Open Access
EDITORIAL
Assessment on Fault Diagnosis and State Evaluation of New Power Grid: A Review
1 Faculty of Electric Power Engineering, Kunming University of Science and Technology, Kunming, 650500, China
2 School of Engineering, University of Lincoln, Lincoln, LN6 7TS, UK
3 The University of Hong Kong, Hong Kong, 999077, China
4 College of Information Science and Engineering, Northeastern University, Shenyang, 110819, China
5 Department of Electrical Engineering and Electronics, University of Liverpool, Liverpool, L69 3GJ, UK
* Corresponding Author: Jingbo Wang. Email:
(This article belongs to the Special Issue: Fault Diagnosis and State Evaluation of New Power Grid)
Energy Engineering 2023, 120(6), 1287-1293. https://doi.org/10.32604/ee.2023.027801
Received 15 November 2022; Accepted 05 January 2023; Issue published 03 April 2023
Abstract
This article has no abstract.Keywords
The proposal of the concept of “New Power System” aims to illustrate the transform direction of the traditional power system, acting as the development core of the future new power grid. To achieve this, the proposed strategic targets of “carbon neutralization and carbon peaking” must be implemented and insisted [1]. The core feature of the new power system is that renewable energy plays a leading role and becomes the main source of energy supply, meanwhile, the goal of green energy utilization has also been put forward on the agenda. Green energy utilization includes two aspects, one is the exploitation and promotion of various green energy technologies, and the other is the digitalization of energy management. Under this trend, stochastic and fluctuating energy sources such as wind power and photovoltaic power replace deterministic controllable power sources such as thermal power, bringing challenges to power grid regulation and dispatching, as well as flexible operation. The large-scale integration of renewable energy and increasingly high proportion of power electronic equipment tend to bring about fundamental changes in the operation characteristics, safety control, and production mode of the power system.
In the future, with the rising proportion of renewable energy consumption, a large number of distributed wind and solar power generation units, battery energy storage, and other equipment will emerge in the new power system [2]. Thus, the current power grid tends to transform from different aspects. First, the number of grid nodes will dramatically increase, such that the grid structure will become increasingly large and complex. Besides, the access mode of renewable energy changes the traditional mode of “generation-transmission-transformation-distribution” of traditional power system and also provides the possibility of distributed access on the load side, which makes it possible for the grid scale to explosively expand and the grid nodes to exponentially rise. Moreover, renewable energy power generation is characterized by randomness and intermittency. Therefore, when the proportion of renewable energy increases, frequent load transfer operations in a short time are highly likely to be a common foreseeable scenario in the future [3]. As a result, the system power flow will be redistributed in a short period of time, and some lines and equipment will even be overloaded. In particular, a large number of DC engineering equipment put into operation in the early stage already have a relatively long service life, such that whether they can effectively withstand the overload test is unknown due to their deteriorated performance. Therefore, it is necessary to combine digital technology and artificial intelligence to realize efficient, accurate, and timely perception, and intelligent decision-making of the status of power grid equipment [4], so as to meet the new challenges brought by the operation and maintenance requirements. At the same time, in order to improve the digital transformation of electrical tests under the new power grid and the standards of new products and technologies, various advanced fault tolerant and diagnosis strategies are imperative to ensure the stable, economic, and safe operation of the new power grid.
In general, new power gird owns high proportion of renewable energy and electronic equipment, in which all links of power supply, power grid, load, and energy storage are coupled through power flow and information flow, and interact with the outside world in terms of energy and digital. For such a complex system, the safe and stable operation is a thorny but critical problem, in which the research on fault diagnosis strategy can effectively ensure reliable fault diagnosis and in-depth evaluation, as well as considerably reduce the workload of personnel. The application of situation awareness technology can promote the integration of application functions of various systems of power grid automation, significantly improve the degree of system intelligence, effectively improve the operating efficiency of the entire power system, and ensure the safe and stable operation of the power grid. The powerful state prediction and decision support ability play critical roles in establishing smart grid. Thus, this work aims to briefly review the development status of state perception and fault diagnosis of new power grids, which tends to provide a guidance to promote up-to-date research and inspire promising ideas in related fields.
