Open Access
ARTICLE
Combined Optimal Dispatch of Thermal Power Generators and Energy Storage Considering Thermal Power Deep Peak Clipping and Wind Energy Emission Grading Punishment
1 Key Laboratory of Modern Power System Simulation and Control & Renewable Energy Technology, Northeast Electric Power University, Ministry of Education, Jilin, 132012, China
2 Songyuan Power Supply Company, State Grid Jilin Electric Power Co., Ltd., Songyuan, 138000, China
3 Changchun Power Supply Company, State Grid Jilin Electric Power Co., Ltd., Changchun, 130021, China
4 Jilin Electric Power Co., Ltd., Jilin Songhuajiang Thermal Power Generation Co., Ltd., Jilin, 132012, China
* Corresponding Author: Cuiping Li. Email:
Energy Engineering 2024, 121(4), 869-893. https://doi.org/10.32604/ee.2024.029722
Received 05 March 2023; Accepted 28 June 2023; Issue published 26 March 2024
Abstract
Peak load and wind energy emission pressure rise more as wind energy penetration keeps growing, which affects the stabilization of the PS (power system). This paper suggests integrated optimal dispatching of thermal power generators and BESS (battery energy storage system) taking wind energy emission grading punishment and deep peak clipping into consideration. Firstly, in order to minimize wind abandonment, a hierarchical wind abandonment penalty strategy based on fuzzy control is designed and introduced, and the optimal grid-connected power of wind energy is determined as a result of minimizing the peak cutting cost of the system. Secondly, considering BESS and thermal power, the management approach of BESS-assisted virtual peak clipping of thermal power generators is aimed at reducing the degree of deep peak clipping of thermal power generators and optimizing the output of thermal power generators and the charging and discharging power of BESS. Finally, Give an example of how this strategy has been effective in reducing abandonment rates by 0.66% and 7.46% individually for different wind penetration programs, and the daily average can reduce the peak clipping power output of thermal power generators by 42.97 and 72.31 MWh and enhances the effect and economy of system peak clipping.Graphic Abstract
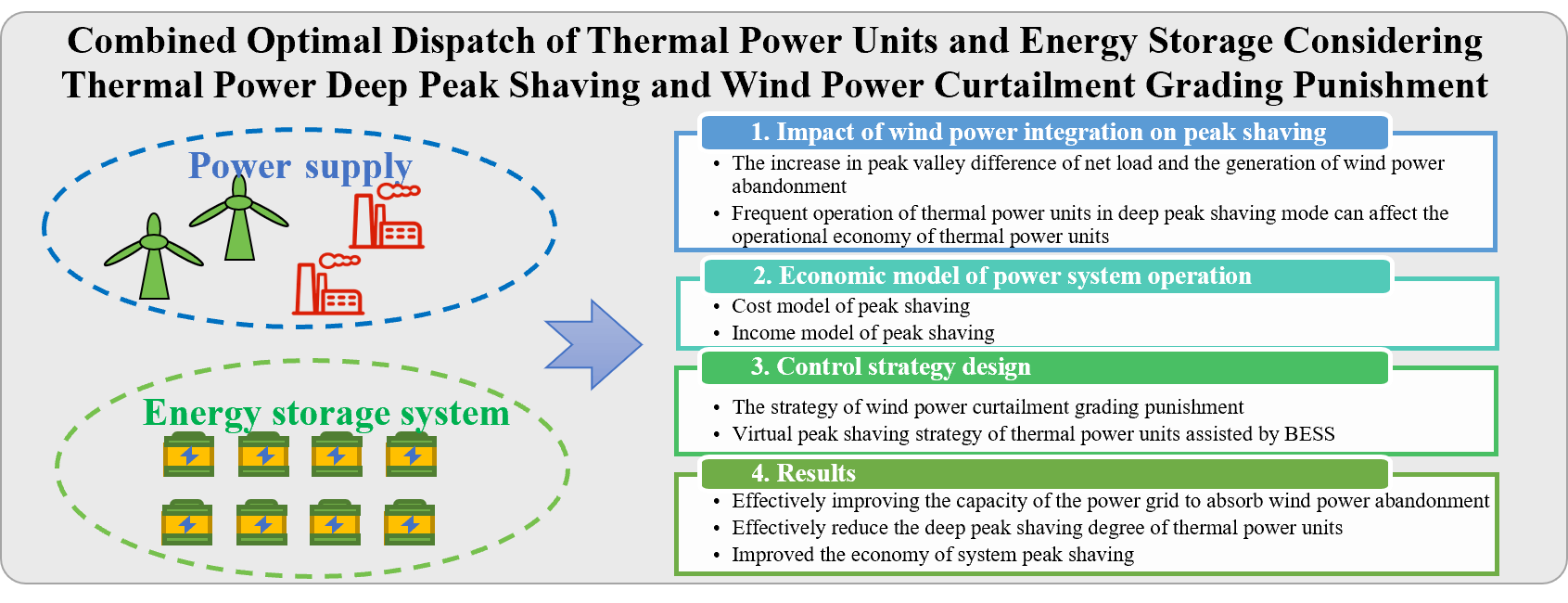
Keywords
The development of new energy is very important in China’s energy strategic layout. By 2020, China’s cumulative installed wind energy capacity is 281 million kilowatts [1]. Due to the randomness and inverse peaking characteristics of wind energy, the increase of wind energy permeability makes the peak-valley difference of load increase sharply, and the emission stresses increase abruptly, which seriously affects the normal operation of the power grid [2,3]. Therefore, peak clipping needs to be addressed for high-penetration wind energy systems. Easing the Peak Clipping Challenge, power grids have launched deep peak clipping of thermal power generators, but deep peak clipping will increase generator operating costs. The balanced relationship between decision economics and peak clipping performance is the key element determining the operation of generators [4,5].
