Open Access
ARTICLE
Enhanced Deep Reinforcement Learning Strategy for Energy Management in Plug-in Hybrid Electric Vehicles with Entropy Regularization and Prioritized Experience Replay
1 School of Computer Science and Engineering, Anhui University of Science & Technology, Huainan, 232001, China
2 School of Information Engineering, Huainan Union University, Huainan, 232001, China
* Corresponding Author: Li Wang. Email:
Energy Engineering 2024, 121(12), 3953-3979. https://doi.org/10.32604/ee.2024.056705
Received 29 July 2024; Accepted 26 September 2024; Issue published 22 November 2024
Abstract
Plug-in Hybrid Electric Vehicles (PHEVs) represent an innovative breed of transportation, harnessing diverse power sources for enhanced performance. Energy management strategies (EMSs) that coordinate and control different energy sources is a critical component of PHEV control technology, directly impacting overall vehicle performance. This study proposes an improved deep reinforcement learning (DRL)-based EMS that optimizes real-time energy allocation and coordinates the operation of multiple power sources. Conventional DRL algorithms struggle to effectively explore all possible state-action combinations within high-dimensional state and action spaces. They often fail to strike an optimal balance between exploration and exploitation, and their assumption of a static environment limits their ability to adapt to changing conditions. Moreover, these algorithms suffer from low sample efficiency. Collectively, these factors contribute to convergence difficulties, low learning efficiency, and instability. To address these challenges, the Deep Deterministic Policy Gradient (DDPG) algorithm is enhanced using entropy regularization and a summation tree-based Prioritized Experience Replay (PER) method, aiming to improve exploration performance and learning efficiency from experience samples. Additionally, the corresponding Markov Decision Process (MDP) is established. Finally, an EMS based on the improved DRL model is presented. Comparative simulation experiments are conducted against rule-based, optimization-based, and DRL-based EMSs. The proposed strategy exhibits minimal deviation from the optimal solution obtained by the dynamic programming (DP) strategy that requires global information. In the typical driving scenarios based on World Light Vehicle Test Cycle (WLTC) and New European Driving Cycle (NEDC), the proposed method achieved a fuel consumption of 2698.65 g and an Equivalent Fuel Consumption (EFC) of 2696.77 g. Compared to the DP strategy baseline, the proposed method improved the fuel efficiency variances (FEV) by 18.13%, 15.1%, and 8.37% over the Deep Q-Network (DQN), Double DRL (DDRL), and original DDPG methods, respectively. The observational outcomes demonstrate that the proposed EMS based on improved DRL framework possesses good real-time performance, stability, and reliability, effectively optimizing vehicle economy and fuel consumption.Keywords
Cite This Article
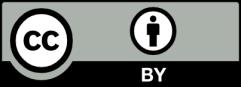
This work is licensed under a Creative Commons Attribution 4.0 International License , which permits unrestricted use, distribution, and reproduction in any medium, provided the original work is properly cited.