2 Fault Diagnosis of New Power Grid
With the continuous expansion of the scale of new power systems, especially under the large-scale integration of various renewable energy and power electronic equipment, ensuring the safe and stable operation of future power systems becomes a key issue [5]. In the case of power system accidents, accurate and rapid fault diagnosis is of great significance for power system restoration and power supply reliability improvement.
With the continuous promotion of the new power grid, the automation level of power generation enterprises' equipment has rapidly improved, and the structure has become more precise and complex, upon which a series of new requirements are put forward for fault diagnosis. During the specific engineering application, there are problems such as the diagnosis technology scheme is difficult to match the actual needs and even flawed diagnosis management schemes, which makes it difficult to effectively carry out intelligent fault diagnosis. Therefore, it is of great significance to improve the establishment of fault diagnosis evaluation model and propose the design scheme of the application platform to provide support for fault diagnosis management decision of power equipment in power system. At the same time, the new power system with large-scale renewable energy has employed a large number of new power system equipment, which formed the status of AC and DC coexistence, changed the operation mode of current power system, and blurred the fault characteristics. Hence, huge challenges are faced during the application of existing relay protection technology in new power systems. In the past 10 years, the research on fault diagnosis of power system has received widespread attention over the world [6]. In particular, artificial intelligence technology has been successfully applied to solve the problem of fault diagnosis, such as expert system, fuzzy theory, artificial neural network, random optimization technology, and logic method. In particular, the application of artificial intelligence technology in the fault diagnosis and control process of the new power system can not only improve the accuracy of fault diagnosis, but also enhance the performance of system control, ensure the stable operation of the new power system, and improve the system’s development degree.
Basically, the approaches of artificial intelligence technology in new power system fault diagnosis can be divided into three groups. Firstly, meta-heuristic algorithm, which is an algorithm that searches for the optimal solution by constantly updating and iterating the solutions [7]. It has the advantage of not relying on the accurate system model and has been widely used to solve high-dimensional complex problems. For power system fault diagnosis, meta-heuristic algorithm technology can be used to optimize and improve the fault diagnosis performance in an all-around way. Once a fault occurs in the system, the fault diagnosis task can be converted into an 0-1 type integer programming problem according to the relationship between the fault components and the specific protection actions [8], and the corresponding optimization algorithm can be constructed to effectively deal with the fault problems. For instance, reference [9] proposed an enhanced history driven differential evolution (HDDE) algorithm to realize online fault diagnosis of transmission lines, whose effectiveness has been validated under comprehensive case studies. If the protection software refuses to operate or the circuit breaker refuses to operate in the overall system, it means that the related diagnosis work is very ideal. Meanwhile, during the application of the meta-heuristic algorithm [10], problems should be considered from a global perspective, accurate diagnosis and analysis should be carried out, and appropriate digital models should be reasonably constructed.