BESS technology is characterized by quick reaction time and two-way regulation due to its own operational characteristics [6]. Currently, the study of BESS participating in peak control strategy has become a hot topic. Gupta et al. [7] have associated a combined system of wind energy generation and energy storage (ES) batteries to alleviate the pressure of peak clipping, aiming at the large-scale penetration of intermittent wind energy generation. Li et al. [8] optimized the combination of BESS and conventional peak clipping due to both technical and economic considerations. Sigrist et al. [9] set up the operating optimum model for centrally operated isolation systems and analyzed the benefits of BESS and evaluated the impact of peak shifting. Li et al. [10] offered a real-time power assignment approach on the basis of BESS to suppress the volatility of new energy. Kobla et al. [11] adopted a two-stage strategy set up by BESS, which enhanced the peak clipping and hill and valley infilling capacity of the power grid and energy quality. Zhu et al. [12] used BESS to smooth the power export in the generation sector, improving peak clipping capability and power regulation quality. Gimelli et al. [13] proposed an optimization control method for BESS-assisted cogeneration systems that provide peak clipping services. Yan et al. [14] proposed the peak regulation BESS control strategy with slack peak regulation bottleneck, which effectively increases the space of wind energy grid connection. Song et al. [15] suggested a home energy management system containing photovoltaic and BESS, which increased the peak-load capability and operation economy of the power grid. Wang et al. [16] proposed a dual time scale BESS peak clipping strategy, which reduces the fluctuation of net load and reduces the peak clipping pressure of the system. To enhance the peak clipping capacity of the PS, Liu et al. [17] conducted joint scheduling between BESS and thermal power generators to promote new energy absorption. Of the above references, most of them use BESS to engage in the peak clipping of the power grid, which is mainly used to suppress the fluctuation of new sources and enhance the peak clipping effect of the PS. However, there is a lack of consideration for BESS to assist in the deep peak clipping of thermal power generators, and there is still a serious problem of life loss of thermal power generators.
In addition, in the present case of the massive integration of wind energy into the grid, how to effectively absorb wind energy emissions has also become a major research issue. In the peak clipping of the joint operation of the thermal power generator and BESS, Chen et al. [18] proposed a thermal power generator and BESS coordination optimization strategy considering demand response and BESS life, with minimum net load as the upper objective to reduce wind abandonment rate. On the basis of the mathematical model of the optimal energy rejection rate, Ye et al. [19] added the wind energy absorption cost to enhance the wind energy absorption. Zhao et al. [20–22] enhanced the absorption of wind energy in the combined peaking of thermal power generators and BESS, introducing wind abandonment penalty cost into the objective function. Zhang et al. [23] addressed the problem of severe wind abandonment, designed a multi-objective strategy with minimum wind abandonment and minimum operating costs, and included the wind abandonment cost in total operating cost in the combined peak clipping strategy of thermal power generators and BESS in the above references. Considering the problem of wind energy absorption, the penalty cost model of wind energy abandonment is usually added to the model to enhance wind energy absorption. Nevertheless, the penalty cost model of wind energy emission is only multiplied by the amount of wind energy emission with a constant penalty factor. The wind energy emission grading punishment strategy proposed in this article is on the basis of fuzzy rules, comprehensively considering the load demand and the wind energy generating capability, and jointly determining the penalty gear factor of wind energy emission. The constraint on wind energy emission is strengthened, and the absorption is increased.
In summary, in response to the issue that frequent deep peak clipping of thermal power generators can affect their lifespan, a BESS-assisted deep peak clipping strategy has been proposed to reduce the degree of deep peak clipping of thermal power generators and enhance overall economy; In response to the current wind energy emission constraint that only uses the product of a constant penalty factor and wind energy output as the cost of wind abandonment punishment, a wind energy emission grading punishment strategy based on fuzzy rules and comprehensive consideration of load and wind energy throughput is proposed to better eliminate wind energy emission and enhance the competitiveness of wind turbine generators.
Section 2 of this paper first explores the impact of grid-connected high infiltration wind energy on peak shaving of the PS. and then establishes the economic model of PS operation in Section 3. On this basis, combined optimal dispatching of thermal power generators and BESS considering deep peak clipping and wind emission grading punishment is proposed in Section 4. In order to reduce wind abandonment effectively, a graded punishment strategy for wind abandonment is proposed. To enhance the operation economy of the PS, reduce the peak clipping degree of thermal power generators, and prolong their life cycle, the BESS and thermal power generators are regarded as the whole of peak clipping, and the BESS-assisted virtual peak clipping control strategy of thermal power generators is designed. Finally, Section 5 of the paper provides an example of the effectiveness of this strategy.
2 Effects of Wind Infiltration on Peak Load Regulation
The PS with high penetration wind energy includes thermal power plant, wind energy plant, BESS, power load, etc. The primary structure of PS is shown in Fig. 1.
Figure 1: Primary structure of PS
Before the wind energy is joined to the grid, the load power demand PloadPload of the grid is all supplied by the thermal power PGPG, namely:
PG=PloadPG=Pload(1)
After the wind energy is joined to the grid, the grid load power demand PloadPload is jointly supplied by the thermal power PGPG and the wind energy PwindPwind, namely:
PG+Pwind=PloadPG+Pwind=Pload(2)
In order to increase the utilization of clean energy, PS uses thermal power to supply residual load demand after wind energy, which is called the equivalent load PloadeqPloadeq, namely:
PG=Ploadeq=Pload−PwindPG=Ploadeq=Pload−Pwind(3)
However, due to the obvious reverse peaking characteristics of wind energy, as wind penetration increases, this will make the peak regulation of the PS difficult. The schematic diagram of peak clipping of PS is shown in Fig. 2.
