Open Access
ARTICLE
Analysis of System Value Evolution Trends of Energy Storage in Decarbonization Process
1 State Grid Liaoning Electric Power Supply Co., Ltd., State Grid Liaoning Electric Power Company Limited Economic Research Instituete, Shenyang, 110015, China
2 School of Electrical and Electronic Engineering, North China Electric Power University, Beijing, 102206, China
* Corresponding Author: Zili Chen. Email:
Energy Engineering 2024, 121(10), 3037-3053. https://doi.org/10.32604/ee.2024.050842
Received 20 February 2024; Accepted 24 June 2024; Issue published 11 September 2024
Abstract
With the continuous expansion of the scale of renewable energy installation, the demand for energy storage has increased significantly. However, there are significant differences in the value of energy storage in different scenarios, and the phenomenon of diminishing marginal benefits of energy storage is becoming more apparent. Therefore, the multi-dimensional value evolution trend of energy storage has become a key issue. This study selects indicators from three dimensions of energy storage: low-carbon emission reduction, smoothing wind and solar power fluctuations, and saving generation costs, quantifying the economic, environmental, and technical values of energy storage. This forms a quantitative evaluation system for energy storage value. By comparing the calculated system values under different energy storage capacities, the marginal value evolution trend of energy storage is obtained. Meanwhile, considering factors such as the utilization rate of renewable energy, the change in energy storage value under different scenarios is analyzed. The results show that the value of long-duration energy storage is significantly affected by the energy storage capacity. Specifically, when the charge-discharge efficiency of long-duration energy storage reaches 0.6 or above, the system value increases significantly. Additionally, appropriately reducing the cost of energy storage capacity also helps to improve its system value.Keywords
Nomenclature
Pesg,tPesg,t | The output of conventional units at time tt |
χco2χco2 | The carbon emission intensity of conventional units |
cco2,tcco2,t | The carbon emission rights price |
TT | The energy storage dispatch cycle |
VsfVsf | The value of smoothing out wind and solar power output fluctuations |
Pupw,tPupw,t | The charging and discharging power of energy storage during time tt |
Pmaxw,tPmaxw,t | The upper and lower limits of wind farm output |
Pw,tPw,t | The actual output power of the wind farm during time tt |
WsfWsf | The charge and discharge capacity of energy storage |
Facing the increasingly prominent energy and environmental problems, China is vigorously developing clean energy and energy storage technologies. A high proportion of clean energy grid connection will become an inevitable development trend and an important feature of China’s power system [1]. With the issuance of the “14th Five-Year Plan for Modern Energy System” by the National Energy Administration, China has made preliminary plans for the current energy development policy, main goals, and task measures [2]. With the increase in the proportion of new energy sources such as wind and light on the power supply side, the randomness and uncertainty of output put higher requirements on the construction of the power system [3–5]. Coordinating the joint operation of multiple types of energy storage is of great significance to alleviate the mismatch between the characteristics of new energy power generation and load, and is conducive to accelerating the process of building a high-proportion clean energy power system in China.
The complementarity of multi-time scale energy storage is beneficial for promoting the widespread application of storage across various sectors. To achieve seasonal energy shifting within the system and to smooth out daily, weekly, and even yearly power fluctuations, long-duration, large-capacity energy storage technologies are required [6]. Compared to long-duration storage, short-duration storage such as electrochemical storage is more flexible in deployment, has shorter construction periods, and responds quickly. However, the cost of electrochemical storage is currently high [7]. Under the strong push of policies and technological advancements, the capacity of renewable energy paired with energy storage is continuously increasing, and the energy storage costs are showing a downward trend year by year [8]. Nonetheless, it must be acknowledged that the current drivers of energy storage development are primarily local-level consumption demands and subsidy policies, limited by the current electricity pricing mechanism and marketization degree [9]. In this context, researching comprehensive value quantification methods for multi-time scale energy storage systems is of significant importance.
With the widespread application of different types of flexibility resources, the extensive construction of clean power sources, and the increasing complexity of the grid structure, the differences in the value exhibited by energy storage systems in the face of rapid changes in China’s energy landscape have become more prominent. Therefore, assessing the changing trends of energy storage value in different scenarios has become an urgently needed problem to solve. However, existing research on energy storage technology mainly focuses on the following aspects: 1) Research on the operational efficiency of energy storage technology itself. Reference [10] analyzed the significant role of energy storage operational efficiency in reshaping energy infrastructure construction and energy market reform. Reference [11] proposed a method for comparing energy storage operational efficiency, providing optimal reference solutions for investment decisions for different types of energy storage in various scenarios. 2) Research on the investment cost of energy storage technology. Reference [8] constructed an empirical curve to predict the future price changes of 11 types of energy storage. Reference [12] proposed a two-stage optimization model and a time-based pricing strategy for energy storage costs to incentivize investment in user-side energy storage. 3) Operation mechanisms for energy storage participation in markets. Reference [13] proposed an alternative energy price market mechanism compatible with existing supply-demand relationships and the essential nature of sustainable renewable energy development. It can be observed from the above research that they mainly focus on the operational efficiency, cost, and market mechanisms of energy storage itself, while neglecting the in-depth exploration of the multidimensional value of energy storage systems across multiple time scales. The comprehensive value of energy storage systems across multiple dimensions and time scales has yet to be fully explored. With the increase in the penetration rate of new energy and the improvement of smart grids, energy storage systems not only need to meet supply-demand balance and regulate peak-to-valley electricity price differentials but also play a crucial role in ensuring flexible operation and enhancing grid operational stability. Additionally, due to market mechanism reforms and adjustments in energy policies, the potential value of energy storage systems is continually increasing. To fully leverage the role of energy storage systems, comprehensive evaluation of their system value is needed, especially in terms of quantitatively evaluating across time scales and meeting diversified energy demand, through joint planning and operation of various types of energy storage with new energy to maximize the value of energy storage systems.
