Open Access
ARTICLE
Power Quality Disturbance Identification Basing on Adaptive Kalman Filter and Multi-Scale Channel Attention Fusion Convolutional Network
School of Automation and Electrical Engineering, Lanzhou Jiaotong University, Lanzhou, 730070, China
* Corresponding Author: Guangdi Liu. Email:
Energy Engineering 2024, 121(7), 1865-1882. https://doi.org/10.32604/ee.2024.048209
Received 30 November 2023; Accepted 13 March 2024; Issue published 11 June 2024
Abstract
In light of the prevailing issue that the existing convolutional neural network (CNN) power quality disturbance identification method can only extract single-scale features, which leads to a lack of feature information and weak anti-noise performance, a new approach for identifying power quality disturbances based on an adaptive Kalman filter (KF) and multi-scale channel attention (MS-CAM) fused convolutional neural network is suggested. Single and composite-disruption signals are generated through simulation. The adaptive maximum likelihood Kalman filter is employed for noise reduction in the initial disturbance signal, and subsequent integration of multi-scale features into the conventional CNN architecture is conducted. The multi-scale features of the signal are captured by convolution kernels of different sizes so that the model can obtain diverse feature expressions. The attention mechanism (ATT) is introduced to adaptively allocate the extracted features, and the features are fused and selected to obtain the new main features. The Softmax classifier is employed for the classification of power quality disturbances. Finally, by comparing the recognition accuracy of the convolutional neural network (CNN), the model using the attention mechanism, the bidirectional long-term and short-term memory network (MS-Bi-LSTM), and the multi-scale convolutional neural network (MSCNN) with the attention mechanism with the proposed method. The simulation results demonstrate that the proposed method is higher than CNN, MS-Bi-LSTM, and MSCNN, and the overall recognition rate exceeds 99%, and the proposed method has significant classification accuracy and robust classification performance. This achievement provides a new perspective for further exploration in the field of power quality disturbance classification.Keywords
Cite This Article
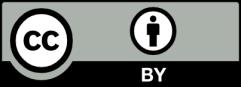
This work is licensed under a Creative Commons Attribution 4.0 International License , which permits unrestricted use, distribution, and reproduction in any medium, provided the original work is properly cited.