Open Access
ARTICLE
Research on Optimal Configuration of Energy Storage in Wind-Solar Microgrid Considering Real-Time Electricity Price
1 Electric Power Research Institute, State Grid Gansu Electric Power Company, Lanzhou, 730070, China
2 School of New Energy and Power Engineering, Lanzhou Jiaotong University, Lanzhou, 730000, China
* Corresponding Author: Zhenzhen Zhang. Email:
Energy Engineering 2023, 120(7), 1637-1654. https://doi.org/10.32604/ee.2023.026942
Received 05 October 2022; Accepted 16 December 2022; Issue published 04 May 2023
Abstract
Capacity allocation and energy management strategies for energy storage are critical to the safety and economical operation of microgrids. In this paper, an improved energy management strategy based on real-time electricity price combined with state of charge is proposed to optimize the economic operation of wind and solar microgrids, and the optimal allocation of energy storage capacity is carried out by using this strategy. Firstly, the structure and model of microgrid are analyzed, and the output model of wind power, photovoltaic and energy storage is established. Then, considering the interactive power cost between the microgrid and the main grid and the charge-discharge penalty cost of energy storage, an optimization objective function is established, and an improved energy management strategy is proposed on this basis. Finally, a physical model is built in MATLAB/Simulink for simulation verification, and the energy management strategy is compared and analyzed on sunny and rainy days. The initial configuration cost function of energy storage is added to optimize the allocation of energy storage capacity. The simulation results show that the improved energy management strategy can make the battery charge-discharge response to real-time electricity price and state of charge better than the traditional strategy on sunny or rainy days, reduce the interactive power cost between the microgrid system and the power grid. After analyzing the change of energy storage power with cost, we obtain the best energy storage capacity and energy storage power.Keywords
Nomenclature
Charging power of the energy storage | |
Charging efficiency | |
Discharging efficiency | |
Area of photovoltaic panels | |
Photovoltaic conversion efficiency | |
Solar irradiance | |
Air density | |
Area swept by the blades of the wind turbine | |
Wind energy utilization efficiency | |
Wind speed in front of the wind rotor | |
Power sold to the grid | |
Selling price | |
Electricity purchased from the main grid | |
Purchase electricity prices | |
Interactive power between microgrid and the main network | |
Maximum transmission line power |
In recent years, renewable energy sources such as PVs and wind power have rapidly emerged in the field of microgrids, but with the continuous expansion of power generation capacity, the randomness and volatility of their output have greatly hindered the progress of this field [1]. In order to improve power quality and meet the basic needs of electrical equipment or grid connection, new energy power generation is applied as a dispatchable power source, and at the same time, energy storage is used to smooth power fluctuations and maintain voltage stability. However, the cost performance of energy storage systems is currently low and it has a limited operating cycle, so under the condition of stable operation of the microgrid, it is of great significance to reasonably configure and optimize the energy storage capacity [2].
For the capacity configuration of energy storage, there have been relevant researches at home and abroad with various methods. Reference [3] established a multi-type hybrid energy storage model based on power output constraints and energy storage economy. Compared with a single energy storage system, it is confirmed that the hybrid energy storage system has obvious advantages in terms of stability and economy. Reference [4] evaluated the energy utilization rate and load loss under the condition of ensuring the minimum total net present value cost, and establishes a hybrid energy storage system containing hydrogen energy storage and storage battery to reduce the operating cost of the microgrid. In the literature [5], under the premise of ensuring the lowest power transaction cost and energy storage investment and operation cost of the hybrid energy storage system, the discrete Fourier transform was used to decompose the original power, and the charging and discharging power of the system is optimized. Reference [6] established an energy storage capacity allocation model by minimizing the initial investment of energy storage and tie line fluctuations. Reference [7] analyzed the characteristics of distributed power generation and the optimization objective function model, and quantitatively evaluates the economic and technical goals of the microgrid, which effectively realizes the multi-objective optimal operation of the microgrid. Reference [8] established a microgrid under the isolated grid operation mode. The economic dispatch strategy and dispatch model of the system are solved by an improved genetic algorithm, and the cogeneration of the microgrid system is realized. Literature [9] proposed a hierarchical control strategy, which can meet the requirements of load power and ensure power quality. Literature [10] proposed a multi-agent system-based bidding optimization strategy