In addition, similar to the investment in conventional energy power resources, the investment in energy storage resources will also have a diminishing marginal benefit effect. When the system continues to expand the investment scale of energy storage capacity, the additional value brought by each additional unit of energy storage capacity will gradually decrease [14]. This not only involves the initial investment cost of energy storage equipment, but also includes long-duration costs in operation and maintenance, and system integration [15]. Therefore, although energy storage may bring significant economic and technical benefits in the initial investment, its marginal value may gradually weaken as the investment scale expands. However, in [16–18], they focused more on maximizing the value of energy storage in a single application scenario, and ignored the differential impact of the phenomenon of diminishing marginal value of energy storage under the influence of various external factors on the investment decision of energy storage capacity. Therefore, it is urgent to deeply explore the value evolution law of energy storage in different scenarios, in order to determine how to balance the investment and return of energy storage on the basis of maximizing system value, and find the operation planning strategy that adapts to the diversified energy supply demand.
To address the aforementioned shortcomings, this paper quantifies the multi-dimensional value of energy storage at different time scales and conducts an in-depth analysis of its value evolution trend. The main contributions are as follows:
(1) Considering the benefits of energy storage in ensuring power supply and reducing carbon emissions, a multi-dimensional value evaluation framework is designed for multi-time scale energy storage systems. This framework incorporated details modeling of energy storage across different time scales, forming a comprehensive value evaluation index system for multi-time scale energy storage. Additionally, the framework exhibits strong adaptability and scalability, allowing for a reasonable evaluation of system values for various types of energy storage.
(2) A calculation method is designed which suits to the value evolution trends of energy storage across different time scales. By incrementally increasing the actual capacity of storage integrated into the system, we quantitatively assesses the corresponding storage value at different capacity points, forming a value curve for the energy storage system. This method balances the value of multi-time scale energy storage systems with investment costs, achieving a balance between system operational economics and low-carbon performance. The value search results indicate that the evolution of the energy storage system value in this region follows a three-stage change trend: value increase, value saturation, and value decline. Furthermore, when the system is configured with multi-time scale energy storage, its storage value can increase by approximately 7.2%.
(3) The evolution trends of the marginal benefits of energy storage systems across diverse application scenarios are explored. Additionally, it analyzes the correlation between various influencing factors and the value of energy storage systems. The results indicate that in the early phase of this region’s low-carbon clean power system transition (2023–2030), electrochemical storage and other short-term storage should be prioritized. In the later phase of the transition (2030–2050), more long-duration storage should be deployed. When the utilization rate of new energy reaches 90% in 2030, and the charge-discharge efficiency of long-duration storage is at or above 0.6, the value of the energy storage system is relatively high.
The remaining structure of this paper is as follows. In Section 2, we propose a multi-dimensional value quantitative evaluation system for the energy storage system. In Section 3, we construct a quantitative evaluation model for the multi-dimensional value of energy storage, corporating detailed modeling of multi-time scale energy storage. In Section 4, we conduct an empirical analysis of the changing law of the value of the energy storage system. Section 5 summarizes the paper.
In this section, we constructed an analysis framework for the evolution trend of energy storage system value. The framework consists of two parts, namely the quantification assessment system of energy storage system value and the calculation method of energy storage value evolution trend. The energy storage system value mentioned in the text mainly includes three dimensions: economic value, environmental value, and technical value. The framework quantitatively evaluates the value of energy storage based on actual wind and solar power output data, load curves, various cost parameters, and unit operation parameters within a certain regional power system. It mainly involves the following steps:
1) Firstly, considering the significant roles of energy storage in peak shaving, carbon emission reduction, and smoothing renewable energy output, we selected quantitative evaluation indicators for the economic, technical, and environmental values of energy storage. For the economic value of energy storage, the indicator chosen was the generation cost savings. This was evaluated by first simulating the change in thermal power output when energy storage replaces part of the thermal power, then calculating the change in fuel generation costs of thermal power. The economic value of energy storage was quantified by measuring the difference in total system costs before and after the integration of energy storage.
2) Subsequently, for the technical value of energy storage, the smoothing effect on renewable energy output fluctuations was used as the evaluation indicator. This was quantified by first simulating and measuring the smoothing effect of energy storage on the renewable energy output and then converting this effect into the economic benefits from reduced renewable energy output fluctuations. This approach provided a quantifiable measure of the technical value of energy storage.
