Open Access
ARTICLE
Deep Learning Approach with Optimizatized Hidden-Layers Topology for Short-Term Wind Power Forecasting
1 School of Computer Science, Jiangsu University of Science and Technology, Zhenjiang, 212003, China
2 School of Automation, Key Laboratory of Measurement and Control for CSE, Ministry of Education, Southeast University, Nanjing, 210096, China
* Corresponding Author: Haijian Shao. Email:
Energy Engineering 2020, 117(5), 279-287. https://doi.org/10.32604/EE.2020.011619
Received 20 May 2020; Accepted 13 August 2020; Issue published 07 September 2020
Abstract
Recurrent neural networks (RNNs) as one of the representative deep learning methods, has restricted its generalization ability because of its indigestion hidden-layer information presentation. In order to properly handle of hidden-layer information, directly reduce the risk of over-fitting caused by too many neuron nodes, as well as realize the goal of streamlining the number of hidden layer neurons, and then improve the generalization ability of RNNs, the hidden-layer information of RNNs is precisely analyzed by using the unsupervised clustering methods, such as Kmeans, Kmeans++ and Iterative self-organizing data analysis (Isodata), to divide the similarity of raw data points, and maps the hidden-layer information into the feature space where data separation is easily implemented. Experiments based on dataset from the National Renewable Energy Laboratory (NREL) is proposed to demonstrate the performance of the proposed approaches, the average forecasting errors of which is respectively increased by 2.1%, 7.6%, 10.26% with respect to 6-steps, 12-steps and 18-steps in four seasons over the ones that achieved using the traditional deep learning approaches.Keywords
Cite This Article
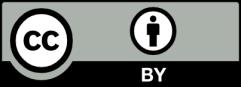
This work is licensed under a Creative Commons Attribution 4.0 International License , which permits unrestricted use, distribution, and reproduction in any medium, provided the original work is properly cited.