, pp. 106332, Jan. 2022. doi: 10.1016/j.biombioe.2021.106332. [Google Scholar] [CrossRef]
24. M. Helmi, K. Tahvildari, A. Hemmati, P. A. Azar, and A. Safekordi, “Converting waste cooking oil into biodiesel using phosphomolybdic acid/clinoptilolite as an innovative green catalyst via electrolysis procedure; optimization by response surface methodology (RSM),” Fuel Process. Technol., vol. 225, Jan. 2022. doi: 10.1016/j.fuproc.2021.107062. [Google Scholar] [CrossRef]
25. S. Sivarethinamohan, J. R. Hanumanthu, K. Gaddam, G. Ravindiran, and A. Alagumalai, “Towards sustainable biodiesel production by solar intensification of waste cooking oil and engine parameter assessment studies,” Sci. Total Environ., vol. 804, pp. 150236, Sep. 2021. doi: 10.1016/j.scitotenv.2021.150236. [Google Scholar] [PubMed] [CrossRef]
26. G. Vaidya, B. T. Nalla, D. K. Sharma, J. Thangaraja, Y. Devarajan and V. Sorakka Ponnappan, “Production of biodiesel from phoenix sylvestris oil: Process optimisation technique,” Sustain. Chem. Pharm., vol. 26, no. 2, pp. 100636, May 2022. doi: 10.1016/j.scp.2022.100636. [Google Scholar] [CrossRef]
27. F. Helmi, M. Helmi, and A. Hemmati, “Phosphomolybdic acid/chitosan as acid solid catalyst using for biodiesel production from pomegranate seed oil via microwave heating system: RSM optimization and kinetic study,” Renew. Energy, vol. 189, pp. 881–898, Apr. 2022. doi: 10.1016/j.renene.2022.02.123. [Google Scholar] [CrossRef]
28. H. Bai, J. Tian, D. Talifu, K. Okitsu, and A. Abulizi, “Process optimization of esterification for deacidification in waste cooking oil: RSM approach and for biodiesel production assisted with ultrasonic and solvent,” Fuel, vol. 318, no. 6, pp. 123697, Jun. 2022. doi: 10.1016/j.fuel.2022.123697. [Google Scholar] [CrossRef]
29. O. D. Samuel et al., “Performance comparison of empirical model and particle swarm optimization & its boiling point prediction models for waste sunflower oil biodiesel,” Case Stud. Therm. Eng., vol. 33, no. 1, pp. 101947, May 2022. doi: 10.1016/j.csite.2022.101947. [Google Scholar] [CrossRef]
30. A. N. Amenaghawon, K. Obahiagbon, V. Isesele, and F. Usman, “Optimized biodiesel production from waste cooking oil using a functionalized bio-based heterogeneous catalyst,” Clean Eng. Technol., vol. 8, pp. 100501, Jun. 2022. doi: 10.1016/j.clet.2022.100501. [Google Scholar] [CrossRef]
31. D. Kumar, T. Das, B. S. Giri, E. R. Rene, and B. Verma, “Biodiesel production from hybrid non-edible oil using bio-support beads immobilized with lipase from Pseudomonas cepacia,” Fuel, vol. 255, no. 20, pp. 115801, Nov. 2019. doi: 10.1016/j.fuel.2019.115801. [Google Scholar] [CrossRef]
32. J. Milano et al., “Physicochemical property enhancement of biodiesel synthesis from hybrid feedstocks of waste cooking vegetable oil and beauty leaf oil through optimized alkaline-catalysed transesterification,” Waste Manag., vol. 80, pp. 435–449, Oct. 2018. doi: 10.1016/j.wasman.2018.09.005. [Google Scholar] [PubMed] [CrossRef]
33. A. Saydut, S. Erdogan, A. B. Kafadar, C. Kaya, F. Aydin and C. Hamamci, “Process optimization for production of biodiesel from hazelnut oil, sunflower oil and their hybrid feedstock,” Fuel, vol. 183, no. 16, pp. 512–517, Nov. 2016. doi: 10.1016/j.fuel.2016.06.114. [Google Scholar] [CrossRef]
34. B. Esther Olubunmi, A. Fatai Alade, S. Ogbeide Ebhodaghe, and O. Tokunbo Oladapo, “Optimization and kinetic study of biodiesel production from beef tallow using calcium oxide as a heterogeneous and recyclable catalyst,” Energy Convers. Manag. X, vol. 14, no. 5, pp. 100221, May 2022. doi: 10.1016/J.ECMX.2022.100221. [Google Scholar] [CrossRef]