3) For the environmental value of energy storage, the carbon reduction effect served as the evaluation indicator. This was quantified by simulating the reduction in system carbon emissions after the integration of energy storage and converting the reduced carbon emissions into decreased carbon emission costs. This method standardized the dimensions of different evaluation indicators for the comprehensive value of energy storage systems, forming a quantitative evaluation index system for the overall value of energy storage.
4) Then, we constructed a comprehensive value assessment model for energy storage systems based on the multidimensional value quantification indicators. We designed calculation methods for the evolution trends of different types of energy storage value, wherein we incrementally increased the actual capacity of energy storage systems and quantitatively calculated the corresponding value of energy storage systems at different capacity points. This process formed energy storage system value curves, enabling the identification of marginal value evolution trends within the system. By evaluating the change in curve values, we assessed the benefits brought by adding unit energy storage capacity to the system, thereby determining the marginal benefits of energy storage systems.
5) Lastly, by comparing the system values at different energy storage capacities, we aimed to identify the capacity point that maximizes the marginal value of the energy storage system.
Through the process of quantitative evaluation and comparison of the value of the energy storage system, it ensures that the capacity corresponding to the maximization of value in multiple dimensions of the energy storage system can provide theoretical guidance for energy storage planning.
In this section, we establish an evaluation index system for the multi-dimensional value of energy storage, considering its economic and environmental benefits. The indices are based on the benefits of energy storage in carbon emission reduction, smoothing renewable energy output, and saving generation costs. A detailed analysis was conducted based on the proposed energy storage value evaluation indicators using hour-level operational simulations. By performing simulations over different time scales, the marginal value of the energy storage system can be calculated according to actual needs across various scales (e.g., intra-day, weekly, monthly, or yearly). This method captures the operational characteristics and economic benefits of energy storage over different time scales, facilitating a comprehensive assessment of its multi-dimensional value.
In terms of economic value, the model takes into account the role of energy storage in reducing system construction, maintenance, and operation costs. The assessment of energy storage environmental value focuses on its effectiveness in reducing system carbon emissions and its role in promoting the integration of renewable energy. In the evaluation of technical value, it is necessary to analyze how energy storage enhances power supply reliability and stability. The objective function of the model is to maximize system value, including three components: economic, environmental, and technical value of energy storage, as follows:
maxV=Venv+Vsf+VecomaxV=Venv+Vsf+Veco(1)
where, VV represents the comprehensive value of the system.
(1) Quantification of environmental value
Venv=T∑t=1(Pg,t−Pesg,t)×χCO2×cco2,tVenv=T∑t=1(Pg,t−Pesg,t)×χCO2×cco2,t(2)
where, VenvVenv represents the low-carbon emission reduction value of energy storage, Pg,tPg,t denotes the output of conventional units at time tt when the system does not incorporate energy storage, Pesg,tPesg,t represents the output of conventional units at time tt when planning in conjunction with new energy and energy storage, χco2χco2 stands for the carbon emission intensity of conventional units, cco2,tcco2,t is the carbon emission rights price, and TT denotes the energy storage dispatch cycle.
(2) Quantification of technological value
Pupw,t=Pw,t−Pmaxw,t,Ploww,t=Pminw,t−Pw,tPupw,t=Pw,t−Pmaxw,t,Ploww,t=Pminw,t−Pw,t(3)
Wsf=T∑t=1(Pupw,t+Ploww,t)ΔtWsf=T∑t=1(Pupw,t+Ploww,t)Δt(4)
Pups,t=Ps,t−Pmaxs,t,Plows,t=Pmins,t−Ps,tPups,t=Ps,t−Pmaxs,t,Plows,t=Pmins,t−Ps,t(5)
Ssf=T∑t=1(Pups,t+Plows,t)ΔtSsf=T∑t=1(Pups,t+Plows,t)Δt(6)
Vsf=Wsfζw+SsfζsVsf=Wsfζw+Ssfζs(7)
where, VsfVsf represents the value of smoothing out wind and solar power output fluctuations, Pupw,tPupw,t and Ploww,tPloww,t respectively denote the charging and discharging power of energy storage during time tt to smooth out wind farm output fluctuations, Pmaxw,tPmaxw,t and Pminw,tPminw,t are the upper and lower limits of the allowable fluctuation range of wind farm output during time tt, Pw,tPw,t is the actual output power of the wind farm during time tt, and WsfWsf represents the charge and discharge capacity of energy storage for smoothing out wind farm output fluctuations. Similarly, Pups,tPups,t and Plows,tPlows,t represent the charging and discharging power of energy storage during time tt to smooth out photovoltaic output fluctuations. Pmaxs,tPmaxs,t and Pmins,tPmins,t are the upper and lower limits of the allowable fluctuation range of photovoltaic output during time tt. Ps,tPs,t is the actual output power of the photovoltaic plant during time tt, and SsfSsf is the charge and discharge capacity of energy storage for smoothing out photovoltaic output fluctuations. ζwζw and ζsζs respectively denote the grid prices for wind and photovoltaic power.
