Open Access
ARTICLE
Identification of High-Risk Scenarios for Cascading Failures in New Energy Power Grids Based on Deep Embedding Clustering Algorithms
1 State Grid Shanxi Electric Power Research Institute, State Grid Shanxi Electric Power Co., Ltd., Taiyuan, 030001, China
2 School of Electrical and Electronic Engineering, North China Electric Power University, Beijing, 102206, China
* Corresponding Author: Ziqi Zhang. Email:
(This article belongs to the Special Issue: Fault Diagnosis and State Evaluation of New Power Grid)
Energy Engineering 2023, 120(11), 2517-2529. https://doi.org/10.32604/ee.2023.042633
Received 06 June 2023; Accepted 01 August 2023; Issue published 31 October 2023
Abstract
At present, the proportion of new energy in the power grid is increasing, and the random fluctuations in power output increase the risk of cascading failures in the power grid. In this paper, we propose a method for identifying high-risk scenarios of interlocking faults in new energy power grids based on a deep embedding clustering (DEC) algorithm and apply it in a risk assessment of cascading failures in different operating scenarios for new energy power grids. First, considering the real-time operation status and system structure of new energy power grids, the scenario cascading failure risk indicator is established. Based on this indicator, the risk of cascading failure is calculated for the scenario set, the scenarios are clustered based on the DEC algorithm, and the scenarios with the highest indicators are selected as the significant risk scenario set. The results of simulations with an example power grid show that our method can effectively identify scenarios with a high risk of cascading failures from a large number of scenarios.Keywords
Cite This Article
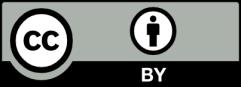
This work is licensed under a Creative Commons Attribution 4.0 International License , which permits unrestricted use, distribution, and reproduction in any medium, provided the original work is properly cited.