Open Access
EDITORIAL
Key Issues for Modelling, Operation, Management and Diagnosis of Lithium Batteries: Current States and Prospects
1 Faculty of Electric Power Engineering, Kunming University of Science and Technology, Kunming, 650500, China
2 State Key Laboratory of Alternate Electrical Power System with Renewable Energy Sources, North China Electric Power University, Beijing, 102206, China
3 School of Engineering, University of Lincoln, Lincoln, LN6 7TS, UK
4 Department of Electrical and Electronic Engineering, The University of Hong Kong, Hong Kong, 999077, China
* Corresponding Author: Bo Yang. Email:
(This article belongs to the Special Issue: Advanced Modelling, Operation, Management and Diagnosis of Lithium Batteries)
Energy Engineering 2024, 121(8), 2085-2091. https://doi.org/10.32604/ee.2024.050083
Received 26 January 2024; Accepted 25 March 2024; Issue published 19 July 2024
Abstract
This article has no abstract.Keywords
With the continuous growth of the global population, the energy demand continues to increase. However, due to the dominance of fossil fuels in global energy and fossil fuels are non-renewable, it has led to the global energy crisis [1]. Besides, the use of fossil fuels will generate a mass of air pollutants (e.g., carbon dioxide, sulfur dioxide, etc.), which will cause serious environmental pollution, climate change [2], etc. To resolve the aforementioned issues, countries around the world have implemented a variety of measures hoping to fundamentally adjust the global energy structure and achieve sustainable development. Thereinto, “Paris Agreement” reached in 2015 under the framework of “United Nations Framework Convention on Climate Change” aims to control the increase in the average temperature of the globe to within 2°C below preindustrial levels, and thereafter to peak global greenhouse gas emissions as soon as possible, continuously decreasing thereafter [3]. United Kingdom plans to reduce the average exhaust emissions of “new cars” to approximately 50–70 g/km by 20230, which is roughly half of what it is now [4]. In addition, China proposed a plan at “United Nations General Assembly” in 2020 to peak carbon dioxide emissions by 2030 and strive to achieve carbon neutrality by 2060. It is a fact that the whole world is committed to changing the current energy structure, protecting the Earth’s ecology, and achieving global sustainable development [5].
Based on the above background, electric vehicles (EVs) have been vigorously promoted and used in various countries around the world. Compared to gasoline vehicles, EVs have the advantages of no exhaust emissions, low noise, no pollution to the environment, and low operating costs [6]. Power batteries are the core components of EVs, and lithium batteries currently occupy a dominant position in EVs power batteries due to unique advantages such as long cycle life, low self-discharge rate [7], etc. Therefore, conducting relevant research on lithium batteries is meaningful for enhancing the performance of batteries and promoting the blossoming of EVs industry.
To ensure the safety, stability, and durability of lithium batteries during charging and discharging, effective management is crucial. It can ensure that the battery can safely produce optimal performance with a sufficiently long lifespan. Battery management system (BMS) can effectively control, monitor, and manage lithium batteries, consisting of various sensors, actuators, controllers, and signal lines, and is one of the core components of the battery system [8]. However, lithium batteries have very complex electrochemical characteristics inside and are easily affected by operating conditions and environmental factors such as environmental temperature and discharge depth. Therefore, establishing accurate models is a prerequisite for battery control and management. Through precise models can gain a deeper understanding of the chemical and physical processes inside the battery, providing a foundation for performance optimization [9]. State estimation and prediction are also vital functions of BMS, which include state of charge (SOC), state of health (SOH), state of power (SOP), and state of energy (SOE). Accurate state estimation can provide crucial guidance for the operation and extend the lifespan of batteries to some degree. In addition, various faults are inevitable during the operation of batteries. Reliable fault diagnosis techniques can timely and effectively diagnose faults, efficiently guide the elimination and prevention of battery system faults, and reduce the cost of battery operation [10].
In summary, the development of advanced and efficient modeling, operation, management, and diagnosis technologies for lithium batteries is of great significance. This work investigates and discusses the key issues currently existing in lithium batteries from three comprehensive aspects, introduces the currently advanced technologies, and finally looks forward to future research on lithium batteries from five perspectives. This work can promote the progress of the new energy industry and make significant contributions to the global energy revolution to a certain extent.