(3) Quantification of economic value
Veco=(Cinvsys−Cinv,essys)+(Copesys−Cope,essys)Veco=(Cinvsys−Cinv,essys)+(Copesys−Cope,essys)(8)
Cinvsys=(Ewcw+Escs)λnes(1+λnes)ynes365[(1+λnes)ynes−1]Cinvsys=(Ewcw+Escs)λnes(1+λnes)ynes365[(1+λnes)ynes−1](9)
Cinv,essys=(Eeswcw+Eesscs)λ(1+λ)ynes365[(1+λ)ynes−1]+CinvesCinv,essys=(Eeswcw+Eesscs)λ(1+λ)ynes365[(1+λ)ynes−1]+Cinves(10)
Copesys=T∑t=1[ug,tfg(Pg,t)Δt+SCgug,t]Copesys=T∑t=1[ug,tfg(Pg,t)Δt+SCgug,t](11)
Cope,essys=T∑t=1[µesg,tfg(Pesg,t)Δt+SCgµesg,t+(Pch,t+Pdis,t)Δt](12)
fg(Pg,t)=agP2g,t+bgPg,t+cg(13)
where, Veco represents the value of energy storage in saving generation costs. Cinvsys and Cinv,essys respectively denote the investment costs before and after integrating energy storage into the system. Copesys and Cope,essys represent the operational costs of units before and after integrating energy storage. Cinves is the investment cost of energy storage. Ew and Es, as well as Eesw and Eess, represent the installed capacities of wind and solar units before and after integrating energy storage. cw and cs are the unit investment costs for wind and solar units, respectively. λ is the discount rate, fg(⋅) represents the fuel cost function for conventional unit g, μg,t is a binary variable taking values between 0 and 1, indicating the start-up status of unit g at time t. SCg,t represents the corresponding start-up cost, and Pch,t and Pdis,t denote the charging and discharging powers of the energy storage.
Regarding the investment costs of energy storage at different time scales, as follows:
Cinves,LD=(Einvescees,LD+Pinves,chcpch,LD+Pinves,discpdis,LD)λ(1+λ)yesLD365[(1+λ)yesLD−1](14)
Cinves,ST=(Einvescees,ST+Pinvescpes,ST)λ(1+λ)yesST365[(1+λ)yesST−1](15)
where, Cinves,LDES and Cinves,STES represent the investment costs for long-duration energy storage and short-term energy storage, respectively. Einves denotes the energy storage capacity and power planned for the system. Pinves,ch and Pinves,dis are the charging and discharging power planned for the long-duration energy storage system. yes is the service life of the energy storage system. cees and cpes are the unit investment costs for energy storage capacity and power, respectively. cees,ch and cees,dis represent the unit investment costs for the charging and discharging power of the energy storage system.
3.2 Multi-Timescale Energy Storage Operation Model
The multi-time scale energy storage in this paper includes short-term storage and long-duration storage. To address the operational characteristics of different time-scale energy storage, we establish energy storage operation models as follows:
En,t=En,t−1+(Pchn,tηchn−Pdisn,t/ηdisn)Δt,∀n∈Ω(16)
0≤En,t≤Einvn,Einvn≤Einvn,max∀n∈Ω(17)
En,T=En,0,∀n∈Ω(18)
0≤Pdisn,t≤Pdisn,max,0≤Pchn,t≤Pchn,max,∀n∈Ω(19)
Pdisn,tΔt≤En,t−1ηdisn,Pchn,tηchnΔt≤Einvn,es−En,t−1,∀n∈Ω(20)
δminn≤Einvn,es/Pdisn,max≤δmaxn,∀n∈Ω(21)
where, Pchn,max and Pdisn,max respectively correspond to the upper limits of charging and discharging power capacities for long-duration energy storage, δminn and δmaxn represent the minimum and maximum energy-to-power ratios for long-duration energy storage, and Ω denotes the set of different time-scale energy storage. Eqs. (16)–(19) constitute the operational simulation model for short-term energy storage, while Eqs. (16)–(21) represent the operational model for long-duration energy storage. Eqs. (16)–(17) denote the capacity constraints for energy storage, Eq. (18) represents the charge-discharge balance constraint, and Eqs. (19)–(20) denote the constraints on charge and discharge power. For long-duration energy storage, its performance parameters are decoupled into charging power capacity and discharging power capacity, where the charge and discharge powers are limited by these two performance parameters. Eq. (21) represents the capacity-to-power ratio for long-duration energy storage.
The operational constraints for wind and solar power, as well as conventional generating units, primarily include output upper and lower limits, as follows:
∑n∈Ω(Pdisn,t−Pchn,t)+Pw,t+Ps,t+Pg,t=Ploadt(22)
Pw,t≤Pmaxw,Ps,t≤Pmaxs,Pg,t≤Pmaxg(23)
The operational constraints of the units mentioned above and the multi-time scale energy storage model together constitute the system operational constraints. The objective function of the model, maximizing the value of the energy storage system, is convex, as are the constraints. Additionally, the feasible domain is non-empty. For energy storage systems of different capacities integrated into the system, the system value satisfying the constraints can be calculated. Clearly, the model is a convex optimization model, implying the existence of a global optimal energy storage capacity that maximizes system value.