Figure 2: Peak clipping diagram of PS
Wind power and load fluctuate in opposite trends, that is, wind energy tends to be lower when load demand is high and higher when load demand is low. From Fig. 2, it can be seen that the peak-to-valley difference of the grid equivalent load increases after the wind energy PwindPwind is connected to the grid from the original PC1PC1 to PC2PC2. In this way, during low load periods, thermal power generators need deep peak clipping operation. If the thermal power generators reach the lower limit of deep peak clipping output PbPb cannot meet the peak clipping needs, energy emission will occur, and with the increase of wind energy permeability, this phenomenon will be more obvious.
BESS has the characteristics of power huff and puff. After auxiliary operation of thermal power generators, the peak clipping degree of thermal power generators is reduced from Pd1Pd1 to Pd2Pd2, to alleviate the peak clipping pressure of thermal power generators, namely:
PG+PBESS=PloadeqPG+PBESS=Ploadeq(4)
where, PBESSPBESS is BESS charge and discharge power.
A well-designed control strategy not just effectively consumes wind energy, but also reduces the peak depth of thermal power generators, improve the initiative of thermal power companies to involve in peaking and enhance the economics of system peaking.
3 Economic Model of PS Operation
The economic modelling of the PS in the peak clipping process is composed of a cost model and a revenue model. This model formula is enhanced and perfected based on references [24,25]. The economic models of each part are established as follows.
3.1 Cost Model of Peak Clipping
(1) Cost model of peak clipping for thermal power generators
The cost of peak clipping for thermal generators is illustrated in Fig. 3. It can be observed from Fig. 3 that this is a piecewise function model. In the normal peaking phase, the peaking cost is the cost per generator of operating coal consumption, and it can be represented as the result of multiplying the amount of coal consumed by the price per unit of coal generator, as illustrated in the figure below:
Figure 3: Cost of thermal power generator during peak load regulation
Ccoal,i,t(PG,i,t)=(aiP2G,i,t+biPG,i,t+ci)pcoalCcoal,i,t(PG,i,t)=(aiP2G,i,t+biPG,i,t+ci)pcoal(5)
where, PG,i,tPG,i,t are the output of the ii thermal power generator at time tt; aiai, bibi, cici are the factors of the absorptive performance function of the ii generator.
When the thermal power generator produces power above its minimum technical output and operates during the deep peaking phase, the deep peaking cost includes not just the coal consumption of the generator, but also the additional life loss cost of the generator and the oil injection cost, which need to be taken into account in the cost calculation. The generator life loss cost is calculated in the following way:
Closs,i,t=12αCcost,i/NG,i,tCloss,i,t=12αCcost,i/NG,i,t(6)
where, αα is the actual operating depletion factor of the coal-fired power plant; Ccost,iCcost,i is the cost of purchasing the ii coal-fired power generator; NG,i,tNG,i,t is the number of rotor fracture cycles of the ii generator at time tt, which is relevant to PG,i,tPG,i,t of the thermal power generators.
When the thermal generator is in oil-filled deep peaking condition, its oil input cost is calculated as follows:
Coil,i,t=toil,iQoil,ipoilCoil,i,t=toil,iQoil,ipoil(7)
where, toil,itoil,i is the duration of peak clipping of the ii thermal power generator; Qoil,iQoil,i is the fuel absorption per generator time of the ii coal-fired power generator; poilpoil is current oil price.
In conclusion, the peaking cost model of the ii thermal power generator can be written as follows:
CG,i,t(PG,i,t)={Ccoal,i,t(PG,i,t)PGmin≤PG,i,t≤PGmaxCcoal,i,t(PG,i,t)+Closs,i,tPa≤PG,i,t<PGminCcoal,i,t(PG,i,t)+Closs,i,t+Coil,i,tPb≤PG,i,t<Pa(8)
where, PGmax and PGmin individually represent the maximum and minimum thresholds of the conventional peak clipping output of thermal power generators; Pa and Pb represent the minimum limit of peak regulating output of deep peak regulating second stage thermal power generator and the lowest value of stable combustion output of third stage thermal power generator individually.
(2) Cost model of wind energy emission grading punishment
After introducing wind energy emission grading punishment gear factor, the punishment cost of emission due to the system’s peak clipping capacity limitation and unable to accept all the wind energy that is able to be generated is written as following:
Cw,t=(1+k⋅θt)pwind(Pwr,t−Pwa,t)ΔT(9)
where, θt is wind energy emission grading punishment gear factor; k is the motion range between adjacent gears (set according to the actual application); pwind is the penalty generator price of wind energy abandonment in PS; Pwr,t and Pwa,t represent wind power and grid-connected wind power individually; ΔT represents the time interval.
(3) Cost model of BESS operating
The ES system incur some operating cost when they are in operation, which is calculated as follows:
CBESS,t=Pprice,M⋅(PC,t+PD,t)ΔT(10)
where, Pprice,M is the generator price of ES system operating cost; PC,t is the charging power of the ES battery at time t; PD,t is the discharge power of the ES battery at time t.