The second group is artificial neural network. For its application, it is necessary to combine the characteristics and features of the new power system, obtain the corresponding knowledge points by reasonably setting the form of network language, and then secretly distribute to the network system to obtain the hidden knowledge points [11], such that an accurate memory of power system production can be generated and conveyed to the entire network. Besides, the noises of the system fault data information are removed during the implementation of artificial neural network, then the knowledge points needed in the fault diagnosis process are obtained. In particular, as one of the most important branches of intelligent technology development (i.e., expert system is the third stage of intelligent technology development, and artificial neural network technology is the fourth stage), expert system is also widely used in power system fault diagnosis. Expert system is a diagnostic method that uses computer models supported by expert reasoning methods to solve power system faults. An important feature of diagnosis method based on expert system is to reflect the protective behavior logic, circuit breaker and operation experience based on historical rules when establishing diagnostic analysis library. However, the current expert system based diagnosis methods still have some shortcomings, (a) the complicated process of creating a diagnostic analysis library and the integrity of the analysis library is not checked; (b) accurate information from the fault diagnosis analysis library is hard to acquire and validate; (c) it is difficult to maintain the fault diagnosis analysis library for large expert systems; (d) low reasoning speed of expert system in diagnosing complex faults. Compared with expert system, the diagnosis method based on artificial neural network owns stronger self-learning ability, fault tolerance ability, and robustness. When power system faults occur, different fault information will be generated due to the change of fault mode combination, thus the fault diagnosis problem can be regarded as a pattern recognition problem, which can be handled by the method of artificial neural network. This method is suitable for the cases where the logical representation between the fault type and the signal is complex and the expert experience is not adequate. In literature [12], a new convolutional neural network (CNN) based on LeNet-5 was proposed for fault diagnosis. Based on the conversion method converting signals into two-dimensional (2-D) images, the proposed method can extract the features of the converted 2-D images and eliminate the effect of handcrafted features. At the same time, the data information is transmitted to the system, so that the overall system state is changed, and the fault problems can be quickly removed [13]. Finally, it is necessary to build a corresponding knowledge based system. After the completion of fault diagnosis, the latest fault data information is stored in the system, which can not only quickly update the corresponding data information, but also provide more basis for subsequent fault diagnosis.
The last group is fuzzy logic, which can reduce the occurrence rate of system faults, and accurately analyze and mine potential faults, upon which accurate fault diagnosis results and data information can be obtained [14]. During the application of fuzzy algorithm, experience of fault handling and control is conveyed into the controller as input. On this basis, the corresponding fuzzy controller is studied and developed, such that the adaptability of the technology application is enhanced. Thus, the new power system fault problems can be automatically and intelligently identified, and the potential faults can be comprehensively excavated and cleared [15]. In particular, the accuracy of the detection work can be ensured based on fuzzy logic algorithm, upon which the fault maintenance personnel can more quickly identify and deal with the faults. In reference [16], an intuitionistic fuzzy spiking neural P (IFSNP) system has been developed via the combination of intuitionistic fuzzy logic and original spiking neural P systems. Compared with a common fuzzy set, intuitionistic fuzzy set can more finely describe the uncertainty thanks to its membership and non-membership degrees.
3 State Evaluation of New Power Grid
The remarkable feature of the new power grid is that renewable energy plays a dominant role in the power supply structure. Thus, the distributed generation mode of renewable energy will inevitably lead to the rapid growth of operating equipment in the new power system. Hence, the new power system will change from “source follows load” to the two-way coordination of “source load interaction”. It is crucial to precisely evaluate and perceive the state of massive equipment in the new power system with digital technology.
In particular, with the high proportion of renewable energy access and the rapid development of ultra-high voltage DC, the operation modes and fault patterns of new power grids are becoming increasingly complex. There are strong uncertainties on the generation side and load side. The rapid fluctuation of renewable energy and impact load lead to the rapid change of power grid operation mode under normal conditions, resulting in local fault affecting the overall situation. The existing dispatching monitoring system generally follows the processing mode of the monitoring information by the dispatching automation system, which adopts the direct and original data processing expression mode. Since this method only delivers information directly, the control personnel are submerged by a large amount of data, which is not conducive to effectively dispatching and monitoring the power grid. Hence, it is difficult to determine the cause of the accident in a short time. In addition, dispatching operators own different concerns about the power grid situation in different power grid operation states. Therefore, in the new power system environment, the state evaluation and perception technology own a solid data and technical foundation, but at the same time, how to deal with the strong uncertainty of the actual power grid, the overall fault, and the difference in the focus of the situation needs further research.