This paper uses a system from a province in Northeast China as an empirical case for joint planning analysis of new energy and energy storage. In 2023, the short-term energy storage capacity in the region reached 4000 MWh/2000 MW, while the long-duration energy storage installed capacity reached 9600 MWh/1200 MW. Based on China’s research on energy development by 2030, the study expands its research under boundary conditions using forecasted data and economic and technical parameters for the region by 2030 (such as various types of power generation scales, loads, energy storage capacity costs, etc.) [19]. Specific parameters can be found in Table 1. By comparing multiple scenarios with the base scenario, the system value of energy storage at different time scales is quantified.
4.2 Multi-Time Scale Energy Storage Value Analysis
4.2.1 Long-Duration Energy Storage System Value
When there are changes in the planned capacity of long-duration energy storage within the system, the value of the energy storage system will also vary accordingly, resulting in the energy storage system value curve as depicted in Fig. 1.
Figure 1: Trends in the evolution of value for long-duration energy storage systems
From Fig. 1, it can be observed that as the system’s integration of storage capacity increases, the total system cost first decreases and then increases. This is primarily because after integrating storage into the system, the output of thermal power units can be replaced, resulting in a significant reduction in both the generation cost of thermal power units and the carbon emission cost of the system. This reduction can offset the increase in investment and operational costs associated with energy storage, leading to a decrease in the total system cost. However, there exists a saturation effect in the system’s demand for energy storage capacity. Continuous growth in energy storage capacity can lead to problems of capacity redundancy. At this point, the economic and low-carbon benefits brought by energy storage are lower than the investment and operational costs associated with increasing energy storage capacity, resulting in an increase in the total system cost thereafter.
Corresponding to the evolution trend of the value of systems, it can be roughly divided into four stages. Initially, as the long-duration energy storage capacity is gradually increased, the trend of value change is relatively slow, and the additional energy storage capacity contributes relatively limitedly to the overall energy supply. However, when the long-duration energy storage capacity reaches a certain level, it can continuously provide a large-scale power supply to the system. At this point, the cost of energy storage is lower than the benefits it generates, leading to a rapid increase in system value. However, as the capacity of long-duration energy storage continues to increase, it may surpass the system’s demand for long-duration storage, causing the system value to change more gradually. Further increasing the long-duration energy storage capacity will result in capacity redundancy issues, leading to a decline in the value of the energy storage.
4.2.2 Short-Term Energy Storage System Value
Fig. 2 displays the value curve of a short-term energy storage system as it varies with changes in the planned storage capacity.
Figure 2: Trends in the evolution of value for short-term energy storage systems
As shown in Fig. 2, the evolution of short-term energy storage value can be broadly divided into three stages. Initially, as the energy storage capacity begins to incrementally increase, introducing short-term energy storage effectively mitigates the volatility of new energy sources, leading to an enhancement in system value due to the increased storage. However, when the energy storage capacity grows to a certain point, given that the operational and investment costs of short-term energy storage are relatively high, the cost increment brought by the introduction of energy storage starts to approach the value it provides to the system. Consequently, the increase in energy storage capacity does not yield a proportional rise in value, resulting in a very slow growth rate of the short-term energy storage system value. Beyond the optimal storage capacity, continuously increasing the short-term energy storage will lead to storage surplus, causing a gradual decline in system value.
In summary, the evolution of the value of long and short-term energy storage systems roughly conforms to the following three-stage trend: the system value increases with the increase of energy storage capacity, reaches its highest point, and then decreases as the system continues to integrate more energy storage capacity. This is mainly because, in the initial stage, as the system integrates more energy storage capacity, the value of the energy storage system in terms of carbon reduction, smoothing renewable energy output fluctuations, and saving generation costs increases significantly. However, as the energy storage capacity continues to increase, its marginal benefits decrease. This is because, after meeting the basic requirements, additional energy storage capacity may only be used to handle less frequent extreme situations, resulting in a smaller contribution to the overall energy system value. Moreover, excessive integration of energy storage capacity can lead to capacity redundancy issues, significantly increasing operational costs and additional investment costs for energy storage, thereby reducing the system value.
By comparing the system values of long and short-term energy storage in different periods in this region, it can be observed that the decarbonization process in the region roughly takes place around the year 2030. In the early stage of the region’s clean and low-carbon transition (2024–2030), the installed capacity of short-term energy storage approximately doubles that of 2023, while the installed capacity of long-duration energy storage expands to 2–3 times. During this stage, priority should be given to the deployment of short-term energy storage such as electrochemical storage. Conversely, in the later stage of the transition (2030–2050), deploying long-duration energy storage is more conducive to enhancing system value. This is mainly because the saturation effect of the value of short-term energy storage becomes significant in the later stages of the transition in this region.
4.3 Multi-Factor Energy Storage Value Analysis
We based on the boundary data of the Northeast region in 2030 for the study. According to the analysis of the evolution trend of multi-time scale energy storage value in Section 4.2, after 2030, the region’s demand for flexibility resources will lean more towards long-duration energy storage. Therefore, this section focuses on analyzing the role of long-duration energy storage in improving renewable energy utilization and system operational economics in this region.