(1) Peaking compensation modelling for thermal power generators
Thermal power producers’ participation in grid peaking can be categorised into basic peaking and paid peaking. Basic peaking refers to the output regulation of thermal power generators in the PGmin to PGmax range. Deep peaking refers to the output regulation of thermal power generators exceeds the conventional free peak load reduction limit, and the compensation can be obtained by participating in the grid peak load reduction under this operating state, which is mainly related to the generation capacity lower than the basic peak load reduction. Peaking compensation income is calculated as following:
Icom,i,t(PG,i,t)={0PGmin≤PG,i,t≤PGmax(PGmin−PG,i,t)Δt⋅pcom1Pa≤PG,i,t<PGmin(PGmin−Pa)Δt⋅pcom1+(Pa−PG,i,t)Δt⋅pcom2Pb≤PG,i,t<Pa(11)
where, pcom1 and pcom2 are the compensation quotations applied by thermal power plants to the power grid individually when no oil is put in and deep peak clipping is put in.
The pricing mechanism for real-time deep peak clipping in the Northeast is shown in Table 1.
(2) Income model of BESS electricity
BESS charges when electricity prices are low and discharges when prices are high to generate revenue. The electricity income is earned through the operation mode of ‘low storage and high generation’.
IBESS,t=(kc∑t=1PC,tΔT)phigh−(kd∑t=1PD,tΔT)plow(12)
where, phigh and plow are individually the peak price and valley price of the system; kc and kd are individually charging and discharging periods in one day.
If the power in BESS remains the same at the beginning of each day, that is, all the power charged in one day is discharged, the above formula can be arranged as follows:
IBESS,t=kc∑t=1PC,tΔT⋅(phighηcηdc−plow)(13)
where, ηc and ηdc are the charging and discharging efficiency of BESS individually.
(3) Income model of BESS environment
BESS operation can decrease the output of thermal power generators, thereby decreasing the emission of pollutant gases generated by coal burning, and has certain environmental income, the proceeds are as following:
Igas,t=Q∑Q=1[ηdc(PD,tΔT)ρQPprice,Q](14)
where, Q is the number of pollutants discharged by the generator set; ρQ is the emission density of the Q-th pollutant that generates a generator of electric energy; Pprice,Q is the generator emission cost of the Q-th pollutant.
(4) Income model of wind energy increasing
Given the combined effect of the wind emission rating penalty strategy and the BESS in the system, it will increase revenues from grid-connected wind energy generation, the proceeds are as following:
Iwind,t=pprice,t(Pwa,t−P0wa,t)ΔT(15)
where, P0wa,t is the original On-grid wind power generation; Pwa,t is the On-grid wind power generation after considering the punishment of wind energy emission; pprice,t is the grid-connected price of wind energy.
In order to effectively reduce wind emission, enhance the depth of thermal generator peaking, and enhance the economy of PS peaking, the joint optimal scheduling strategy of thermal power generator and BESS is designed considering the depth peaking and the classification penalty of wind energy wind emission.
The frame structure of the combined optimal dispatch of thermal power generators and BESS considering deep peaking and wind energy emission grading punishment is shown in Fig. 4.
Figure 4: Framework of combined optimal dispatch of thermal power generators and BESS
First of all, taking the minimum peak load cost as the goal, considering the power equation constraint of the PS and the operational constraint of the thermal power generator, the output Pgd of each thermal power generator and the optimal grid-connected power Pwa of wind energy are preliminarily determined, so as to achieve the effect of absorbing the grid wind abandonment.
Then, it considers the operation constraints of BESS and the thermal power generators, to enhance the efficiency using the characteristics of BESS to handle electric energy with the operation of thermal power generators, the goal of virtual peak clipping of thermal power generators can be achieved, more compensation benefits of deep peak clipping are obtained, to enhance the overall economy of peak clipping of PS.
4.2.1 The Strategy of Wind Energy Emission Grading Punishment
To absorb the wind energy in the system more effectively, a hierarchical punishment strategy for wind curation is proposed. Based on the fuzzy rule and considering the load demand and wind energy capacity, the graded penalty factor θ is determined. The fuzzy reasoning model of the penalty gear factor θ for wind energy emission classification is shown in Fig. 5.
Figure 5: Fuzzy inference model of wind energy emission grading punishment gear factor θ
The value rule of wind energy emission grading punishment gear factor θ is given: depending on the actual load conditions, the marginal power of high, medium and low loads is determined, and load requirements are categorised into high, medium and low loads. Similarly, depending on the actual situation of wind energy generation, the marginal power of high, medium and low loads is determined, and the wind energy generation can be split into high wind energy, medium wind energy and low wind energy, When load demand is high but wind capacity is low, wind energy should be captured to the fullest extent possible. In this case, the emission penalty for wind energy is supposed to be maximum, that is, the gear factor θ is the highest gear. When load demand is small and the wind energy generation capacity is big, to admit wind energy as possible, due to the constraints of real-time power balance of the system, a certain amount of wind emission will inevitably occur. In this case, the wind energy emission punishment should be the minimum, that is, the gear factor θ is the lowest gear. The fuzzy rules are presented as Table 2.
According to the fuzzy rules in Table 2, the punishment factor θ of wind energy emission grading is determined. Considering the above economic models, such as the punishment cost of wind energy emission grading, the peaking cost of thermal power generators, the cost of BESS operating, the peak clipping compensation income of thermal power generators, the electricity income of BESS, environment income of BESS, revenue from grid-connected wind energy and so on, and targeting the minimum peak clipping cost of the system, as shown in the following formula, the output of each thermal power generator and the optimal grid-connected power of wind energy are determined by iterative method.
minF=∑t=1∑i=1CG,i,t+∑t=1Cw,t+∑t=1CBESS,t−(∑t=1∑i=1Icom,i,t+∑t=1IBESS,t+∑t=1Igas,t+∑t=1Iwind,t)(16)
4.2.2 BESS-Assisted Virtual Peak Clipping Strategy for Thermal Generators
This method mainly uses the characteristics of BESS and huff and puff to cooperate with thermal power generators, which not only reduce the degree and persistence of deep peak clipping of thermal power generators, but also earn peak clipping compensation income. The output rules are as follows.
