Open Access
ARTICLE
Bearing Fault Diagnosis Method of Wind Turbine Based on Improved Anti-Noise Residual Shrinkage Network
Beijing Goldwind Science & Goldwind Huineng Technology Co., Ltd., Beijing, 100176, China
* Corresponding Author: Xiaolei Li. Email:
(This article belongs to the Special Issue: Advancements in Renewable Energy Systems with AI, Big Data, BlockChain, IoT, and Machine Learning Applications)
Energy Engineering 2022, 119(2), 665-680. https://doi.org/10.32604/ee.2022.019292
Received 14 September 2021; Accepted 22 November 2021; Issue published 24 January 2022
Abstract
Aiming at the difficulty of rolling bearing fault diagnosis of wind turbine under noise environment, a new bearing fault identification method based on the Improved Anti-noise Residual Shrinkage Network (IADRSN) is proposed. Firstly, the vibration signals of wind turbine rolling bearings were preprocessed to obtain data samples divided into training and test sets. Then, a bearing fault diagnosis model based on the improved anti-noise residual shrinkage network was established. To improve the ability of fault feature extraction of the model, the convolution layer in the deep residual shrinkage network was replaced with a Dense-Net layer. To further improve the anti-noise ability of the model, the first layer of the model was set as the Drop-block layer. Finally, the labeled data samples were used for training model and the trained model was applied to the test set to output the fault diagnosis results. The results showed that the proposed method could achieve the fault diagnosis of wind turbine bearing more accurately in the high noise environment through comparison and verification.Keywords
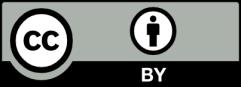