1) Ratio of new energy utilization
Fig. 3 illustrates the value changes of long-duration energy storage systems under different rates of new energy utilization.
Figure 3: The system value changes under different rates of new energy integration (The a–d representing the system value changes corresponding to renewable energy utilization rates of 0.8, 0.85, 0.9, and 0.95, respectively)
Clearly, as the prescribed wind and solar utilization rates of the system increase, the system requires more flexible energy dispatch methods to adapt to the continuously changing energy generation. Consequently, there is a growing demand for long-duration energy storage capacity in the system. Additionally, it can be observed that with the continuous increase in the optimal capacity of long-duration energy storage, its corresponding marginal benefits also increase.
2) Long-duration energy storage capacity cost
Fig. 4 illustrates the evolution trend of energy storage system value as the unit capacity cost of LDES changes. It can be observed that when the energy storage capacity cost exceeds 2100 × 103 $/MWh, the marginal benefits of long-duration energy storage essentially fall below 5%. For LDES at different capacity costs, the system value generally does not exceed 70%, corresponding to the theoretically optimal performance development level for long-duration energy storage. The highest system value corresponds to a unit capacity cost of long-duration energy storage ranging from 1050 × 103 $/MWh to 1400 × 103 $/MWh. Additionally, as the cost of energy storage capacity increases, the system reduces its demand for long-duration energy storage to some extent.
Figure 4: The changes in energy storage system value under different unit capacity costs for long-duration energy storage
3) Energy storage charging and discharging efficiency
The efficiency of energy storage charging and discharging is a crucial indicator for assessing the performance and value of an energy storage system. It directly affects the economic benefits and sustainability of the energy storage system. Figs. 5 and 6 depict the evolution trend of energy storage system value corresponding to changes in the charging and discharging efficiency of long-duration energy storage.
Figure 5: Trends in energy storage value changes under different charging efficiencies
Figure 6: Trends in energy storage value changes under different discharging efficiencies
From Fig. 5, it can be observed that when the charging efficiency improves, the system’s demand for energy storage capacity correspondingly decreases. As a result, the system’s investment and operational costs decrease. Concurrently, the utilization rate of new energy and the system conversion efficiency increase, leading to an enhancement in system value. When the charging efficiency of long-duration energy storage reaches 0.6 or higher, the system value is relatively high. However, if the planned capacity of long-duration energy storage exceeds approximately 1.3 times the optimal capacity, the system value will plummet to a very low level.
As can be seen from Fig. 6, with the increase in the discharge efficiency of long-duration energy storage, the optimal capacity of long-duration energy storage corresponding to the maximumsystem value also continues to decrease. At the same time, the increase in discharge efficiency makes the system more adaptable to the volatility of new energy, so the maximum value of the corresponding system value is also constantly increasing. When the discharge efficiency of long-duration energy storage is above 0.6, the system value is greatly affected by the planning results of energy storage capacity. This is because the increase in discharge efficiency reduces the energy capacity and charging power capacity required for long-duration energy storage to release a certain amount of electricity. That is to say, under the same other conditions, a higher discharge efficiency can reduce the charging power requirements of energy storage and reduce the cost of energy storage capacity.
4.4 The Correlation Analysis of Energy Storage Value
Fig. 7 analyzes the relationship between renewable energy utilization rate and system value. The shading in the figure represents the degree of correlation between renewable energy utilization rate and system value.
Figure 7: The correlation analysis between renewable energy utilization rate and system value
Fig. 7 illustrates a positive correlation between renewable energy utilization rate and system value. The increase in renewable energy utilization rate benefits the reduction of thermal power generation output, thereby decreasing system carbon emissions and enhancing its environmental value. Moreover, the higher renewable energy utilization rate implies a more pronounced effect of long-duration and short-duration energy storage in smoothing output fluctuations across different time scales, leading to an increase in its technical value and consequently boosting the overall system value. When the renewable energy utilization rate exceeds 0.65, a strong correlation with system value is observed, indicating the significance of effective renewable energy utilization in the decarbonization process and emphasizing its proactive role in accelerating the transition to low-carbon and clean systems.
Fig. 8 analyzes the relationship between energy storage unit capacity cost and system value. The shading in the figure represents the degree of correlation between energy storage unit capacity cost and system value.
Figure 8: The correlation analysis between unit capacity cost of LDES and system value
Fig. 8 indicates a negative correlation between energy storage unit capacity cost and system value, meaning that lower energy storage unit capacity costs correspond to higher system values. The reduction in energy storage unit capacity costs benefits from incentivizing system energy storage investments, smoothing output fluctuations across different time scales, enhancing system technical value, and effectively promoting system decarbonization, thus increasing environmental value. Most importantly, the decrease in energy storage unit capacity costs also reduces system costs, thereby improving system economic value.
