Open Access
ARTICLE
Investigating Periodic Dependencies to Improve Short-Term Load Forecasting
1 State Grid Zhejiang Jiaxing Electric Power Co., Ltd., Jiaxing, 314000, China
2 State Grid Zhejiang Electric Power Co., Ltd., Hangzhou, 310000, China
* Corresponding Author: Jialin Yu. Email:
Energy Engineering 2024, 121(3), 789-806. https://doi.org/10.32604/ee.2023.043299
Received 28 June 2023; Accepted 09 October 2023; Issue published 27 February 2024
Abstract
With a further increase in energy flexibility for customers, short-term load forecasting is essential to provide benchmarks for economic dispatch and real-time alerts in power grids. The electrical load series exhibit periodic patterns and share high associations with metrological data. However, current studies have merely focused on point-wise models and failed to sufficiently investigate the periodic patterns of load series, which hinders the further improvement of short-term load forecasting accuracy. Therefore, this paper improved Autoformer to extract the periodic patterns of load series and learn a representative feature from deep decomposition and reconstruction. In addition, a novel multi-factor attention mechanism was proposed to handle multi-source metrological and numerical weather prediction data and thus correct the forecasted electrical load. The paper also compared the proposed model with various competitive models. As the experimental results reveal, the proposed model outperforms the benchmark models and maintains stability on various types of load consumers.Keywords
Cite This Article
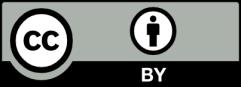
This work is licensed under a Creative Commons Attribution 4.0 International License , which permits unrestricted use, distribution, and reproduction in any medium, provided the original work is properly cited.