Open Access
ARTICLE
Long-Term Electricity Demand Forecasting for Malaysia Using Artificial Neural Networks in the Presence of Input and Model Uncertainties
1 Centre for Modelling and Simulation, Faculty of Engineering, Built Environment and Information Technology, SEGi University, Petaling Jaya, Malaysia
2 Mechanical Engineering Department, Faculty of Engineering, Built Environment and Information Technology, SEGi University, Petaling Jaya, Malaysia
3 Lee Kong Chian Faculty of Engineering and Science, UTAR, Kajang, Malaysia
4 Faculty of Civil Engineering, Universiti Teknologi MARA, Shah Alam, Malaysia
* Corresponding Author: Vin Cent Tai. Email:
(This article belongs to the Special Issue: The Role of Artificial Intelligence for Modeling and Optimizing the Energy Systems )
Energy Engineering 2021, 118(3), 715-725. https://doi.org/10.32604/EE.2021.014865
Received 04 November 2020; Accepted 29 January 2021; Issue published 22 March 2021
Abstract
Electricity demand is also known as load in electric power system. This article presents a Long-Term Load Forecasting (LTLF) approach for Malaysia. An Artificial Neural Network (ANN) of 5-layer Multi-Layered Perceptron (MLP) structure has been designed and tested for this purpose. Uncertainties of input variables and ANN model were introduced to obtain the prediction for years 2022 to 2030. Pearson correlation was used to examine the input variables for model construction. The analysis indicates that Primary Energy Supply (PES), population, Gross Domestic Product (GDP) and temperature are strongly correlated. The forecast results by the proposed method (henceforth referred to as UQ-SNN) were compared with the results obtained by a conventional Seasonal Auto-Regressive Integrated Moving Average (SARIMA) model. The R2 scores for UQ-SNN and SARIMA are 0.9994 and 0.9787, respectively, indicating that UQ-SNN is more accurate in capturing the non-linearity and the underlying relationships between the input and output variables. The proposed method can be easily extended to include other input variables to increase the model complexity and is suitable for LTLF. With the available input data, UQ-SNN predicts Malaysia will consume 207.22 TWh of electricity, with standard deviation (SD) of 6.10 TWh by 2030.Keywords
Cite This Article
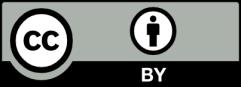