Open Access
ARTICLE
Improved Unit Commitment with Accurate Dynamic Scenarios Clustering Based on Multi-Parametric Programming and Benders Decomposition
1 State Grid Corporation of China, Beijing, 100031, China
2 Central China Branch, State Grid Corporation of China, Wuhan, 430077, China
3 School of Electric and Electronic Engineering, Huazhong University of Science and Technology, Wuhan, 430074, China
4 China Electric Power Research Institute Co., Ltd., Beijing, 100192, China
* Corresponding Author: Mingyu Yan. Email:
Energy Engineering 2024, 121(6), 1557-1576. https://doi.org/10.32604/ee.2024.047401
Received 04 November 2023; Accepted 23 January 2024; Issue published 21 May 2024
Abstract
Stochastic unit commitment is one of the most powerful methods to address uncertainty. However, the existing scenario clustering technique for stochastic unit commitment cannot accurately select representative scenarios, which threatens the robustness of stochastic unit commitment and hinders its application. This paper provides a stochastic unit commitment with dynamic scenario clustering based on multi-parametric programming and Benders decomposition. The stochastic unit commitment is solved via the Benders decomposition, which decouples the primal problem into the master problem and two types of subproblems. In the master problem, the committed generator is determined, while the feasibility and optimality of generator output are checked in these two subproblems. Scenarios are dynamically clustered during the subproblem solution process through the multi-parametric programming with respect to the solution of the master problem. In other words, multiple scenarios are clustered into several representative scenarios after the subproblem is solved, and the Benders cut obtained by the representative scenario is generated for the master problem. Different from the conventional stochastic unit commitment, the proposed approach integrates scenario clustering into the Benders decomposition solution process. Such a clustering approach could accurately cluster representative scenarios that have impacts on the unit commitment. The proposed method is tested on a 6-bus system and the modified IEEE 118-bus system. Numerical results illustrate the effectiveness of the proposed method in clustering scenarios. Compared with the conventional clustering method, the proposed method can accurately select representative scenarios while mitigating computational burden, thus guaranteeing the robustness of unit commitment.Keywords
Cite This Article
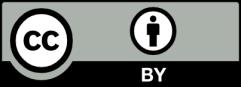
This work is licensed under a Creative Commons Attribution 4.0 International License , which permits unrestricted use, distribution, and reproduction in any medium, provided the original work is properly cited.