Open Access
ARTICLE
A Novel Ultra Short-Term Load Forecasting Method for Regional Electric Vehicle Charging Load Using Charging Pile Usage Degree
School of Automation, Wuhan University of Technology, Wuhan, 430070, China
* Corresponding Author: Jinrui Tang. Email:
Energy Engineering 2023, 120(5), 1107-1132. https://doi.org/10.32604/ee.2023.025666
Received 25 July 2022; Accepted 30 September 2022; Issue published 20 February 2023
Abstract
Electric vehicle (EV) charging load is greatly affected by many traffic factors, such as road congestion. Accurate ultra short-term load forecasting (STLF) results for regional EV charging load are important to the scheduling plan of regional charging load, which can be derived to realize the optimal vehicle to grid benefit. In this paper, a regional-level EV ultra STLF method is proposed and discussed. The usage degree of all charging piles is firstly defined by us based on the usage frequency of charging piles, and then constructed by our collected EV charging transaction data in the field. Secondly, these usage degrees are combined with historical charging load values to form the input matrix for the deep learning based load prediction model. Finally, long short-term memory (LSTM) neural network is used to construct EV charging load forecasting model, which is trained by the formed input matrix. The comparison experiment proves that the proposed method in this paper has higher prediction accuracy compared with traditional methods. In addition, load characteristic index for the fluctuation of adjacent day load and adjacent week load are proposed by us, and these fluctuation factors are used to assess the prediction accuracy of the EV charging load, together with the mean absolute percentage error (MAPE).Keywords
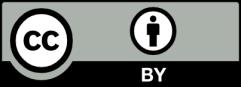