By comparing Figs. 7 and 8, it can be observed that the correlation between renewable energy utilization rate and system value is stronger, and the variation in renewable energy utilization rate has a greater impact on system value. Therefore, for the new energy installed capacity accounted for a high proportion or wind power generation potential of the region, it is more appropriate to expand the scale of investment in energy storage capacity to improve the new energy utilisation rate, and enhance the comprehensive value of the energy storage system, or also through the improvement of energy storage technology to reduce the cost of its investment and operation, so as to enhance the value of the application of energy storage.
The paper designs a quantitative evaluation system for the multidimensional value of energy storage systems, quantitatively assessing the multidimensional value of storage at different time scales. It delves into the value evolution trends and capacity planning strategies under various influencing factors such as the new energy utilization ratio and capacity cost. The conclusions are as follows:
(1) As the planned long-duration energy storage capacity in the system increases, the value of the energy storage system grows initially at a slow pace and then accelerates. After reaching the optimal value point, there’s a phenomenon of diminishing marginal benefits, leading to a decline in value due to capacity redundancy. The evolution trend of system value for short-term energy storage is similar to long-duration storage, but the saturation effect on the value of short-term storage is more pronounced.
(2) The dominance of multi-time scale energy storage in the region’s power system during different stages of low-carbon clean transformation varies. In the early stage of low-carbon transition, short-term energy storage should be prioritized to mitigate instantaneous fluctuations in wind and solar power, thereby increasing the penetration rate of renewable energy. Conversely, in the later stage of low-carbon transition, the expansion of long-duration energy storage plays a crucial role in ensuring power supply and facilitating low-carbon operation in the region.
(3) The value of multi-time scale energy storage systems changes over time, leading to changes in the priority of energy storage resource allocation. In the current stage, the cost of long-duration energy storage capacity in the region is higher than 3 × 105¥/MWh, resulting in low system value, making it more suitable to prioritize the deployment of short-term energy storage such as electrochemical storage. However, in the later stages of the power system’s low-carbon clean transformation, after the unit capacity cost of long-duration energy storage decreases to 2.4 × 105 ¥/MWh, its system value reaches its maximum level. At this stage, expanding long-duration energy storage capacity will be more economical. Furthermore, in the later stages of the power system transformation, with advances in energy storage technology, when the efficiency of long-duration energy storage charging and discharging exceeds 0.6, the system value will be higher, contributing to the economic and low-carbon operation as well as stable power supply of the system.
In future research, it would be beneficial to comprehensively consider various factors influencing the value of energy storage systems, such as technology costs, policy support, market mechanisms, energy demand patterns, and user behavior. This will allow for a more accurate assessment of the economic benefits, social value, and environmental impact of energy storage systems, providing decision-makers with more scientifically reliable theoretical references and guidance.
Acknowledgement: The Thematic Project of the State Grid Liaoning Electric Power Company Limited Economic Research Institute contributed to this study.
Funding Statement: This work is supported financially by Thematic Project of the State Grid Liaoning Electric Power Company Limited Economic Research Institute under Grant SGLNJY00GHJS2310109.
Author Contributions: Mo Zhou, Conceptualization, Methodology, Software; Jingming Zhao, Methodology, Investigation, Formal analysis; Zili Chen, Visualization, Data curation; Qiushi Fang, Writing–review & editing; Hua Li, Methodology, Formal analysis, Writing–review & editing; Zhaoyuan Wu, Supervision, Funding acquisition. All authors reviewed the results and approved the final version of the manuscript.
Availability of Data and Materials: The data that support the findings of this study are available from the corresponding author, Zili Chen, upon reasonable request.
Ethics Approval: Not applicable.
Conflicts of Interest: The authors declare that they have no conflicts of interest to report regarding the present study.