2.1 Accurate Modelling of Batteries
Accurate modeling is important for battery simulation analysis, optimization control, status monitoring, and life management. Due to the complex internal characteristics of batteries, to simplify the modeling problem of batteries, several key model parameters are generally used to replace the complex chemical and physical processes inside the battery. So far, the models of lithium batteries mainly include equivalent circuit models, electrochemical models, and mathematical models. The widely used models are equivalent circuit models and electrochemical models. Common equivalent circuit models include resistor-capacitance (RC) network models, partnership for a new generation of vehicles (PNGV) models, and general nonlinear (GNL) models [11]. Common electrochemical models include single particle model (SPM), pseudo-two-dimensional model (P2D), etc. The electrochemical model starts from the basic principles of electrochemistry to describe the dynamic process between the positive and negative electrodes of lithium batteries. Based on the transfer process of internal charged particles, the model has higher accuracy compared to the equivalent circuit model. However, its disadvantage is that the calculation process is complex and limited to laboratory research. The equivalent circuit model has better universality and stronger scalability and is widely used in lithium batteries research. Identifying model parameters is a prerequisite for model establishment, and the methods of parameter identification are mainly divided into offline methods and online methods [12]. Offline methods mainly include least squares method, impedance spectroscopy method, meta-heuristic algorithm, etc., which largely rely on the test data of the battery under different conditions. Online methods mainly include recursive least squares, Kalman filtering, adaptive observer, etc. The online method can identify the parameters of the model in real time and has an excellent ability to capture parameter changes [13].
At present, some research on battery modeling has been carried out. Reference [14] employed 1st-order RC equivalent circuit model as the research object and used the least squares method to identify the model parameters. The results show that this method has high identification accuracy and low computational complexity. Huang et al. [15] conducted parameter identification research on both 1st-order RC equivalent circuit model and 2nd-order RC equivalent circuit model of batteries, using an improved linear particle swarm optimization algorithm for parameter identification. By comparing the experimental results, it is obvious that this method has fast convergence speed and high accuracy. Furthermore, by comparing and analyzing the two models, it can be concluded that 2nd-order RC equivalent circuit model can better simulate the dynamic characteristics of batteries. Liu et al. [16] systematically reviewed the development of chemical models and parameter identification research for lithium batteries, introduced P2D models and simplified models, and classified parameter identification, summarizing the characteristics of various methods.
It is worth noting that since lithium batteries are currently widely used in practical applications, it is important to study online battery modeling techniques. Some artificial intelligence (AI) methods have also been applied to parameter identification of lithium batteries models, such as meta-heuristic algorithms (MhAs), which can effectively improve the efficiency and accuracy of battery modeling.
2.2 Reliable Operation and Management of Batteries
Batteries continuously generate heat during operation, which can cause an increase in battery temperature and affect performance and cycle life. In severe cases, it can also cause safety accidents such as combustion and even explosions. Therefore, effective heat dissipation technology is crucial for the operation of batteries [17]. In addition, lithium batteries are widely used in energy storage stations, conventional in the form of battery packs. The initial capacity of battery pack in the energy storage station is normally different from each other. If the rate of SOC decrease during discharge is not consistent, the lower SOC will be discharged first, which will reduce the capacity utilization and service life of the energy storage station. Therefore, it is necessary to study the equilibrium strategy of lithium battery SOC, which is extremely important for the operation of the battery. In addition, the internal materials of the battery are also crucial for its performance, and the use of appropriate electrode materials can also help improve the battery’s performance [18].
Battery management is also very necessary, and it cannot be separated from accurate estimation and prediction of the current condition of the battery. Through accurate state estimation, guidance can be provided for subsequent battery management. At present, research on state estimation for lithium batteries mainly includes SOC estimation, state of health estimation, and life cycle assessment (LCA), in which LCA is used to assess the impact of products, production processes, and activities on the environment. In addition, there are also some predictive studies on the remaining life of batteries. Reference [19] reviewed the methods and clearly classified for estimating SOC of lithium batteries, and systematically evaluated and compared the advantages and disadvantages of each method, providing important guidance for subsequent research on SOC estimation of lithium batteries. Yang et al. [20] provide a comprehensive introduction and summary of SOH estimation methods and summarize various parameters that reflect battery aging. The study provides important guidance value for subsequent measurement of battery aging and estimation of battery SOH. Wang et al. [21] conducted estimation research on the remaining useful life (RUL) of lithium batteries and proposed a novel estimation model based on an improved anti-noise adaptive long short-term memory (ANA-LSTM) neural network. The model considers changes in parameters such as current rate and environment and optimizes the model parameters. The research result proved that the model has good accuracy and robustness. Reference [22] proposed a RUL estimation method based on singular filtering-Gaussian process regression-long short-term memory (SF-GPR-LSTM). This method can quickly and accurately achieve estimation tasks, and has advantages such as high robustness and good stability, laying a theoretical foundation for the industrial application of lithium batteries.
