Open Access
ARTICLE
Analysis and Modeling of Time Output Characteristics for Distributed Photovoltaic and Energy Storage
1 China Electric Power Research Institute, Beijing, 100192, China
2 Electric Power Research Institute, State Grid Gansu Electric Power Company, Lanzhou, 730000, China
3 State Key Laboratory of Power Grid Safety, Beijing, 100084, China
* Corresponding Author: Kaicheng Liu. Email:
(This article belongs to the Special Issue: Renewable Energy Technologies for Sustainable Energy Production)
Energy Engineering 2024, 121(4), 933-949. https://doi.org/10.32604/ee.2023.043658
Received 08 July 2023; Accepted 11 October 2023; Issue published 26 March 2024
Abstract
Due to the unpredictable output characteristics of distributed photovoltaics, their integration into the grid can lead to voltage fluctuations within the regional power grid. Therefore, the development of spatial-temporal coordination and optimization control methods for distributed photovoltaics and energy storage systems is of utmost importance in various scenarios. This paper approaches the issue from the perspective of spatiotemporal forecasting of distributed photovoltaic (PV) generation and proposes a Temporal Convolutional-Long Short-Term Memory prediction model that combines Temporal Convolutional Networks (TCN) and Long Short-Term Memory (LSTM). To begin with, an analysis of the spatiotemporal distribution patterns of PV generation is conducted, and outlier data is handled using the 3σ rule. Subsequently, a novel approach that combines temporal convolution and LSTM networks is introduced, with TCN extracting spatial features and LSTM capturing temporal features. Finally, a real spatiotemporal dataset from Gansu, China, is established to compare the performance of the proposed network against other models. The results demonstrate that the model presented in this paper exhibits the highest predictive accuracy, with a single-step Mean Absolute Error (MAE) of 1.782 and an average Root Mean Square Error (RMSE) of 3.72 for multi-step predictions.Keywords
Cite This Article
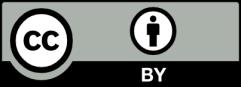
This work is licensed under a Creative Commons Attribution 4.0 International License , which permits unrestricted use, distribution, and reproduction in any medium, provided the original work is properly cited.