The output curve Pgd of deep peaking generators obtained on the optimization in this grading punishment strategy for wind energy emission is analyzed, and the deep peaking power ED of generators within one day is calculated.
Pdeep,t={PGmin−Pgd,t(Pgd,t<PGmin)0(Pgd,t≥PGmin)(17)
ED=∑t=1Pdeep,tΔT(18)
where, Pdeep is the deep peaking power. BESS is used to cut the peak and fill the valley of Pgd, and BESS’s charge and discharge power PBESS is calculated.Emax=Em⋅SOCmax−E0(19)
PBESS,t={Pb1−Pgd,t(Pgd,t<Pb1)0(other)Pb2−Pgd,t(Pb2<Pgd,t)(20)
Let Ef be the virtual peak clipping power and Ey be the remaining capacity space of BESS, which can be represented as shown in the following formula:
Ef=EmaxEy=0}(ED>Emax)Ef=EDEy=Emax−ED}(ED≤Emax)(21)
It can earn additional virtual peak clipping compensation income IBESScom IBESScom and BESS ‘low storage and high generation’s operation power income IBESS,t.
For different loads and wind energy conditions, there are three cases of deep peaking power ED of generators:
1) When Emax > ED > 0, it indicates that the generator is in a deep peaking phase. At this time, part of the output of BESS is employed for the purpose of assisting the virtual peaking of thermal power generators. The virtual peaking power Ef = ED and the remaining space capacity Ey = Emax − ED are used for ‘low storage and high generation’ peak clipping.
2) When ED > Emax, the full capacity of BESS is used to assist the virtual peak clipping of the generator, and the virtual peak clipping capacity is Ef = Emax.
3) When ED < 0, that is, the generator does not carry out deep peak clipping, the virtual peaking capacity is Ef = 0, and its full capacity space of BESS is used for ‘low storage and high generation’ peak clipping.
Taking the first case as an example, the virtual peak clipping diagram of the BESS auxiliary generator during periods of low load is shown in Fig. 6. Before peak clipping, the generator output is Pgd, and the deep peak clipping power is ΔPQ. After peaking, the generator output is Pgdf = PBESS + Pgd, and the deep peaking energy is 0.
Figure 6: Deep peaking of thermal power generators assisted by BESS during periods of low load
The conventional constraints in the peaking process are as followed:
1) Power balance constraints
∑i=1PG,i+Pwa−PL=Pload(22)
PL=ρ(∑i=1PG,i+Pwa)(23)
ρ=AR×10−3120U2av⋅1f+AR×10−330U2avcos2(φ)+ΔpA(24)
where, Pload is the load demand; PL is the total transmitted loss of the system; ρ is the line loss ratio, which is related with the system load factor [26]; f is the load factor; A is the total power absorption; R is the resistance of the power grid element; Uav is the line voltage converted to a certain voltage level; cos(φ) is the power factor; Δp is the daily fixed power absorption.
2) Operational limitations of thermal power plants
Generator output constraints:
Pmax,i≥PG,i,t≥Pmin,i(25)
where, Pmax,i and Pmin,i are the biggest and smallest output of conventional peaking generator. i individually.
Generator reserve capacity constraints:
∑i=1Pmax,i−Pload≥Ps(26)
where, Ps is the total reserved capability of the generator in the system.
Generator standby ramp rate constraint:
Pup,i≥PG,i,t+1−PG,i,t≥Pdown,i(27)
where, Pup,i and Pdown,i are the maximum upward and downward climbing individually.
3) Operation constraints of BESS
BESS’s power, Electricity, SOC constraints:
Pm≥PBESS,t≥−Pm(28)
Emax≥Ec≥Ed(29)
SOCmax≥SOC≥SOCmin(30)
where, Pm is the rating of BESS; Em, Ec and Ed the nominal capacity, charging capacity and discharging capacity of BESS individually; SOCmax and SOCmin are the limits for BESS of SOC.
This article takes the minimum system peak clipping cost as the object to optimize the power of each thermal power generator, wind turbine generator, and BESS. On this method of solving the optimum goal, based on the MATLAB 2018b platform and using the traversal method for iterative solution.
Firstly, input initial data and determine the wind energy emission grading punishment gear factor at each time based on fuzzy rules; Then, under the operation constraints of thermal power generators, load balancing cost is calculated according to all possible output conditions, and the output f each generator is determined when the total cost of system peak load balancing is lowest. Finally, using the benefit of BESS auxiliary thermal power generator peaking depth, the cost of peak regulation is corrected. The specific solution process of the joint optimal scheduling model of thermal power generators and BESS considering the depth peak load balancing and the classification penalty of wind abandonment is shown in Fig. 7.
Figure 7: Solving process of combined optimal dispatch of thermal power generators and BESS considering deep peaking and wind energy emission grading punishment
1) Thermal power generator
This article analyses and demonstrates the case of a local power grid in Northeast China, which has an instructed thermal PS with an installed capacity of 3700 MW. The generator composition and specifications are listed as Table 3.
The boiler fuel of thermal power generator is coking coal, and the price is 685 yuan/t; The punishment cost of wind energy emission is 0.35 yuan/(kWh).
Two 600 MW generators can conduct deep peaking, and the relevant operating parameters of deep peaking are as follows: The oil absorption of the generator in the deep-peak clipping phase is 4.8 t/h, and current oil price is 6130 yuan/T; The operating loss factors of the generator in the deep-peak clipping phase without oil input and oil input depth are 1.2 and 1.5 individually.