35. R. Foroutan, S. J. Peighambardoust, R. Mohammadi, S. H. Peighambardoust, and B. Ramavandi, “Application of waste chalk/CoFe2O4/K2CO3 composite as a reclaimable catalyst for biodiesel generation from sunflower oil,” Chemosphere, vol. 289, no. 12, pp. 133226, Feb. 2022. doi: 10.1016/j.chemosphere.2021.133226. [Google Scholar] [PubMed] [CrossRef]
36. P. Kalita, B. Basumatary, P. Saikia, B. Das, and S. Basumatary, “Biodiesel as renewable biofuel produced via enzyme-based catalyzed transesterification,” Energy Nexus, vol. 6, no. 2, pp. 100087, Jun. 2022. doi: 10.1016/j.nexus.2022.100087. [Google Scholar] [CrossRef]
37. J. Liu, G. Chen, B. Yan, W. Yi, and J. Yao, “Biodiesel production in a magnetically fluidized bed reactor using whole-cell biocatalysts immobilized within ferroferric oxide-polyvinyl alcohol composite beads,” Bioresour Technol., vol. 355, no. 1, pp. 127253, Jul. 2022. doi: 10.1016/j.biortech.2022.127253. [Google Scholar] [PubMed] [CrossRef]
38. K. Mahmodi, M. Mostafaei, and E. Mirzaee-Ghaleh, “Detecting the different blends of diesel and biodiesel fuels using electronic nose machine coupled ANN and RSM methods,” Sustain. Energy Technol. Assessments, vol. 51, no. 1, pp. 101914, Jun. 2022. doi: 10.1016/j.seta.2021.101914. [Google Scholar] [CrossRef]
39. C. M. Agu, C. C. Orakwue, M. C. Menkiti, A. C. Agulanna, and F. C. Akaeme, “RSM/ANN based modeling of methyl esters yield from Anacardium occidentale kernel oil by transesterification, for possible application as transformer fluid,” Curr. Res. Green Sustain. Chem., vol. 5, no. 4, pp. 100255, Jan. 2022. doi: 10.1016/j.crgsc.2021.100255. [Google Scholar] [CrossRef]
40. T. F. Adepoju, H. A. Akens, and E. B. Ekeinde, “Synthesis of biodiesel from blend of seeds oil-animal fat employing agricultural wastes as base catalyst,” Case Stud. Chem. Environ. Eng., vol. 5, no. 16, pp. 100202, May 2022. doi: 10.1016/J.CSCEE.2022.100202. [Google Scholar] [CrossRef]
41. X. Li et al., “Optimization of key parameters using RSM for improving the production of the green biodiesel from FAME by hydrotreatment over Pt/SAPO-11,” Biomass Bioenergy, vol. 158, no. 19, pp. 106379, Mar. 2022. doi: 10.1016/j.biombioe.2022.106379. [Google Scholar] [CrossRef]
42. A. P. Bora, L. D. N. V. V. Konda, S. Pasupuleti, and K. S. Durbha, “Synthesis of MgO/MgSO4 nanocatalyst by thiourea-nitrate solution combustion for biodiesel production from waste cooking oil,” Renew. Energy, vol. 190, pp. 474–486, May 2022. doi: 10.1016/j.renene.2022.03.127. [Google Scholar] [CrossRef]
43. S. Simsek, S. Uslu, and H. Simsek, “Proportional impact prediction model of animal waste fat-derived biodiesel by ANN and RSM technique for diesel engine,” Energy, vol. 239, no. 2, pp. 122389, Jan. 2022. doi: 10.1016/j.energy.2021.122389. [Google Scholar] [CrossRef]
44. E. C. Pham et al., “Optimization of microwave-assisted biodiesel production from waste catfish using response surface methodology,” Energy Rep., vol. 8, no. 57, pp. 5739–5752, Nov. 2022. doi: 10.1016/j.egyr.2022.04.036. [Google Scholar] [CrossRef]
45. J. Du, R. K. Yuan, R. X. Hu, H. L. Zhang, Y. T. Qi and W. N. Zhang, “Biodiesel production from Momordica cochinchinensis (Lour.) spreng seed oil,” Fuel, vol. 314, no. 8–9, pp. 123047, Apr. 2022. doi: 10.1016/j.fuel.2021.123047. [Google Scholar] [CrossRef]
46. G. Muhammad et al., “Enhanced biodiesel production from wet microalgae biomass optimized via response surface methodology and artificial neural network,” Renew. Energy, vol. 184, no. 17, pp. 753–764, Jan. 2022. doi: 10.1016/j.renene.2021.11.091. [Google Scholar] [CrossRef]
47. M. Helmi, M. Ghadiri, K. Tahvildari, and A. Hemmati, “Biodiesel synthesis using clinoptilolite-Fe3O4-based phosphomolybdic acid as a novel magnetic green catalyst from salvia mirzayanii oil via electrolysis method: Optimization study by Taguchi method,” J. Environ. Chem. Eng., vol. 9, no. 5, pp. 105988, Oct. 2021. doi: 10.1016/j.jece.2021.105988. [Google Scholar] [CrossRef]