References
1. Z. Li, W. Zhang, R. Zhang, and H. Sun, “Development of renewable energy multi-energy complementary hydrogen energy system (a case study in ChinaA review,” Energ. Explor. Exploit., vol. 38, no. 6, pp. 2099–2127, Nov. 2020. doi: 10.1177/0144598720953512. [Google Scholar] [CrossRef]
2. Z. Zhao, K. Yang, and Y. Xu, “Low-carbon transformation of power structure under the “double carbon” goal: Power planning and policy implications,” Environ. Sci. Pollut. Res., vol. 30, no. 25, pp. 66961–66977, 2023. doi: 10.1007/s11356-023-27027-9. [Google Scholar] [PubMed] [CrossRef]
3. Z. Wu, M. Zhou, J. Wang, E. Du, N. Zhang and G. Li, “Profit-sharing mechanism for aggregation of wind farms and concentrating solar power,” IEEE Trans. Sustain. Energy, vol. 11, no. 4, pp. 2606–2616, 2020. doi: 10.1109/TSTE.2020.2967860. [Google Scholar] [CrossRef]
4. A. Zakaria, F. B. Ismail, M. H. Lipu, and M. A. Hannan, “Uncertainty models for stochastic optimization in renewable energy applications,” Renew. Energy, vol. 145, no. 4, pp. 1543–1571, 2020. doi: 10.1016/j.renene.2019.07.081. [Google Scholar] [CrossRef]
5. H. Yang et al., “Exploiting the operational flexibility of a concentrated solar power plant with hydrogen production,” Sol. Energy, vol. 247, no. 19, pp. 158–170, Nov. 2022. doi: 10.1016/j.solener.2022.10.011. [Google Scholar] [CrossRef]
6. M. Emmanuel, K. Doubleday, B. Cakir, M. Marković, and B. M. Hodge, “A review of power system planning and operational models for flexibility assessment in high solar energy penetration scenarios,” Sol. Energy, vol. 210, no. 4, pp. 169–180, 2020. doi: 10.1016/j.solener.2020.07.017. [Google Scholar] [CrossRef]
7. Q. Abbas, M. Mirzaeian, M. R. Hunt, P. Hall, and R. Raza, “Current state and future prospects for electrochemical energy storage and conversion systems,” Energies, vol. 13, no. 21, pp. 5847, 2020. doi: 10.3390/en13215847. [Google Scholar] [CrossRef]
8. O. Schmidt, A. Hawkes, A. Gambhir, and I. Staffell, “The future cost of electrical energy storage based on experience rates,” Nat. Energy, vol. 2, no. 8, pp. 17110, Jul. 2017. doi: 10.1038/nenergy.2017.110. [Google Scholar] [CrossRef]
9. J. Sun, J. Liu, Y. Wang, H. Yuan, and Z. Yan, “Development status, policy, and market mechanisms for battery energy storage in the US, China, Australia, and the UK,” J. Renew. Sustain. Energy, vol. 15, no. 2, pp. 024101, Mar. 2023. doi: 10.1063/5.0146184. [Google Scholar] [CrossRef]
10. L. Tronchin, M. Manfren, and B. Nastasi, “Energy efficiency, demand side management and energy storage technologies—A critical analysis of possible paths of integration in the built environment,” Renew. Sustain. Energ. Rev., vol. 95, pp. 341–353, Nov. 2018. doi: 10.1016/j.rser.2018.06.060. [Google Scholar] [CrossRef]
11. J. Torres, P. Moreno-Torres, G. Navarro, M. Blanco, and M. Lafoz, “Fast energy storage systems comparison in terms of energy efficiency for a specific application,” IEEE Access, vol. 6, pp. 40656–40672, 2018. doi: 10.1109/ACCESS.2018.2854915. [Google Scholar] [CrossRef]
12. D. Zhao, H. Wang, J. Huang, and X. Lin, “Time-of-use pricing for energy storage investment,” IEEE Trans. Smart Grid, vol. 13, no. 2, pp. 1165–1177, 2022. doi: 10.1109/TSG.2021.3136650. [Google Scholar] [CrossRef]
13. J. Xiao, G. He, S. Fan, and Z. Li, “Substitute energy price market mechanism for renewable energy power system with generalized energy storage,” Appl. Energy, vol. 328, pp. 120219, Dec. 2022. doi: 10.1016/j.apenergy.2022.120219. [Google Scholar] [CrossRef]
14. M. Jafari, A. Botterud, and A. Sakti, “Decarbonizing power systems: A critical review of the role of energy storage,” Renew. Sustain. Energ. Rev., vol. 158, no. 360, pp. 112077, Apr. 2022. doi: 10.1016/j.rser.2022.112077. [Google Scholar] [CrossRef]
15. A. Sánchez, Q. Zhang, M. Martín, and P. Vega, “Towards a new renewable power system using energy storage: An economic and social analysis,” Energy Convers. Manag., vol. 252, pp. 115056, Jan. 2022. doi: 10.1016/j.enconman.2021.115056. [Google Scholar] [CrossRef]
16. Y. Wang et al., “Research on planning optimization of integrated energy system based on the differential features of hybrid energy storage system,” J. Energy Storage, vol. 55, no. 2, pp. 105368, Nov. 2022. doi: 10.1016/j.est.2022.105368. [Google Scholar] [CrossRef]
17. B. K. Das, R. Hassan, M. S. Islam, and M. Rezaei, “Influence of energy management strategies and storage devices on the techno-enviro-economic optimization of hybrid energy systems: A case study in Western Australia,” J. Energy Storage, vol. 51, no. 1, pp. 104239, Jul. 2022. doi: 10.1016/j.est.2022.104239. [Google Scholar] [CrossRef]
18. F. J. de Sisternes, J. D. Jenkins, and A. Botterud, “The value of energy storage in decarbonizing the electricity sector,” Appl. Energy, vol. 175, no. 2, pp. 368–379, Aug. 2016. doi: 10.1016/j.apenergy.2016.05.014. [Google Scholar] [CrossRef]
19. Y. Wang, A. Gu, and A. Zhang, “Recent development of energy supply and demand in China, and energy sector prospects through 2030,” Energy Policy, vol. 39, no. 11, pp. 6745–6759, Nov. 2011. doi: 10.1016/j.enpol.2010.07.002. [Google Scholar] [CrossRef]
Cite This Article
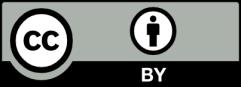
This work is licensed under a Creative Commons Attribution 4.0 International License , which permits unrestricted use, distribution, and reproduction in any medium, provided the original work is properly cited.