2) ES system
In the calculation example, lithium iron phosphate battery with large-scale application is selected as the ES type. The generator price of power is 1500 yuan/kW, and the generator price of capability is 2500/(kWh). Two 600 MW generators capable of deep peak clipping are equipped with a set of 20 MW/100 MWh BESS, and its specific parameters are illustrated in Table 4.
BESS’s electricity revenue is realized through time-of-use electricity prices in the region, which are illustrated in Table 5 for each period of time.
In addition, the BESS obtains environmental income by reducing thermal power output. The generator emission density and generator emission cost of pollutants of thermal power generators are illustrated in Table 6.
3) Simulation data
The maximum and minimum load values in this area are 3168.1 and 823.3 MW individually, Now, the penetration rate of wind energy in the region is 33.07%, and the installed capacity is 1048 MW. The load and wind profiles are shown at Fig. 8.
Figure 8: Load and wind energy curve
5.2 Peak Clipping Scenario and Scheme Design
For the purpose of validating the rationality and effectiveness of the strategy suggested in this article, two wind energy infiltration programs and four peak load balancing scenarios are designed for comparative analysis, and the absorption level of economic and clean energy operation under different scenarios is analyzed, as shown below:
1) Peak clipping scenario:
Scenario I: The wind energy infiltration is 33.07%, which is the current penetration rate of wind energy in the region.
Scenario II: The wind energy infiltration is 40%.
2) Peak clipping scheme:
Scheme I: Do not consider wind energy emission grading punishment and do not include BESS.
Scheme II: Consider wind energy emission grading punishment and not including BESS.
Scheme III: Do not consider wind energy emission grading punishment, and BESS is shaving the peak and filling the valley on the grid side.
Scheme IV: Consider wind energy emission grading punishment, and virtual peak clipping strategy of BESS-assisted thermal generators, which is the strategy suggested in this paper.
5.3 Analysis of Peak Clipping Results under Two Wind Energy Infiltration
For the purpose of validating the rationality of the strategy suggested in this article, based on the example data in Section 5.1, the peaking scheme in this paper is used for modelling the power grid peaking considering two wind energy encroachment scenarios.
The marginal power of high, medium and low load is determined as 2800 and 2600 MW, the marginal power of high, medium and low load wind energy in Scenario I and Scenario II is determined as 700,400 and 800,500 MW individually. According to the value rule of wind energy emission grading punishment strategy, the grading punishment factor θ of wind energy emission under the two wind energy infiltration programs are shown in Appendix A and Appendix B individually.
The power accumulation diagram and BESS action of various types of generators and wind energy grid connected under Scenario I are shown in Figs. 9 and 10. The power accumulation diagram and BESS action of various types of generators and wind energy grid connected under Scenario II are shown in Figs. 11 and 12.
Figure 9: Output of various types of generators and wind energy grid-connected power accumulation diagram in Scenario I
Figure 10: BESS operation in Scenario I
Figure 11: Output of various types of generators and wind energy grid-connected power accumulation diagram in Scenario II
Figure 12: BESS operation in Scenario II
From Figs. 9 and 11, it is easy to see that the control strategy as proposed in this article can achieve the balance between supply and demand. and demand in each period of the PS by setting reasonable load and high and low marginal power of wind energy under two programs, and through the combined action of wind energy emission grading punishment strategy and BESS-assisted virtual peak clipping for thermal generators, the load supply and demand balance of PS can be realized at all times, and peak adjustment task can be completed based on satisfying the respective operation constraints.
From Figs. 10 and 12, the control strategy can ensure that SOC is in good condition.
In summary, under the two scenarios of wind energy penetration, the BESS can well assist the thermal power generator in virtual peak clipping, and the peak clipping effect is more obvious when the wind energy penetration is 40%.
5.4 Analysis of Operation Results of Various Peak Clipping Schemes
In addition, this paper also carries on the simulation analysis of four peak clipping schemes under two wind energy penetration scenarios.
(1) Analysis of wind energy emission
The wind energy emission after peak clipping under two wind energy penetration scenarios with four peak clipping schemes is shown in Figs. 13 and 14 individually.
Figure 13: The wind energy emission after peak clipping of four schemes in Scenario I
Figure 14: The wind energy emission after peak clipping of four schemes in Scenario II
It can be seen from Figs. 13 and 14 that for two different scenarios of wind energy penetration, relative to Scheme III, Scheme II and Scheme IV are adopted for PS peak clipping, which significantly enhances the absorption of wind energy emission in the system.
Moreover, the wind energy emission in the power grid often occurs in the valley loading periods of the day. At this time, the load demand is low, and wind energy is high. Due to the action of the wind energy emission grading punishment strategy, the wind energy emission grading punishment gear factor is relatively high, which enhances the initiative of deep peaking, thereby improving wind energy emission absorption.
After calculation, the wind energy emission rate is reduced by 0.66% when it is used for the wind energy penetration grid in Scenario I, and by 7.46% when it is applied to the wind energy penetration grid in Scenario II. As wind energy infiltration increases, control strategies can potentially enhance the wind energy absorption capacity.
(2) Output of deep peaking generator
The active power output of the deep peak regulating generator after peak regulation in two wind penetration scenarios is shown in Figs. 15 and 16 individually by adopting four peak regulation schemes.
Figure 15: Active power output of deep peak clipping generator in Scenario I
Figure 16: Active power output of deep peak clipping generator in Scenario II
As can be seen from Figs. 15 and 16, due to the role of emission penalty strategy, the deep peak regulating generator of Scheme II and Scheme IV works in the peak regulation state of deep oil injection during the valley load period. Furthermore, due to the role of the virtual peaking strategy, the peak adjustment depth of the deep peak regulating generator in Scheme IV is significantly lower than that of Scheme II.