for microgrids, which determines the cost of power generation from the system level of the microgrid. have a discussion. The hybrid energy storage system in the literature [11] used wavelet decomposition algorithm to decouple out the battery and supercapacitor components, and the energy storage components are the mean, variance, fluctuation range and number of fluctuation points are used as the indicators to analyze the fluctuation quantity at different confidence levels and capacities. The mean, variance, fluctuation range and number of fluctuation points are used as indicators to analyze the smoothing effect of the hybrid energy storage system. The mean, variance, fluctuation range and number of fluctuation points are used as indicators to analyze the smoothing effect of the hybrid energy storage system. The literature [12,13] considered the problem of the battery’s own operating characteristics limitations and proposed a battery capacity determination method based on the internal characteristics of the battery. The method of determining the battery capacity based on the internal characteristics of the battery is proposed. Three different optimization schemes are established according to the optimization objectives and constraints. The literature [14,15] based According to the characteristics of combined PV system-energy storage operation, considering the operation process the dynamic change process of energy storage energy, and the technical characteristics of energy storage unit The technical characteristics of the energy storage unit are used as constraints, and a joint allocation method of PV and energy storage capacity is proposed with the load shortage rate and energy spillover ratio as the assessment indicators. The joint allocation method of PV and energy storage capacity is proposed with the technical characteristics of energy storage unit as the constraint. Under the given case the capacity allocation of three types of energy storage cells is calculated separately under the given case conditions. Under the given case conditions, the capacity allocation of three types of storage cells is calculated separately, and the optimal photovoltaic storage capacity is calculated with the objective of minimizing the initial investment. The optimal photovoltaic storage capacity configuration is calculated with the objective of minimizing the initial investment. In the literature [16], a compromise approach was proposed to achieve the maximum utilization of wind power and the minimum cost of energy storage devices with the goal of smoothing the power output of wind power. In the literature [17], a battery storage capacity optimization model that integrates wind power scheduling power optimization and variable lifetime characteristics was proposed with the objective of maximizing the annual return of the combined wind storage system.
Most of the above methods start from improving hybrid energy storage and dispatching strategies, and have achieved good results in the optimization of stability and economy [18,19]. However, few articles have researched the management strategy of real-time electricity price on the optimal configuration of energy storage. Traditional energy management strategies only consider the state of charge (SOC) of energy storage, and do not consider the constraints of electricity prices in this period. As long as the state of charge of the battery is within the specified value, it can be charged and discharged, which increases the frequent fluctuations of the state of charge, resulting in a decrease in the service life of energy storage, thus causing an increase in the total cost of electricity [20]. In addition, the energy storage system only charges when the new energy output is greater than the load demand and meets the state of charge range. The impact of real-time electricity prices on energy management and energy storage optimization is not considered, and the cost of energy storage configuration cannot be reduced by the difference between peak and valley electricity prices and lost some economic benefits [21].
Based on the above research, an improved energy management strategy considering real-time electricity price combined with state of charge is proposed for the optimal configuration of wind-solar storage microgrid energy storage system, and solved by linear programming [22]. Taking cloudy and sunny days in a certain area as typical representative days, the optimal allocation results of traditional energy management strategies and improved energy management strategies on wind [23,24], solar storage microgrids under two scenarios were analyzed. It has been verified that the improved energy management strategy proposed in this paper can use the peak-valley electricity price to reduce the interactive power cost between the microgrid and the main network to a certain extent, and overcome the shortcomings of the battery weakening its role according to the fixed charging and discharging rules [25,26]. On the basis of ensuring stability, the purpose of maximizing economic benefits is achieved [27,28].
2 Wind-Solar Storage Microgrid System Structure and Power Generation Model
2.1 Wind-Solar Storage Microgrid System Structure
The wind-solar-storage microgrid system is mainly composed of wind power system, PV system, energy storage system, energy management system and energy conversion device [10], as shown in Fig. 1.
