Open Access
ARTICLE
The Short-Term Prediction of Wind Power Based on the Convolutional Graph Attention Deep Neural Network
1 State Grid Hubei Electric Power Research Institute, Wuhan, 430077, China
2 College of Energy and Electrical Engineering, Hohai University, Nanjing, 210098, China
* Corresponding Author: Yeyang Li. Email:
(This article belongs to the Special Issue: Wind Energy Development and Utilization)
Energy Engineering 2024, 121(2), 359-376. https://doi.org/10.32604/ee.2023.040887
Received 03 April 2023; Accepted 08 August 2023; Issue published 25 January 2024
Abstract
The fluctuation of wind power affects the operating safety and power consumption of the electric power grid and restricts the grid connection of wind power on a large scale. Therefore, wind power forecasting plays a key role in improving the safety and economic benefits of the power grid. This paper proposes a wind power predicting method based on a convolutional graph attention deep neural network with multi-wind farm data. Based on the graph attention network and attention mechanism, the method extracts spatial-temporal characteristics from the data of multiple wind farms. Then, combined with a deep neural network, a convolutional graph attention deep neural network model is constructed. Finally, the model is trained with the quantile regression loss function to achieve the wind power deterministic and probabilistic prediction based on multi-wind farm spatial-temporal data. A wind power dataset in the U.S. is taken as an example to demonstrate the efficacy of the proposed model. Compared with the selected baseline methods, the proposed model achieves the best prediction performance. The point prediction errors (i.e., root mean square error (RMSE) and normalized mean absolute percentage error (NMAPE)) are 0.304 MW and 1.177%, respectively. And the comprehensive performance of probabilistic prediction (i.e., continuously ranked probability score (CRPS)) is 0.580. Thus, the significance of multi-wind farm data and spatial-temporal feature extraction module is self-evident.Keywords
Cite This Article
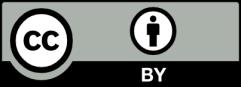
This work is licensed under a Creative Commons Attribution 4.0 International License , which permits unrestricted use, distribution, and reproduction in any medium, provided the original work is properly cited.