The active power output continuous curve of the deep peak regulating generator after peak adjustment in two wind energy scenarios is shown in Figs. 17 and 18 individually.
Figure 17: Output duration curve of deep peak clipping generator in Scenario I
Figure 18: Output duration curve of deep peak clipping generator in Scenario II
As we can see from Figs. 17 and 18 that this strategy suggested in this article can decrease the degree of deep peak clipping of thermal power generators under both scenarios.
Based on the calculation, the first scheme can reduce the average daily peak-load generation of thermal power generators by 42.97 MW, and the second scheme can reduce the average daily peak-load generation of thermal power generators by 72.31 MW. This control strategy can efficiently enhance the depth of peaking, reduce generator loss and prolong generator service life.
(3) Economic analysis of peak clipping (generator: 10000 yuan)
Tables 7 and 8 show the daily average cost of the two scenarios after peak clipping with four peak clipping schemes.
From Table 7, after the strategy of grading and punishing wind energy emissions is implemented, the wind energy emission punishment price increases with the gearing factor, resulting in the increase of wind energy emission punishment cost in Scheme II and Scheme IV, but the income of wind energy grid-connected power increases. Increase in thermal generation depth increases the cost of thermal generation depth. Due to the virtual peak clipping control strategy of BESS, the peaking cost of thermal power generators in Scheme IV is lower compared to Scheme II, and compensation revenue from deep peaking is also lower than that in Scheme II.
From Table 8, due to the high wind energy infiltration of the system, the most serious wind energy emission in Scheme I results into the highest punishment cost of wind energy emission. In Scheme IV, the wind energy emission classification penalty strategy not only greatly reduces the wind energy emission rate, but also enhances the enthusiasm of wind energy grid connection. Deep peaking of thermal power generators not only increases costs, but also obtains a certain peak clipping compensation income, and BESS assists its virtual peak clipping, which not only obtains the income of BESS operation, but also alleviates the degree of deep peaking of thermal power generators.
After calculation, the strategy in this paper can reduce the daily average system peak clipping cost by 0.064% in Scenario I and 1.58% in Scenario II.
In the context of the overall peaking economy of PS, the strategy raised can efficiently eliminate the wind energy emission and enhance the system economy, and the more obvious the effect is to enhance the system economy as wind energy infiltration increases.
For many problems brought by high penetration wind energy grid connection to peak clipping, A strategy is presented in this article of combined optimal dispatch of thermal power generators and BESS considering deep peaking and wind energy emission grading punishment. The simulation results can be concluded as follows:
(1) The strategy of wind energy emission grading punishment based on the fuzzy principle can reduce the emission rate by 0.66% in Scenario I, and 7.46% in Scenario II. The strategy can efficiently enhance the capacity of wind energy dissipation in the power grid.
(2) The proposed virtual peak clipping control strategy can reduce the daily average peak clipping power generation of thermal power generators by 42.97 MWh in Scenario I, and 72.31 MWh in Scenario II. The proposed control strategy can effectively reduce the deep peaking degree of thermal power generators, reduce the generator loss and prolong its service life.
(3) The proposed strategy in this article decreases the daily average system peak clipping cost by 0.064% in Scenario I and 1.58% in Scenario II. This strategy can not just valid y eliminate the wind energy emission, but also enhance the economy of system peak clipping. It performs well in both peak clipping technology and economy.
Acknowledgement: The authors acknowledge the reviewers for providing valuable comments and helpful suggestions to enhance the manuscript.
Funding Statement: This work was supported by Jilin Province Higher Education Teaching Reform Research Project in 2021 (JLJY202186163419).
Author Contributions: The authors confirm their contribution to the paper as follows: Study conception and design: Junhui Li, Xuanzhong Luo, Changxing Ge and Cuiping Li. Data collection: Junhui Li, Xuanzhong Luo and Cuiping Li. Analysis and interpretation of results: Cuiping Li and Changrong Wang. Draft manuscript preparation: Xuanzhong Luo and Changrong Wang. All authors reviewed the results and approved the final version of the manuscript.
Availability of Data and Materials: Data supporting this study are included within the article.
Conflicts of Interest: The authors declare that they have no conflicts of interest to report regarding the present study.