The status of power grid equipment is related to the safe and stable operation of the whole system, and the status recognition of power grid equipment under the new power system will face tests and requirements from reliability, accuracy, timeliness, and intelligence [17]. In order to realize the status evaluation and intelligent decision-making of electrical equipment in each link of the source network load storage, and solve the security problems caused by large-scale new energy access, an important prerequisite is the accurate measurement of equipment status. In other words, the acquisition and accumulation of basic data related to equipment status is the foundation [18]. After the construction of new power system, on the one hand, it is critical to determine how the state data of the original equipment can be accurately measured in the increasingly complex electrical environment; on the other hand, how to solve the deficiency that effective state measurement means are insufficient for a large number of power electronic equipment brought by the renewable energy access.
Firstly, the accurate measurement of the original equipment status data needs to be investigated [19]. At present, the equipment status is mainly determined by manually conducting live tests after the power grid equipment is detected as abnormal, whose fineness of this information collection method still has a lot of room for improvement. During the construction of the new power system, the total number of equipment will considerably increases and the layout tends to be more compact, thus the measurement difficulty is further increased and the real-time requirements for equipment status acquisition are gradually improved [20]. Therefore, it is necessary to study the intelligentization of measurement means, in which one of the directions is utilizing robots to replace manual work. In the future, robot technology exploration can be extended to other key equipment to achieve 24-hour real-time measurement of equipment status data. Through improving the intelligent level of operation and maintenance, the safety risks faced by personnel during on-site working can be effectively reduced. To exploit the monitoring approaches of power equipment status via the Internet of Things, literature [21] integrated work practice to analyze the data information, tasks, and technical methods of power equipment status monitoring, conducted detailed research on primary equipment and secondary equipment, and formulated the power equipment status information monitoring strategy and method based on the Internet of things.
In addition, it is necessary to solve the problem of state data collection of power electronic equipment [22]. The control of the system equipment level faces the challenges of high concurrency analysis and calculation caused by the expansion of power grid [23], as well as the high real-time analysis and calculation caused by explosive growth of system nodes and the complex system characteristics such as harmonics and overload. In response to this challenge, the integration of technologies in the fields of computer, information technology, and artificial intelligence with traditional electrical technologies can form a new intelligent method system to solve practical problems.
Under the targets of “carbon neutralization and carbon peaking”, the construction of the new power grid is an inevitable trend in the upgrading and development of traditional power grids. Compared with the traditional power grid, the new power grid owns higher requirements for flexibility, controllability, safety, and stability, and thus faces great challenges in ensuring the reliable operation of power equipment. At the same time, the new power grid is influenced by the randomness, volatility, and intermittency characteristics of renewable energy, such that its system regulation resource demand is large. Thus, major challenges in continuous and reliable power supply, power grid security and stability, and power generation are generated. Therefore, it is necessary to realize efficient, accurate, and timely state perception and intelligent decision-making of the power grid operation status, thus improving the real-time information acquisition and analysis capabilities, and enhancing the regulatory decision-making capabilities. At the same time, the development and application of advanced fault diagnosis strategies can not only effectively diagnose faults, but also intelligently and automatically control the system to improve the stability and reliability of the operation of the new power grid. As key technical supports, fault diagnosis and state perception will significantly improve the transparency level of the new power grid and ensure its safe operation after a high proportion of renewable energy integration. This work aims to analyze the main challenges that are encountered in the field of fault diagnosis and state evaluation of new power grid, and highlight the current state of technologies and applications. Hopefully, this review can act as one guidebook to encourage original contributions regarding promising developments in suitable technologies, ideas, and solutions for related research fields.
Funding Statement: The authors received no specific funding for this study.
Conflicts of Interest: The authors declare that they have no conflicts of interest to report regarding the present article.