Overall, the most widely used state estimation methods currently are data-driven methods, such as neural networks, machine learning algorithms, etc. Compared to other methods, this method has faster estimation accuracy and stronger generalization, but it heavily relies on battery test data [23].
2.3 Accurate Diagnosis of Batteries Systems
Lithium batteries operate under complex and variable operating conditions in practical applications, and the impact of extreme environmental factors can also increase safety hazards and shorten useful life [24]. Therefore, fault diagnosis technology is extremely important for improving the reliability and safety of batteries. Through accurate fault diagnosis, battery faults can be eliminated timely, and then targeted operation and maintenance can be carried out. It can enhance the service life of batteries, and reduce the cost of battery operation and management. Diagnosis of faults cannot be separated from analyzing the triggering mechanism and behavioral characteristics of battery faults [25,26]. The most commonly used diagnosis technology currently is based on AI technology, which uses algorithms to extract features from fault data to identify fault categories.
Reference [27] summarized the research status and achievements of fault diagnosis for lithium-ion battery systems from three aspects: Data fusion, fault recognition, and fault prediction, with the background of big data. It also looks forward to future fault diagnosis technologies based on the fusion of big data and artificial intelligence. Reference [28] classified faults into three categories based on different locations: Internal battery faults, sensor faults, and actuator faults. A comprehensive review of various fault mechanisms, features, and diagnostic methods was conducted, and this work also provides more prospects for future research directions, such as developing and using more data-driven methods and utilizing multiple fault features and diagnostic methods. However, it is worth noting that fault diagnosis techniques based on big data heavily rely on algorithm performance and require high quantity and quality of data samples.
This article comprehensively analyzes the correlative investigation on key issues of modeling, operation, management, and diagnosis of lithium batteries and investigates some current advanced technologies, which can provide valuable guiding significance for future research. Based on the aforementioned key issues and technologies, five constructive prospects are summarized as follows:
• The current modeling technique for lithium batteries chiefly focuses on single battery, but battery appear in the form of battery packs at actual usage. Therefore, it is necessary to conduct more modeling analysis and research on lithium battery packs.
• Due to the nonlinear and time-varying characteristics of lithium batteries, it is essential to develop more online parameter identification techniques, which can provide vital technical support for the management of lithium batteries in practical operation.
• Reasonable operating strategies and reliable battery management techniques can reduce the cost of battery operation. Hence, more operational technologies related to lithium battery systems can be developed from an optimization perspective. Meanwhile, online state estimation technology and joint estimation methods should be developed, which can improve the efficiency of battery management;
• In the context of big data, developing and utilizing more novel data-driven methods (such as machine learning, deep learning, transfer learning, etc.) are of great meaning for solving the above issues, which can significantly improve the ability and efficiency of battery management, and effectively promote the evolution of lithium batteries technology.
• It is indispensable to implement more related studies on lithium batteries (e.g., LCA, SOP, SOE, etc.). Besides, it is vital to conduct more research on the internal mechanisms of lithium batteries to further improve the power supply performance.
Acknowledgement: This research did not receive any specific grant from funding agencies in the public, commercial, or not-for-profit sectors.
Funding Statement: The authors received no specific funding for this study.
Author Contributions: Bo Yang: Writing the original draft and editing. Yucun Qian and Jianzhong Xu: Conceptualization. Yaxing Ren and Yixuan Chen: Contributed to the discussion of the topic. All authors have read and agreed to the published version of the manuscript.
Availability of Data and Materials: No data was used for the research described in the article.
Conflicts of Interest: The authors declare that they have no conflicts of interest to report regarding the present study.