Figure 1: Wind-solar storage microgrid system structure
2.2 Energy Storage Battery Model
The remaining power EB (t) of the energy storage battery at time t depends on the remaining power at the previous moment, the charging and discharging efficiency of the battery, and the charging and discharging power at this moment. When the energy storage battery is charged (PB(t) < 0), the remaining power can be expressed by Eq. (1):
When the energy storage battery is discharged (PB(t) > 0), the remaining power can be expressed by Eq. (2):
In the formula:
To simplify PV generation, the PV output power model is defined as:
The actual output active power of the wind turbine is shown in Eq. (4):
3 Energy Storage Configuration Optimization Model
The microgrid has the smallest electricity cost in a day. The daily electricity cost when the microgrid is connected to the grid includes the electricity cost purchased by the microgrid from the main grid and the electricity revenue sold to the main grid and battery charging and discharging costs. Therefore, the electricity cost function of the microgrid in one day can be expressed as:
wherein: f is the electricity cost.
After the optimization objective function is determined, certain constraints need to be met. According to the established economic and power supply reliability objective functions, the constraints are set as wind power, PV power generation output power constraints, voltage constraints, energy storage charge and discharge constraints, and capacity constraints. and load power balance constraints [13].
(1) Voltage constraints
(2) Energy storage system charge and discharge power constraints
(3) Charge and discharge state transition constraints
In the formula:
(4) Power load balancing constraints
In the formula:
(5) SOC constraints
(6) Transmission line power constraints
The interactive power range between the microgrid and the main network is within the power limit of the transmission line to ensure the safe operation of the system.
4 Real-Time Electricity Price Combined with State-of-Charge Energy Management Strategy
In order to ensure the economy of microgrid operation, the relationship between the real-time electricity price of micro-source and the real-time electricity price of the main grid needs to be compared in the grid-connected operation control strategy. After reviewing the data, it was found that the wind photovoltaic price was slightly higher than the minimum electricity price of the grid, 17 Cents/kWh, and did not change much with time. In order to simplify the model, this paper sets the wind and photovoltaic price as a constant, and the purchase and sale price of the main network is the same. In order to reduce the number of battery charge and discharge, improve battery service life, realize peak-valley electricity price arbitrage, and reduce costs, this paper proposes an improved energy management strategy based on real-time electricity price combined with charging state. Adopt a strategy that combines the state of charge with the price of electricity. Because the grid electricity price is generally low when the wind and solar are strong, and high when the wind and solar are insufficient. For microgrids, this paper sets the idea of selling electricity to the main grid as much as possible during periods of high electricity prices, and self-meeting demand as much as possible during periods of low electricity prices. It can prevent frequent charging and discharging of batteries, extend service life, and reduce the interactive power consumption cost between the microgrid system and the main network through peak-valley electricity prices.
During the energy optimization process, the difference between the electric energy provided by the distributed power source and the electric energy required by the load is shown in Eq. (9).
According to the electricity price data of a certain place in Gansu, as shown in Fig. 3b, the electricity price is set to different time periods shown in Table 1. There are 2 periods of high electricity prices in the day, and the rest are normal periods.
Different management strategies are adopted for different electricity price periods. During periods of high electricity prices, the concrete management strategies adopted are shown in the Fig. 2 below.
Figure 2: Improve the logical flow of energy management strategies
When the grid electricity price is at a high level and the wind power and solar power in the microgrid are greater than the load power, priority is given to dispatching the energy storage device to sell the full power generation to the main grid until the minimum value of SOC is reached, while the excess wind energy and photovoltaic power are also connected to the grid to sell electricity until the power limit of the transmission line is reached; Energy greater than the power of the transmission line charges idle batteries, and eventually excess power is discarded [28]. If wind power and solar power generation are less than the load power, the energy storage device generates electricity at full power to meet the load until the minimum value of SOC is reached, excess wind and solar energy can be sold to the main grid, and when the load demand cannot be met, the final dispatch purchases power from the grid to meet the load demand.
When the grid electricity price is in the normal period and the wind power and solar power generation power is greater than the load power, the energy storage is prioritized to charge until the maximum value of the SOC, and the excess power can be sold to the grid; If wind and solar power generation is less than the load power, the main grid is dispatched to meet the charging of the load and energy storage up to the maximum value of the SOC.
