Open Access
ARTICLE
Production Dynamic Prediction Method of Waterflooding Reservoir Based on Deep Convolution Generative Adversarial Network (DC-GAN)
1
Cooperative Innovation Center of Unconventional Oil and Gas (Ministry of Education & Hubei Province), Yangtze University,
Wuhan, 430100, China
2
Key Laboratory of Drilling and Production Engineering for Oil and Gas, Wuhan, 430100, China
3
School of Petroleum Engineering, Yangtze University, Wuhan, 430100, China
* Corresponding Author: Xiang Rao. Email:
Energy Engineering 2022, 119(5), 1905-1922. https://doi.org/10.32604/ee.2022.019556
Received 29 September 2021; Accepted 22 December 2021; Issue published 21 July 2022
Abstract
The rapid production dynamic prediction of water-flooding reservoirs based on well location deployment has been the basis of production optimization of water-flooding reservoirs. Considering that the construction of geological models with traditional numerical simulation software is complicated, the computational efficiency of the simulation calculation is often low, and the numerical simulation tools need to be repeated iteratively in the process of model optimization, machine learning methods have been used for fast reservoir simulation. However, traditional artificial neural network (ANN) has large degrees of freedom, slow convergence speed, and complex network model. This paper aims to predict the production performance of water flooding reservoirs based on a deep convolutional generative adversarial network (DC-GAN) model, and establish a dynamic mapping relationship between well location deployment and output oil saturation. The network structure is based on an improved UNet framework. Through a deep convolutional network and deconvolution network, the features of input well deployment images are extracted, and the stability of the adversarial model is strengthened. The training speed and accuracy of the proxy model are improved, and the oil saturation of water flooding reservoirs is dynamically predicted. The results show that the trained DC-GAN has significant advantages in predicting oil saturation by the well-location employment map. The cosine similarity between the oil saturation map given by the trained DC-GAN and the oil saturation map generated by the numerical simulator is compared. In above, DC-GAN is an effective method to conduct a proxy model to quickly predict the production performance of water flooding reservoirs.Keywords
Cite This Article
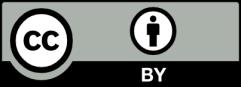
This work is licensed under a Creative Commons Attribution 4.0 International License , which permits unrestricted use, distribution, and reproduction in any medium, provided the original work is properly cited.