References
1. Yang, P. J., Peng, S., Benani, N., Dong, L. Y., Li, X. M. et al. (2022). An integrated evaluation on China’s provincial carbon peak and carbon neutrality. Journal of Cleaner Production, 377, 134497. https://doi.org/10.1016/j.jclepro.2022.134497 [Google Scholar] [CrossRef]
2. Li, Y. H., Wang, R., Zhou, W. P., Gao, B. T. (2022). Evaluation of dispatching results of power system with high penetration of renewable energy based on Pythagorean fuzzy set and TOPSIS. Energy Reports, 8(1), 524–532. https://doi.org/10.1016/j.egyr.2022.08.134 [Google Scholar] [CrossRef]
3. Dai, Y. R., Zeng, Y. P. (2022). Optimization of CCHP integrated with multiple load, replenished energy, and hybrid storage in different operation modes. Energy, 260(1), 125129. https://doi.org/10.1016/j.energy.2022.125129 [Google Scholar] [CrossRef]
4. Utama, C., Meske, C., Schneider, J., Ulbrich, C. (2022). Reactive power control in photovoltaic systems through (explainable) artificial intelligence. Applied Energy, 328(3), 120004. https://doi.org/10.1016/j.apenergy.2022.120004 [Google Scholar] [CrossRef]
5. Ajagekar, A., You, F. Q. (2022). Quantum computing based hybrid deep learning for fault diagnosis in electrical power systems. Applied Energy, 303(6), 117628. https://doi.org/10.1016/j.apenergy.2021.117628 [Google Scholar] [CrossRef]
6. Vaish, R., Dwivedi, U. D., Tewari, S., Tripathi, S. M. (2021). Machine learning applications in power system fault diagnosis: Research advancements and perspectives. Engineering Applications of Artificial Intelligence, 106, 104504. https://doi.org/10.1016/j.engappai.2021.104504 [Google Scholar] [CrossRef]
7. Li, C. S., Xiong, G. J., Fu, X. F., Mohamed, A. W., Yuan, X. F. et al. (2022). Takagi-Sugeno fuzzy based power system fault section diagnosis models via genetic learning adaptive GSK algorithm. Knowledge-Based Systems, 255(4), 109773. https://doi.org/10.1016/j.knosys.2022.109773 [Google Scholar] [CrossRef]
8. Fan, Q. C., Yu, F., Xuan, M. (2021). Transformer fault diagnosis method based on improved whale optimization algorithm to optimize support vector machine. Energy Reports, 7(4), 856–866. https://doi.org/10.1016/j.egyr.2021.09.188 [Google Scholar] [CrossRef]
9. Zhao, J., Xu, Y., Luo, F., Dong, Z. Y., Peng, Y. (2014). Power system fault diagnosis based on history driven differential evolution and stochastic time domain simulation. Information Sciences, 275(5), 13–29. https://doi.org/10.1016/j.ins.2014.02.039 [Google Scholar] [CrossRef]
10. Du, R., Wei, X., Wang, X., Chen, S., Yuan, H. et al. (2021). A fault diagnosis model for proton exchange membrane fuel cell based on impedance identification with differential evolution algorithm. International Journal of Hydrogen Energy, 46(78), 38795–38808. https://doi.org/10.1016/j.ijhydene.2021.09.126 [Google Scholar] [CrossRef]
11. Xiong, G. J., Shi, D. Y., Chen, J. F., Zhu, L., Duan, X. Z. (2022). Divisional fault diagnosis of large-scale power systems based on radial basis function neural network and fuzzy integral. Electric Power Systems Research, 105(1), 9–19. https://doi.org/10.1016/j.epsr.2013.07.005 [Google Scholar] [CrossRef]
12. Wen, L., Li, X., Gao, L., Zhang, Y. (2018). A new convolutional neural network-based data-driven fault diagnosis method. IEEE Transactions on Industrial Electronics, 65(7), 5990–5998. https://doi.org/10.1109/TIE.2017.2774777 [Google Scholar] [CrossRef]