A summary of the decision variables and their status is shown in Table 2.
The physical model of the wind-solar-storage microgrid is built in MATLAB/Simulink, and the bus voltage reference is 5000 V. The rated power of the wind and the PV are both 450 kW. The battery state of charge limits is SOCmax = 0.8, SOCmin = 0.2,
In the parameter settings of Simulink simulation model, the photovoltaic panel area is 2500 square meters, the photoelectric conversion efficiency is 0.3, the wind power conversion efficiency is 0.3, the upper and lower limits of state of charge are 0.8 and 0.2, the initial battery rated capacity is 2500 kWh, the diameter of wind blades is 18 m, the battery penalty factor is 0.45 and the wind speed range of the fan is shown.
Under the sunny and cloudy scenarios, the optimal operation and economic costs of microgrid energy storage under the control of traditional energy management strategy and improved energy management strategy were analyzed, and the energy storage capacity under the improved strategy was obtained.
5.1 Comparison of Two Energy Management Strategies on Sunny Day
(1) Traditional energy management strategies
It can be seen from Figs. 3a and 3b that the traditional heuristic EMS has no connection with the real-time electricity price for the charge and discharge management of the energy storage system, and the energy storage SOC is unchanged or slightly decreased during the high price period of the day, and it is in a state of not charging or slowly discharging. The charge and discharge state and power of energy storage completely depend on the difference between wind and energy and load in the microgrid, and the peak-valley difference of the grid electricity price cannot be used to reduce the cost.
Figure 3: (a) The output of each unit in the microgrid (b) Battery SOC and real-time electricity price
(2) Improve energy management strategies
It can be seen from Figs. 4a and 4b that the improved EMS strategy combined with real-time electricity price is significantly optimized for energy storage charging and discharging. In the two periods of high electricity prices defined earlier, the battery SOC dropped significantly and was in a state of rapid discharge.
Figure 4: (a) The output of each unit in the microgrid (b) Battery SOC and real-time electricity price
And observing the output of each unit of the system, it can be seen that in the two high electricity price time periods, the wind and solar power generation is less than the load demand, but the battery is full power generation, and under the premise of meeting the load, the surplus power is supplied to the grid, which better responds to the improved energy management strategy set above, realizes the arbitrage of peak and valley electricity prices, and reduces the interactive power cost between the microgrid and the main network. During the trough period of electricity prices, the main network can be dispatched as much as possible to meet the load of the microgrid and charge energy storage, which can accumulate electricity for the subsequent period of high electricity prices.
Comparing Figs. 3 and 4, it can be seen that the traditional EMS only calls the battery for charging when the photovoltaic output is greater than the load demand, while the EMS combined with real-time electricity price improvement can call the battery to charge in advance when the electricity price and load are low, and when the load and electricity price peak, it can reduce the grid power supply or supply power to the grid to achieve the goal of reducing the cost of electricity.
5.2 Comparison of Two Energy Management Strategies on Cloudy Day
(1) Traditional energy management strategies
It can be seen from Figs. 5a and 5b that cloudy days bring more uncertainty and random fluctuations to PV power generation, reducing PV output. Observing the optimization and coordination of energy storage by traditional EMS at this time, the SOC of the energy storage system fluctuates and decreases throughout the day, and is always lower than the initial SOC. Within 2 time periods of high electricity prices, the energy storage SOC remains almost constant. In the state of non-charging and non-discharging, the difference between the load demand and the PV output is completely supplied by the grid.
Figure 5: (a) The output of each unit in the microgrid (b) Battery SOC and real-time electricity price
(2) Improve energy management strategies
It can be seen from Figs. 6a and 6b that during the trough period of load and electricity price (2000 –12000 s), when the photovoltaic has not yet generated electricity, the EMS is improved to dispatch the power grid to supply power to the energy storage system, so that the SOC of the energy storage system rises to the constrained SOC. Within 2 time periods of high electricity prices, dispatching energy storage to run power generation at full power reduces the power supply from the grid to the microgrid, reduces the cost of electricity interaction between the microgrid and the grid, and makes better use of the benefits of the peak-to-valley difference in electricity prices. It can also be seen that the improved EMS has a good optimal control for the SOC of the energy storage, which reduces the frequent fluctuation of the SOC and reduces the life cycle cost of the energy storage.
