Open Access
ARTICLE
Predicting Turbidite Channel in Deep-Water Canyon Based on Grey Relational Analysis-Support Vector Machine Model: A Case Study of the Lingshui Depression in Qiongdongnan Basin, South China Sea
1 School of Earth and Space Sciences, Peking University, Beijing, 100871, China
2 Key Laboratory of Orogenic Belts and Crustal Evolution, Peking University, Beijing, 100871, China
3 Geotechnical Physical and Mechanical Properties Laboratory, Langfang Normal University, Langfang, 065000, China
4 Heilongjiang Northeast Petroleum University Science Park Development Co., Ltd., Daqing, 163318, China
5 College of Petroleum Engineering, Northeast Petroleum University, Daqing, 163318, China
* Corresponding Authors: Li Li. Email: ; Zhandong Li. Email:
(This article belongs to the Special Issue: Carbon Reduction and Optimized Recovery Strategies for Unconventional Resource)
Energy Engineering 2024, 121(9), 2435-2447. https://doi.org/10.32604/ee.2024.050771
Received 17 February 2024; Accepted 17 May 2024; Issue published 19 August 2024
Abstract
The turbidite channel of South China Sea has been highly concerned. Influenced by the complex fault and the rapid phase change of lithofacies, predicting the channel through conventional seismic attributes is not accurate enough. In response to this disadvantage, this study used a method combining grey relational analysis (GRA) and support vector machine (SVM) and established a set of prediction technical procedures suitable for reservoirs with complex geological conditions. In the case study of the Huangliu Formation in Qiongdongnan Basin, South China Sea, this study first dimensionalized the conventional seismic attributes of Gas Layer Group I and then used the GRA method to obtain the main relational factors. A higher relational degree indicates a higher probability of responding to the attributes of the turbidite channel. This study then accumulated the optimized attributes with the highest relational factors to obtain a first-order accumulated sequence, which was used as the input training sample of the SVM model, thus successfully constructing the SVM turbidite channel model. Drilling results prove that the GRA-SVM method has a high drilling coincidence rate. Utilizing the core and logging data and taking full use of the advantages of seismic inversion in predicting the sand boundary of water channels, this study divides the sedimentary microfacies of the Huangliu Formation in the Lingshui 17-2 Gas Field. This comprehensive study has shown that the GRA-SVM method has high accuracy for predicting turbidite channels and can be used as a superior turbidite channel prediction method under complex geological conditions.Keywords
Cite This Article
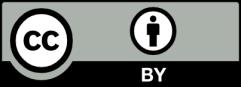
This work is licensed under a Creative Commons Attribution 4.0 International License , which permits unrestricted use, distribution, and reproduction in any medium, provided the original work is properly cited.