References
1. National Energy Administration (2021). Transcript of online press conference of national energy administration in the first quarter of 2021. http://www.nea.gov.cn/2021-01/30/c_139708580.htm (accessed on 25/11/2023). [Google Scholar]
2. Sun, W. Q., Luo, J., Zhang, J. (2021). Energy storage capacity allocation and influence factor analysis of a power system with a high proportion of wind energy. Power System Protection and Control, 49(15), 9–18. [Google Scholar]
3. Olawumi, T. O., Chan D. W., M. (2018). A scientometric review of global research on sustainability and sustainable development. Journal of Cleaner Production, 183, 231–250. [Google Scholar]
4. Wang, J. J., Zhang, S., Huo, J. K., Zhou, Y., Li, L. et al. (2021). Dispatch optimization of thermal power generator flexibility transformation under the deep peak clipping demand based on invasive weed optimization. Journal of Cleaner Production, 315, 128047. [Google Scholar]
5. Yang, Y. P., Qin, C., Zeng, Y., Wang, C. S. (2019). Interval optimization-based generator commitment for deep peak regulation of thermal generators. Energies, 12(5), 922. [Google Scholar]
6. Hu, Y., Armada, M., Sanchez, M. J. (2022). Potential utilization of battery energy storage systems (BESS) in the major European electricity markets. Applied Energy, 322, 119512. [Google Scholar]
7. Gupta, P. P., Jain, P., Kalkhambkar, V., Sharma, K. C., Bhakar, R. (2020). Stochastic security constrained generator commitment with battery energy storage and wind energy integration. International Transactions on Electrical Energy Systems, 30(10), e12556. [Google Scholar]
8. Li, J. H., Wang, S. (2017). Optimal combined peak-shaving scheme using energy storage for auxiliary considering both technology and economy. Automation of Electric Power Systems, 41(9), 44–50+150 (In Chinese). [Google Scholar]
9. Sigrist, L., Lobato, E., Rouco, L. (2013). Energy storage systems providing primary reserve and peak clipping in small isolated power systems: An economic assessment. International Journal of Electrical Power & Energy Systems, 53(12), 675–683. [Google Scholar]
10. Li, X. J., Yao, L. Z., Hui, D. (2016). Optimal control and management of a large-scale battery energy storage system to mitigate fluctuation and intermittence of renewable generations. Journal of Modern Power Systems and Clean Energy, 4(4), 593–603. [Google Scholar]
11. Kobla, T. R., Jonghoon, K. (2022). Binary-phase service battery energy storage system strategy for peak demand shaving and enhanced power quality. Sustainable Energy Technologies and Assessments, 52, 102328. [Google Scholar]
12. Zhu, J. Z., Cui, X. B., Ni, W. D. (2022). Model predictive control based control strategy for battery energy storage system integrated power plant meeting deep load peak clipping demand. Journal of Energy Storage, 46, 103811. [Google Scholar]
13. Gimelli, A., Mottola, F., Muccillo, M., Proto, D., Amoresano, A. et al. (2019). Optimal configuration of modular cogeneration plants integrated by a battery energy storage system providing peak clipping service. Applied Energy, 242, 974–993. [Google Scholar]
14. Yan, G. G., Feng, X. D., Li, J. H., Mu, G., Xie, G. Q. et al. (2012). Optimization of energy storage system capacity for relaxing peak load regulation bottlenecks. Proceedings of the CSEE, 32(28), 27–35 (In Chinese). [Google Scholar]
15. Song, Z. Y., Guan, X., Cheng, M. (2022). Multi-objective optimization strategy for home energy management system including PV and battery energy storage. Energy Reports, 8, 5396–5411. [Google Scholar]
16. Wang, X. D., Zhang, Y. L., Liu, Y. M., Miao, Y. Z. (2021). Double time scale power prediction based peaking shaving control strategy of energy storage system. Acta Energiae Solaris Sinica, 42(7), 58–64. [Google Scholar]
17. Liu, C., Sun, A., Wang, Y. B., He, H., Zhang, H. L. et al. (2022). Day-ahead and intra-day joint economic dispatching method of electric power system considering combined peak-shaving of fused magnesium load and energy storage. Electric Power Automation Equipment, 42(2), 8–15. [Google Scholar]
18. Chen, Y. B., Wu, C., Jiao, Y., Sun, Z. X., Dai, S. et al. (2022). Coordinated optimal operation strategy of thermal power-energy storage considering demand response and life model of energy storage. Electric Power Automation Equipment, 42(2), 16–24. [Google Scholar]
19. Ye, Z., Li, X. Q., Jiang, F., Chen, L., Wang, Y. L. et al. (2021). Hierarchical optimization economic dispatching of combined wind-PV-thermal-energy storage system considering the optimal energy abandonment rate. PS Technology, 45(6), 2270–2280. [Google Scholar]
20. Zhao, S. Q., Wang, Y., Xu, Y. (2014). Dependent chance programming dispatching of integrated thermal power generation and energy storage system based on wind energy forecasting error. Proceedings of the CSEE, 34(S1), 9–16 (In Chinese). [Google Scholar]
21. Wang, Y. C., Lou, S. H., Wu, Y. W., Wang, S. R. (2020). Flexible operation of retrofitted coal-fired power plants to reduce wind emission considering thermal energy storage. IEEE Transactions on Power Systems, 35(2), 1178–1187. [Google Scholar]
22. Zhao, S. Q., Wu, Y., Li, Z. W., Wei, Z. Y., Lin, J. W. (2022). Analysis of PS peaking capacity and economy considering uncertainty of wind and solar output. Power System Technology, 46(05), 1752–1761. [Google Scholar]
23. Zhang, M. G., Xu, W. Q., Zhao, W. Y. (2023). Combined optimal dispatching of wind-light-fire-storage considering electricity price response and uncertainty of wind and photovoltaic power. Energy Reports, 9(S1), 790–798. [Google Scholar]
24. Lin, L., Xu, B. Q., Xia, S. W. (2019). Multi-Angle economic analysis of coal-fired generators with plasma ignition and oil injection during deep peak clipping in China. Applied Sciences, 9(24), 5399. [Google Scholar]
25. Lin, L., Tian, X. Y. (2017). Analysis of deep peak regulation and its benefit of thermal generators in power system with large scale wind energy integrated. Power System Technology, 41(7), 2255–2263. [Google Scholar]
26. Zhu, L. B., Sun, S. Q., Chang, L., Wei, G. H., Lu, Z. G. (2010). Quantitative relationship between load factor and power loss and application in cost-benefit analysis of TOU. Power System Protection and Control, 38(17), 43–46+52 (In Chinese). [Google Scholar]
Appendix A
Cite This Article
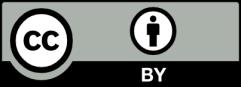
This work is licensed under a Creative Commons Attribution 4.0 International License , which permits unrestricted use, distribution, and reproduction in any medium, provided the original work is properly cited.