References
1. M. S. H. Lipu, et al., “A review of state of health and remaining useful life estimation methods for lithium-ion battery in electric vehicles: Challenges and recommendations,” J. Clean. Prod., vol. 205, pp. 115–133, Dec. 2018. doi: 10.1016/j.jclepro.2018.09.065. [Google Scholar] [CrossRef]
2. B. Yang et al., “Critical summary and perspectives on state-of-health of lithium-ion battery,” Renew. Sustain. Energ. Rev., vol. 190, pp. 1–28, Feb. 2024. doi: 10.1016/j.rser.2023.114077. [Google Scholar] [CrossRef]
3. United Nations, The Sustainable Development Goals Report, 2020. [Google Scholar]
4. A. Fotouhi, D. J. Auger, K. Propp, S. Longo, and M. Wild, “A review on electric vehicle battery modelling: From lithium-ion toward lithium-sulphur,” Renew. Sustain. Energ. Rev., vol. 56, pp. 1008–1021, Apr. 2016. doi: 10.1016/j.rser.2015.12.009. [Google Scholar] [CrossRef]
5. B. Yang et al., “Optimization and control of new power systems under the dual carbon goals: Key issues, advanced techniques, and perspectives,” Energies, vol. 16, no. 3904, pp. 1–4, May 2023. doi: 10.3390/en16093904. [Google Scholar] [CrossRef]
6. Y. Zhang, J. C. Chen, T. Yao, Y. J. Zhang, Z. H. Liu and C. L. Du, “Energy-aware optimization of connected and automated electric vehicles considering vehicle-traffic nexus,” IEEE Trans. Ind. Electron., vol. 71, no. 1, pp. 282–293, Jan. 2024. doi: 10.1109/TIE.2023.3245204. [Google Scholar] [CrossRef]
7. Q. Xiong, Z. G. Di, and S. C. Ji, “Review on health state estimation and life prediction of lithium-ion batteries,” (in ChineseHigh Voltage Eng., pp. 1–14, Jan. 2023. doi: 10.13336/j.1003-6520.hve.20221843. [Google Scholar] [CrossRef]
8. L. Xu, Z. W. Deng, Y. Xie, and X. S. Hu, “Review on research progress in high-fidelity modeling, parameter identification and lifetime prognostics of lithium-ion battery,” (in ChineseJ. Mech. Eng., vol. 58, no. 22, pp. 19–35, Nov. 2022. doi: 10.3901/JME.2022.22.019. [Google Scholar] [CrossRef]
9. P. R. Iturriaga, D. Ansean, S. R. Bolivar, V. M. Garcia, M. Gonzalez and J. A. L. Villanueva, “Modeling current-rate effects in lithium-ion batteries based on a distributed, multi-particle equivalent circuit model,” Appl. Energy, vol. 353, Jan. 2024. doi: 10.1016/j.apenergy.2023.122141. [Google Scholar] [CrossRef]
10. M. V. Afraz, Z. A. Mohammadi, and G. Karimi, “A novel compact thermal management model for performance evaluation of tesla-like lithium-ion battery packs,” Energy Convers. Manag., vol. 300, Jan. 2024. doi: 10.1016/j.enconman.2023.117927. [Google Scholar] [CrossRef]
11. X. K. Chen and D. Sun, “Research on lithium-ion battery modeling and model parameter identification methods,” (in ChineseProc. of the CSEE, vol. 36, no. 22, pp. 6254–6261, Nov. 2016. doi: 10.13334/j.0258-8013.pcsee.152441. [Google Scholar] [CrossRef]
12. S. Panchal, M. Mathew, R. Fraser, and M. Fowlerb, “Electrochemical thermal modeling and experimental measurements of 18650 cylindrical lithium-ion battery during discharge cycle for an EV,” Appl. Therm. Eng., vol. 135, pp. 123–132, May 2018. doi: 10.1016/j.applthermaleng.2018.02.046. [Google Scholar] [CrossRef]
13. X. S. Hu and X. L. Tang, “Review of modeling techniques for lithium-ion traction batteries in electric vehicles,” (in ChineseJ. Mech. Eng., vol. 53, no. 16, pp. 20–31, Aug. 2017. doi: 10.3901/JME.2017.16.020. [Google Scholar] [CrossRef]
14. R. Zhu, B. Duan, F. Z. Wen, J. M. Zhang, and C. H. Zhang, “Lithium-ion battery modeling and parameter identification based on decentralized least squares method,” (in ChineseJ. Mech. Eng., vol. 55, no. 20, pp. 85–93, Oct. 2019. doi: 10.3901/JME.2019.20.085. [Google Scholar] [CrossRef]
15. K. Huang, Y. F. Guo, and Z. G. Li, “Modeling of power lithium-ion battery behavior considering hysteresis effect,” (in ChinesePower Syst. Technol., vol. 41, no. 8, pp. 2695, Aug. 2017. doi: 10.13335/j.1000-3673.pst.2017.0026. [Google Scholar] [CrossRef]