Figure 6: (a) The output of each unit in the microgrid (b) Battery SOC and real-time electricity price
Observing SOC changes under improved energy management strategies on sunny and cloudy days, it was found to be almost identical. Analyzing the output of each unit in both cases, it is found that this is in line with the proposed strategy for improving energy management. During the first period of high electricity prices (0–0.2 × 104 s), the load demand is greater than the wind and solar output power, so the battery is prioritized to discharge at full power, and the load demand is met, and the excess wind and solar power is sold to the main network. Then comes the first normal electricity price period (0.2 × 104–1.5 × 104), the wind and solar power is not enough to meet the load demand, purchase the main grid power to meet the load and charge the battery at full power. In the second high electricity price period (1.5 × 104–2.5 × 104), similar to the situation in the first high electricity price period, the load demand is not met, the battery is prioritized to meet the load, and the rest of the wind and solar are sold to the main network. At the end of this period, although the state of charge of the battery has not reached the minimum, the electricity price returns to normal and the discharge still stops. The rest of the tariff periods are similar to those described above and will not be repeated here. It can be seen from the analysis that the real-time electricity price combined with the state-of-charge EMS has a strong control effect on the microgrid and the battery, and the robustness is nice.
It can be seen from Fig. 7a that on sunny days, under the traditional heuristic EMS, combined with the improved EMS of real-time electricity price and the three modes without energy storage, the electricity cost of the traditional EMS is 542.88 $, and the cost of the improved EMS is 381.51 $, the maximum cost without energy storage is 601.25 $. Using the improved EMS combined with real-time electricity price, compared with the traditional EMS, the electricity cost is reduced by 29.73%.
Figure 7: (a) The cumulative cost on sunny day in three ways (b) The cumulative cost on cloudy day in three ways
It can be seen from Fig. 7b that on cloudy days, the electricity costs of the microgrid in the three cases of traditional heuristic EMS, improved EMS and no energy storage are 790.68 $, 628.80 $ and 836.72 $, respectively. Compared with the traditional EMS, the use of the improved EMS reduces the electricity cost by 20.47%.
5.3 Optimal Configuration of the Battery
In microgrids, the power and capacity of energy storage configuration are related to economic costs. This section optimizes battery configuration costs by adding the objective function described above. Taking the rated power of the battery configuration as the decision-making variable, the maximum operating time of 2 or 4 h stipulated by the policy of Gansu Province was selected for optimal configuration. The initial configuration cost is 200 $/kWh, which translates to 0.055 $ per day based on the maximum battery life of 10 years. The microgrid cost explained in the previous section plus the initial configuration cost of energy storage is the total cost, and the total cost of the initial energy storage size is shown in Table 4. Under the proven and improved energy management strategy, the optimization results of energy storage configuration of 2 and 4 h under sunny and cloudy weather scenarios are analyzed. The results are shown in Figs. 8 and 9.
Figure 8: (a) Optimized battery configuration on sunny days (b) Optimized battery configuration on cloudy days
Figure 9: (a) Optimized battery configuration on sunny days (b) Optimized battery configuration on cloudy days
As can be seen from Figs. 8 and 9, under the improved energy management strategy, when the full power run time of the battery is set to 2 h, the cost difference between sunny and cloudy energy storage configurations is large, but the optimal energy storage power is the same as 225 kW. On a sunny day, configuring a 225 kW/450 kWh battery in a microgrid can minimize the total operating costs of the microgrid at 491.87 $. On cloudy days, distributing 225 kW/450 kWh of energy storage in the microgrid can minimize the total cost of the microgrid at 739.08 $. When the full power run time of the battery is set to 4 h, on sunny days, configuring a 150 kW/600 kWh battery in the microgrid can minimize the total operating costs of the microgrid at 487.32 $. On cloudy days, distributing200 kW/800 kWh of energy storage in the microgrid can minimize the cost of the microgrid at 734.59 $.
