Open Access
ARTICLE
Multi-Criteria Decision-Making for Power Grid Construction Project Investment Ranking Based on the Prospect Theory Improved by Rewarding Good and Punishing Bad Linear Transformation
1 Guangdong Power Grid Co., Ltd., Guangzhou, 510410, China
2 Electric Power Development Research Institute, China Electricity Council (CEC), Beijing, 100053, China
* Corresponding Author: Mingrui Zhao. Email:
Energy Engineering 2023, 120(10), 2369-2392. https://doi.org/10.32604/ee.2023.028727
Received 04 January 2023; Accepted 23 March 2023; Issue published 28 September 2023
Abstract
Using the improved prospect theory with the linear transformations of rewarding good and punishing bad (RGPBIT), a new investment ranking model for power grid construction projects (PGCPs) is proposed. Given the uncertainty of each index value under the market environment, fuzzy numbers are used to describe qualitative indicators and interval numbers are used to describe quantitative ones. Taking into account decision-maker’s subjective risk attitudes, a multi-criteria decision-making (MCDM) method based on improved prospect theory is proposed. First, the [−1, 1] RGPBIT operator is proposed to normalize the original data, to obtain the best and worst schemes of PGCPs. Furthermore, the correlation coefficients between interval/fuzzy numbers and the best/worst schemes are defined and introduced to the prospect theory to improve its value function and loss function, and the positive and negative prospect value matrices of the project are obtained. Then, the optimization model with the maximum comprehensive prospect value is constructed, the optimal attribute weight is determined, and the PGCPs are ranked accordingly. Taking four PGCPs of the IEEERTS-79 node system as examples, an illustration of the feasibility and effectiveness of the proposed method is provided.Keywords
Cite This Article
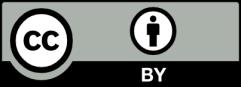
This work is licensed under a Creative Commons Attribution 4.0 International License , which permits unrestricted use, distribution, and reproduction in any medium, provided the original work is properly cited.