Open Access
ARTICLE
Probabilistic Calculation of Tidal Currents for Wind Powered Systems Using PSO Improved LHS
School of Automation and Electrical Engineering, Lanzhou Jiaotong University, Lanzhou, 730070, China
* Corresponding Author: Shilin Song. Email:
Energy Engineering 2024, 121(11), 3289-3303. https://doi.org/10.32604/ee.2024.054643
Received 03 June 2024; Accepted 27 July 2024; Issue published 21 October 2024
Abstract
This paper introduces the Particle Swarm Optimization (PSO) algorithm to enhance the Latin Hypercube Sampling (LHS) process. The key objective is to mitigate the issues of lengthy computation times and low computational accuracy typically encountered when applying Monte Carlo Simulation (MCS) to LHS for probabilistic trend calculations. The PSO method optimizes sample distribution, enhances global search capabilities, and significantly boosts computational efficiency. To validate its effectiveness, the proposed method was applied to IEEE34 and IEEE-118 node systems containing wind power. The performance was then compared with Latin Hypercubic Important Sampling (LHIS), which integrates significant sampling with the Monte Carlo method. The comparison results indicate that the PSO-enhanced method significantly improves the uniformity and representativeness of the sampling. This enhancement leads to a reduction in data errors and an improvement in both computational accuracy and convergence speed.Keywords
Cite This Article
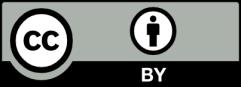
This work is licensed under a Creative Commons Attribution 4.0 International License , which permits unrestricted use, distribution, and reproduction in any medium, provided the original work is properly cited.