Open Access
ARTICLE
Electricity Demand Time Series Forecasting Based on Empirical Mode Decomposition and Long Short-Term Memory
1 Department of Mechanical and Energy Engineering, Purdue University, Indianapolis, USA
2 Department of Mechanical Engineering, School of Engineering, Aalto University, Espoo, Finland
* Corresponding Author: Behnam Talebjedi. Email:
Energy Engineering 2021, 118(6), 1577-1594. https://doi.org/10.32604/EE.2021.017795
Received 08 June 2021; Accepted 29 July 2021; Issue published 10 September 2021
Abstract
Load forecasting is critical for a variety of applications in modern energy systems. Nonetheless, forecasting is a difficult task because electricity load profiles are tied with uncertain, non-linear, and non-stationary signals. To address these issues, long short-term memory (LSTM), a machine learning algorithm capable of learning temporal dependencies, has been extensively integrated into load forecasting in recent years. To further increase the effectiveness of using LSTM for demand forecasting, this paper proposes a hybrid prediction model that incorporates LSTM with empirical mode decomposition (EMD). EMD algorithm breaks down a load time-series data into several sub-series called intrinsic mode functions (IMFs). For each of the derived IMFs, a different LSTM model is trained. Finally, the outputs of all the individual LSTM learners are fed to a meta-learner to provide an aggregated output for the energy demand prediction. The suggested methodology is applied to the California ISO dataset to demonstrate its applicability. Additionally, we compare the output of the proposed algorithm to a single LSTM and two state-of-the-art data-driven models, specifically XGBoost, and logistic regression (LR). The proposed hybrid model outperforms single LSTM, LR, and XGBoost by, 35.19%, 54%, and 49.25% for short-term, and 36.3%, 34.04%, 32% for long-term prediction in mean absolute percentage error, respectively.Keywords
Cite This Article
Citations
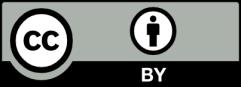
This work is licensed under a Creative Commons Attribution 4.0 International License , which permits unrestricted use, distribution, and reproduction in any medium, provided the original work is properly cited.