48. M. Elkelawy et al., “Maximization of biodiesel production from sunflower and soybean oils and prediction of diesel engine performance and emission characteristics through response surface methodology,” Fuel, vol. 266, no. 1, pp. 117072, Apr. 2020. doi: 10.1016/j.fuel.2020.117072. [Google Scholar] [CrossRef]
49. C. Jia et al., “Abscisic acid improves the safflower seed oil content for biodiesel production via CtDof2 gene regulation,” Ind. Crops Prod., vol. 184, no. 7, pp. 115020, Sep. 2022. doi: 10.1016/j.indcrop.2022.115020. [Google Scholar] [CrossRef]
50. A. S. Yusuff, O. D. Adeniyi, S. O. Azeez, M. A. Olutoye, and U. G. Akpan, “Synthesis and characterization of anthill-eggshell-Ni-Co mixed oxides composite catalyst for biodiesel production from waste frying oil, biofuels,” Bioprod. Biorefining, vol. 13, no. 1, pp. 37–47, Jan. 2019. doi: 10.1002/bbb.1914. [Google Scholar] [CrossRef]
51. K. Cholapandian, B. Gurunathan, and N. Rajendran, “Investigation of CaO nanocatalyst synthesized from Acalypha indica leaves and its application in biodiesel production using waste cooking oil,” Fuel, vol. 312, no. 1, pp. 122958, Mar. 2022. doi: 10.1016/j.fuel.2021.122958. [Google Scholar] [CrossRef]
52. B. Ghasemzadeh, A. A. Matin, B. Habibi, and M. Ebadi, “Rubber-Fe3O4@SiO2@H3PMo12O40 as heterogeneous catalyst for biodiesel production: Optimized by response surface methodology,” Mater. Chem. Phys., vol. 287, pp. 126268, Aug. 2022. doi: 10.1016/j.matchemphys.2022.126268. [Google Scholar] [CrossRef]
53. P. Dutta, B. Biswas, B. Pal, M. Majumder, and A. K. Das, “Response surface methodology-based optimization of parameters for biodiesel production,” Sustain. Dev. by Artif. Intell. Mach. Learn. Renew. Energies., vol. 38, no. 4, pp. 321–339, Jan. 2022. doi: 10.1016/B978-0-323-91228-0.00002-1. [Google Scholar] [CrossRef]
54. M. Athar et al., “Biodiesel production by single-step acid-catalysed transesterification of Jatropha oil under microwave heating with modelling and optimisation using response surface methodology,” Fuel, vol. 322, no. 60, pp. 124205, Aug. 2022. doi: 10.1016/j.fuel.2022.124205. [Google Scholar] [CrossRef]
55. B. Maleki and S. S. Ashraf Talesh, “Optimization of ZnO incorporation to αFe2O3 nanoparticles as an efficient catalyst for biodiesel production in a sonoreactor: Application on the CI engine,” Renew. Energy, vol. 182, pp. 43–59, Jan. 2022. doi: 10.1016/j.renene.2021.10.013. [Google Scholar] [CrossRef]
56. N. Rajendran, D. Kang, J. Han, and B. Gurunathan, “Process optimization, economic and environmental analysis of biodiesel production from food waste using a citrus fruit peel biochar catalyst,” J. Clean. Prod., vol. 365, pp. 132712, Sep. 2022. doi: 10.1016/j.jclepro.2022.132712. [Google Scholar] [CrossRef]
57. A. Alagumalai, O. Mahian, F. Hollmann, and W. Zhang, “Environmentally benign solid catalysts for sustainable biodiesel production: A critical review,” Sci. Total Environ., vol. 768, no. 1, pp. 144856, May 2021. doi: 10.1016/j.scitotenv.2020.144856. [Google Scholar] [PubMed] [CrossRef]
58. F. Fangfang, A. Alagumalai, and O. Mahian, “Sustainable biodiesel production from waste cooking oil: ANN modeling and environmental factor assessment,” Sustain. Energy Technol. Assessments, vol. 46, no. 7, pp. 101265, Aug. 2021. doi: 10.1016/j.seta.2021.101265. [Google Scholar] [CrossRef]
Cite This Article
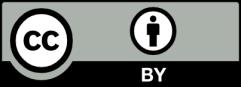
This work is licensed under a Creative Commons Attribution 4.0 International License , which permits unrestricted use, distribution, and reproduction in any medium, provided the original work is properly cited.