Open Access
ARTICLE
Dynamic Economic Scheduling with Self-Adaptive Uncertainty in Distribution Network Based on Deep Reinforcement Learning
1 State Grid Liaoning Electric Power Co., Ltd., Liaoyang Power Supply Company, Liaoyang, 111000, China
2 Key Laboratory of Modern Power System Simulation and Control & Renewable Energy Technology, Ministry of Education (Northeast Electric Power University), Jilin, 132012, China
3 Jilin Northeast Electric Power University Science and Technology Development Co., Ltd., Jilin, 132012, China
* Corresponding Author: Jinling Li. Email:
(This article belongs to the Special Issue: Key Technologies of Renewable Energy Consumption and Optimal Operation under )
Energy Engineering 2024, 121(6), 1671-1695. https://doi.org/10.32604/ee.2024.047794
Received 17 November 2023; Accepted 08 January 2024; Issue published 21 May 2024
Abstract
Traditional optimal scheduling methods are limited to accurate physical models and parameter settings, which are difficult to adapt to the uncertainty of source and load, and there are problems such as the inability to make dynamic decisions continuously. This paper proposed a dynamic economic scheduling method for distribution networks based on deep reinforcement learning. Firstly, the economic scheduling model of the new energy distribution network is established considering the action characteristics of micro-gas turbines, and the dynamic scheduling model based on deep reinforcement learning is constructed for the new energy distribution network system with a high proportion of new energy, and the Markov decision process of the model is defined. Secondly, Second, for the changing characteristics of source-load uncertainty, agents are trained interactively with the distributed network in a data-driven manner. Then, through the proximal policy optimization algorithm, agents adaptively learn the scheduling strategy and realize the dynamic scheduling decision of the new energy distribution network system. Finally, the feasibility and superiority of the proposed method are verified by an improved IEEE 33-node simulation system.Keywords
Cite This Article
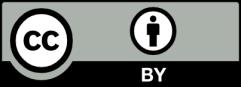
This work is licensed under a Creative Commons Attribution 4.0 International License , which permits unrestricted use, distribution, and reproduction in any medium, provided the original work is properly cited.