Open Access
ARTICLE
Deep Learning Network for Energy Storage Scheduling in Power Market Environment Short-Term Load Forecasting Model
1
State Grid Zhejiang Electric Power Co., Ltd., Hangzhou, 310007, China
2
Strategy and Development Research Center, Economic and Technical Research Institute, State Grid Zhejiang Electric Power Co.,
Hangzhou, 310000, China
3
Beijing Key Laboratory of New Energy Power and Low Carbon Development Research, North China University of Electric Power,
Beijing, 102206, China
* Corresponding Author: Sha Peng. Email:
Energy Engineering 2022, 119(5), 1829-1841. https://doi.org/10.32604/ee.2022.020118
Received 05 November 2021; Accepted 27 December 2021; Issue published 21 July 2022
Abstract
In the electricity market, fluctuations in real-time prices are unstable, and changes in short-term load are determined by many factors. By studying the timing of charging and discharging, as well as the economic benefits of energy storage in the process of participating in the power market, this paper takes energy storage scheduling as merely one factor affecting short-term power load, which affects short-term load time series along with time-of-use price, holidays, and temperature. A deep learning network is used to predict the short-term load, a convolutional neural network (CNN) is used to extract the features, and a long short-term memory (LSTM) network is used to learn the temporal characteristics of the load value, which can effectively improve prediction accuracy. Taking the load data of a certain region as an example, the CNN-LSTM prediction model is compared with the single LSTM prediction model. The experimental results show that the CNN-LSTM deep learning network with the participation of energy storage in dispatching can have high prediction accuracy for short-term power load forecasting.Keywords
Cite This Article
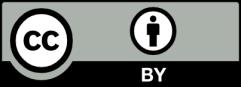
This work is licensed under a Creative Commons Attribution 4.0 International License , which permits unrestricted use, distribution, and reproduction in any medium, provided the original work is properly cited.