13. Dhibi, K., Mansouri, M., Bouzrara, K., Nounou, H., Nounou, M. (2022). Reduced neural network based ensemble approach for fault detection and diagnosis of wind energy converter systems. Renewable Energy, 194(3), 778–787. https://doi.org/10.1016/j.renene.2022.05.082 [Google Scholar] [CrossRef]
14. Montazeri-Gh, M., Yazdani, S. (2020). Application of interval type-2 fuzzy logic systems to gas turbine fault diagnosis. Applied Soft Computing, 96, 106703. https://doi.org/10.1016/j.asoc.2020.106703 [Google Scholar] [CrossRef]
15. Sarikh, S., Raoufi, M., Bennouna, A., Ikken, B. (2021). Characteristic curve diagnosis based on fuzzy classification for a reliable photovoltaic fault monitoring. Sustainable Energy Technologies and Assessments, 43(2), 100958. https://doi.org/10.1016/j.seta.2020.100958 [Google Scholar] [CrossRef]
16. Peng, H., Wang, J., Ming, J., Shi, P., Pérez-Jiménezet, M. J. et al. (2021). Fault diagnosis of power systems using intuitionistic fuzzy spiking neural P systems. IEEE Transactions on Smart Grid, 9(5), 4777–4784. https://doi.org/10.1109/TSG.2017.2670602 [Google Scholar] [CrossRef]
17. Ding, Y., Cui, W. Q., Zhang, S. J., Hui, H. X., Qiu, Y. Q. (2019). Multi-state operating reserve model of aggregate thermostatically-controlled-loads for power system short-term reliability evaluation. Applied Energy, 241, 46–58. https://doi.org/10.1016/j.apenergy.2019.02.018 [Google Scholar] [CrossRef]
18. Shao, Z. T., Zhai, Q. Z., Mao, Y. M., Guan, X. H. (2023). A method for evaluating and improving linear power flow models in system with large fluctuations. International Journal of Electrical Power & Energy Systems, 145(6), 108635. https://doi.org/10.1016/j.ijepes.2022.108635 [Google Scholar] [CrossRef]
19. Wang, N., Ni, L. L., Wang, A., Shan, H. S., Jia, H. Z. et al. (2022). High-efficiency photovoltaic-thermoelectric hybrid energy harvesting system based on functionally multiplexed intelligent thermal management. Energy Conversion and Management, 272(2), 116377. https://doi.org/10.1016/j.enconman.2022.116377 [Google Scholar] [CrossRef]
20. Chen, X. Y., Liang, W., Zhou, X. L., Jiang, D. C., Kui, X. Y. et al. (2021). An efficient transmission algorithm for power grid data suitable for autonomous multi-robot systems. Information Sciences, 572(3), 543–557. https://doi.org/10.1016/j.ins.2021.05.033 [Google Scholar] [CrossRef]
21. Long, L. (2021). Research on status information monitoring of power equipment based on Internet of Things. Energy Reports, 8(2), 281–286. https://doi.org/10.1016/j.egyr.2022.01.018 [Google Scholar] [CrossRef]
22. Ghasemi, A., Sedighizadeh, M., Fakharian, A., Nasiri, M. R. (2022). Intelligent voltage and frequency control of islanded micro-grids based on power fluctuations and communication system uncertainty. International Journal of Electrical Power & Energy Systems, 143(4), 108383. https://doi.org/10.1016/j.ijepes.2022.108383 [Google Scholar] [CrossRef]
23. Shams, M. H., Niaz, H., Hashemi, B., Liu, J. J., Siano, P. et al. (2021). Artificial intelligence-based prediction and analysis of the oversupply of wind and solar energy in power systems. Energy Conversion and Management, 250(10), 114892. https://doi.org/10.1016/j.enconman.2021.114892 [Google Scholar] [CrossRef]
Cite This Article
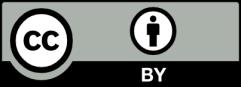
This work is licensed under a Creative Commons Attribution 4.0 International License , which permits unrestricted use, distribution, and reproduction in any medium, provided the original work is properly cited.