16. K. L. Liu et al., “Electrochemical modeling and parameterization towards control-oriented management of lithium-ion batteries,” Control Eng. Pract., vol. 124, Jul. 2022. doi: 10.1016/j.conengprac.2022.105176. [Google Scholar] [CrossRef]
17. M. Chen and J. J. Li, “Experiment on heat dissipation performance of electric vehicle lithium battery based on pulsating heat pipe,” (in ChineseChemi. Indust. and Eng. Prog., vol. 40, no. 6, pp. 3163–3171, Jun. 2021. doi: 10.16085/j.issn.1000-6613.2020-1400. [Google Scholar] [CrossRef]
18. S. P. S. Arockia, S. J. Arlyn, S. Padmaja, M. P. Selvi, S. Sinthika and R. N. Elizabeth, “Enhanced electrochemical performance by facilitating li-ion diffusion in LiNi0.1MgxCo0.9-xO2(0 ≤ x ≤ 0.2)-for high energy li-ion batteries,” Nano-Struct. & Nano-Object., vol. 24, Oct. 2020. doi: 10.1016/j.nanoso.2020.100559. [Google Scholar] [CrossRef]
19. B. Yang et al., “Classification, summarization and perspectives on state-of-charge estimation of lithium-ion batteries used in electric vehicles: A critical comprehensive survey,” J. Energy Storage, vol. 39, Jul. 2021. doi: 10.1016/j.est.2021.102572. [Google Scholar] [CrossRef]
20. S. J. Yang, C. P. Zhang, J. C. Jiang, W. G. Zhang, L. J. Zhang and Y. B. Wang, “Review on state-of-health of lithium-ion batteries: Characterizations, estimations and applications,” J. Clean. Prod., vol. 314, Sep. 2021. doi: 10.1016/j.jclepro.2021.128015. [Google Scholar] [CrossRef]
21. S. L. Wang, Y. C. Fan, S. Y. Jin, P. T. Aninakwa, and C. Fernandez, “Improved anti-noise adaptive long short-term memory neural network modeling for the robust remaining useful life prediction of lithium-ion batteries,” Reliab. Eng. Syst. Saf., vol. 230, Oct. 2023. doi: 10.1016/j.ress.2022.108920. [Google Scholar] [CrossRef]
22. S. L. Wang, F. Wu, P. T. Aninakwa, C. Fernandez, D. I. Stroe and Q. Huang, “Improved singular filtering-Gaussian process regression-long short-term memory model for whole-life-cycle remaining capacity estimation of lithium-ion batteries adaptive to fast aging and multi-current variations,” Energy, vol. 284, Aug. 2023. doi: 10.1016/j.energy.2023.128677. [Google Scholar] [CrossRef]
23. M. U. Cuma and T. Koroglu, “A comprehensive review on estimation strategies used in hybrid and battery electric vehicles,” Renew. Sustain. Energ. Rev., vol. 42, pp. 517–531, Feb. 2015. doi: 10.1016/j.rser.2014.10.047. [Google Scholar] [CrossRef]
24. G. J. Ma, S. P. Xu, and C. Cheng, “Fault detection of lithium-ion battery packs with a graph-based method,” J. Energy Storage, vol. 43, Nov. 2021. doi: 10.1016/j.est.2021.103209. [Google Scholar] [CrossRef]
25. R. Xiong, R. X. Yang, Z. Y. Chen, W. X. Shen, and F. C. Sun, “Online fault diagnosis of external short circuit for lithium-ion battery pack,” IEEE Trans. Ind. Electron., vol. 67, no. 2, pp. 1081–1091, Feb. 2020. doi: 10.1109/TIE.2019.2899565. [Google Scholar] [CrossRef]
26. Q. Q. Yu, C. Wang, J. M. Li, R. Xiong, and M. Pecht, “Challenges and outlook for lithium-ion battery fault diagnosis methods from the laboratory to real world applications,” eTransportation, vol. 17, Jul. 2023. doi: 10.1016/j.etran.2023.100254. [Google Scholar] [CrossRef]
27. Z. P. Wang, X. Y. Li, C. G. Yuan, and X. H. Li, “Challenge and prospects for fault diagnosis of power battery system for electrical vehicles based on big-data,” (in ChineseJ. Mech. Eng., vol. 57, no. 14, pp. 52–63, Jul. 2021. doi: 10.3901/JME.2021.14.052. [Google Scholar] [CrossRef]
28. X. S. Hu, K. Zhang, K. L. Liu, X. Lin, S. Dey and S. Onori, “Advanced fault diagnosis for lithium-ion battery systems: A review of fault mechanisms, fault features, and diagnosis procedures,” Ind. Electron. Mag., vol. 14, no. 3, pp. 65–91, Sept. 2020. doi: 10.1109/MIE.2020.2964814. [Google Scholar] [CrossRef]
Cite This Article
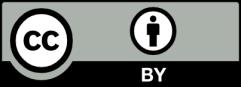
This work is licensed under a Creative Commons Attribution 4.0 International License , which permits unrestricted use, distribution, and reproduction in any medium, provided the original work is properly cited.