The comparison chart and table show that whether on sunny or cloudy days, the cost of configuring a 4-h battery is lower than that of a 2-h battery, and it is also lower than the cost of the initial energy storage size. Although the initial capacity cost of configuring a 2-h battery is lower, the cost of interacting with electricity due to short operating time in the microgrid in this study is greatly increased. Configuring a 4-h battery can well meet the time requirements of the microgrid to respond to real-time electricity prices. Considering the lack of clouds and rain in Gansu Province, a 150 kW/600 kW battery was selected as the optimal configuration for microgrid energy storage.
Aiming at the optimization problem of economic operation in wind-solar microgrid, this paper establishes a model, takes the interactive electricity cost of microgrid and main network as the objective function, proposes an improved energy management strategy based on real-time electricity price combined with state of charge, and compares and analyzes the optimization configuration results under sunny and cloudy days. The following conclusions are drawn:
(1) In the wind-solar-storage microgrid, the real-time electricity price combined with the state-of-charge energy management strategy can coordinate the random fluctuations of wind power, photovoltaics and loads, and can more intuitively reflect the optimal adjustment sequence of each micro-source, and significantly improve the overall economic benefits of the microgrid;
(2) When the light conditions are insufficient, improving the energy management strategy can optimize the charging and discharging of the energy storage system, reduce the frequent fluctuations of the state of charge of the energy storage, overcome the shortcomings of the battery that weakens its function according to the fixed charging and discharging rules, and realize the battery’s effect on the main network.
Funding Statement: This paper is a phased achievement of Gansu Province’s Major Science and Technology Project (W22KJ2722005) “Research on Optimal Configuration and Operation Strategy of Energy Storage under “New Energy + Energy Storage” Mode”.
Conflicts of Interest: The authors declare that they have no conflicts of interest to report regarding the present study.
References
1. Zhang, K., Qiu, X. Y., Liu, M. Y. (2020). Hybrid energy storage capacity allocation scheme based on spectrum analysis and moving average filtering. Electrical Measurement & Instrumentation, 57(19), 51–57. [Google Scholar]
2. Zhang, X. Y., Zhang, J. C., Wang, N. (2018). Coordinated control of hybrid energy storage system based on variational mode decomposition. Electric Power, 51(9), 165–173. [Google Scholar]
3. Xie, Y. Z., Lu, J. P., Weng, Z. L. (2016). Optimal configuration of composite energy storage system to improve output power characteristics of wind power. Power System Technology, 40(7), 2052–2058. [Google Scholar]
4. Li, Y. Z., Guo, X. J., Dong, H. Y. (2020). Capacity optimization of wind/solar/storage microgrid hybrid energy storage system. Proceedings of the Chinese Society of Universities for Electric Power System and Automation, 32(6), 123–128. [Google Scholar]
5. Zhong, G. B., Wu, T., Zeng, J. (2018). Optimal allocation of hybrid energy storage capacity in active distribution network based on discrete Fourier transform. Electric Power Construction, 39(8), 85–93. [Google Scholar]
6. Li, J. L., Guo, B. Q., Niu, M. (2018). Optimal allocation strategy for energy storage capacity of wind and solar storage systems. Transactions of China Electrotechnical Society, 33(6), 1189–1196. [Google Scholar]
7. Xing, H., Lin, Z., Fu, M. (2017). Distributed algorithm for dynamic economic power dispatch with energy storage in smart grids. IET Control Theory and Applications, 11(11), 1813–1821. https://doi.org/10.1049/iet-cta.2016.1389 [Google Scholar] [CrossRef]
8. Thale, S. S., Agarwal, V. (2015). Controller area network assisted grid synchronization of a microgrid with renewable energy sources and storage. IEEE Transactions on Smart Grid, 7(3), 1–3. [Google Scholar]
9. Lu, L. M., Chu, G. W., Zhang, T. (2020). Optimal configuration of energy storage in microgrid based on improved multi-objective particle swarm algorithm. Power System Protection and Control, 48(15), 116–124. [Google Scholar]
