Open Access
ARTICLE
An Intelligent Diagnosis Method of the Working Conditions in Sucker-Rod Pump Wells Based on Convolutional Neural Networks and Transfer Learning
1 Shengli College China University of Petroleum, Dongying, 257061, China
2 China University of Petroleum (East China), Qingdao, 266580, China
* Corresponding Author: Ruichao Zhang. Email:
Energy Engineering 2021, 118(4), 1069-1082. https://doi.org/10.32604/EE.2021.014961
Received 11 November 2020; Accepted 12 December 2020; Issue published 31 May 2021
Abstract
In recent years, deep learning models represented by convolutional neural networks have shown incomparable advantages in image recognition and have been widely used in various fields. In the diagnosis of sucker-rod pump working conditions, due to the lack of a large-scale dynamometer card data set, the advantages of a deep convolutional neural network are not well reflected, and its application is limited. Therefore, this paper proposes an intelligent diagnosis method of the working conditions in sucker-rod pump wells based on transfer learning, which is used to solve the problem of too few samples in a dynamometer card data set. Based on the dynamometer cards measured in oilfields, image classification and preprocessing are conducted, and a dynamometer card data set including 10 typical working conditions is created. On this basis, using a trained deep convolutional neural network learning model, model training and parameter optimization are conducted, and the learned deep dynamometer card features are transferred and applied so as to realize the intelligent diagnosis of dynamometer cards. The experimental results show that transfer learning is feasible, and the performance of the deep convolutional neural network is better than that of the shallow convolutional neural network and general fully connected neural network. The deep convolutional neural network can effectively and accurately diagnose the working conditions of sucker-rod pump wells and provide an effective method to solve the problem of few samples in dynamometer card data sets.Keywords
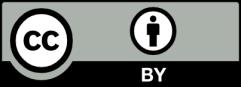