10. Su, H., Zhang, J. C., Feng, D. H. (2019). Modular hybrid energy storage system and its energy management strategy. Electric Power Automation Equipment, 39(1), 127–133, 140. [Google Scholar]
11. Li, X., Zhang, J. C., Wang, N. (2018). Research on capacity allocation method of supercapacitor-flywheel-battery hybrid energy storage system. Electric Power, 51(11), 117–124. [Google Scholar]
12. Li, L. L., Wang, X., Lang, Y. B. (2019). Capacity optimization configuration of microgrid composite energy storage system based on improved w-hale algorithm. Electrical Measurement & Instrumentation, 56(16), 104–110. [Google Scholar]
13. Mao, X. M., Chen, S., Wu, J. K. (2015). Optimal dispatching of microgrid containing battery under time-of-use price mechanism. Power System Technology, 39(5), 1192–1197. [Google Scholar]
14. Meng, J. H., Peng, J. L., Wang, Y. (2020). Improved flexible virtual inertial control considering energy storage state of charge and frequency recovery characteristics. Electric Power Automation Equipment, 40(6), 100–107. [Google Scholar]
15. Zhu, Y. Q., Liu, K., Zhang, Q. (2019). Capacity configuration of interconnected converters in AC-DC hybrid microgrid considering energy storage system dispatching. Electric Power Construction, 40(10), 84–93. [Google Scholar]
16. Wang, C. S., Yang, Z. G., Wang, S. X. (2010). Analysis of structural characteristics and control approaches of experimental microgrid systems. Automation of Electric Power Systems, 34(1), 99–105. [Google Scholar]
17. Li, H., Huang, Y. M., Ma, F. (2017). Coordinated control strategy of hybrid energy storage system based on state of charge. Electric Power, 50(1), 158–163. [Google Scholar]
18. Li, L. (2011). Study of economic operation in microgrid. Beijing, China: North China Electric Power University. [Google Scholar]
19. Chang, K., Zhou, T., Zhang, H., Liu, S. F. (2022). Optimization of energy storage battery participation in grid secondary frequency regulation parameters. Electronic Design Engineering, 30(2), 64–68. [Google Scholar]
20. Shi, Q. J., Jiang, Q. Y. (2013). Real-time optimal energy dispatch for microgrid with battery storage. Electric Power Automation Equipment, 33(5), 76–82. [Google Scholar]
21. Hong, B., Guo, L., Wang, C. S. (2013). Model and method of dynamic multi-objective optimal dispatch for microgrid. Electric Power Automation Equipment, 33(3), 100–107. [Google Scholar]
22. Ma, S. L., Jian, X. P., Ma, H. M. (2014). Capacity configuration of the hybrid energy storage system for wind power smoothing. Power System Protection and Control, 42(8), 108–114. [Google Scholar]
23. Zhu, L., Yan, Z., Yang, X. (2012). Optimal configuration of battery capacity in microgrid composed of wind power and photovoltaic generation with energy storage. Power System Technology, 36(12), 26–31. [Google Scholar]
24. Yi, L., Lou, S. H., Wu, Y. W. (2015). Optimal battery capacity based on lifetime predication for improving the schedulability of the wind power. Transactions of China Electrotechnical Society, 30(15), 53–59. [Google Scholar]
25. Li, Q., Yuan, Y., Tan, D. Z. (2010). Progress on application of energy storage technology in wind power integration. Journal of Hohai University, 38(1), 115–122. [Google Scholar]
26. Tian, J., Zhu, Y. Q., Chen, C. H. (2010). Application of energy storage technologies in distributed generation. Electrical Engineering, 8, 28–32. [Google Scholar]
27. Huang, J. Y., Li, X. R., Cao, Y. J. (2015). Capacity allocation of energy storage system considering its action moment and output depth in rapid frequency regulation. Transactions of China Electrotechnical Society, 30(12), 454–464. [Google Scholar]
28. Dong, H. Y., Chu, Y. T., Yu, Y. T. (2018). Operation optimization of microgrid system with battery under real-time electricity price theory. Acta Energiae Solaris Sinica, 39(10), 2831–2842. [Google Scholar]
Cite This Article
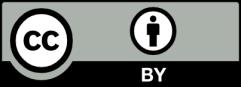
This work is licensed under a Creative Commons Attribution 4.0 International License , which permits unrestricted use, distribution, and reproduction in any medium, provided the original work is properly cited.