Open Access
ARTICLE
Reinforcement Learning Model for Energy System Management to Ensure Energy Efficiency and Comfort in Buildings
1 Department of Heat and Alternative Power Engineering, National Technical University of Ukraine “KPI”, Kyiv, 03056, Ukraine
2 Department of Structural Transformation of the Fuel and Energy Complex, General Energy Institute of NAS of Ukraine, Kyiv, 03150, Ukraine
* Corresponding Author: Oleksandr Novoseltsev. Email:
Energy Engineering 2024, 121(12), 3617-3634. https://doi.org/10.32604/ee.2024.051684
Received 12 March 2024; Accepted 24 April 2024; Issue published 22 November 2024
Abstract
This article focuses on the challenges of modeling energy supply systems for buildings, encompassing both methods and tools for simulating thermal regimes and engineering systems within buildings. Enhancing the comfort of living or working in buildings often necessitates increased consumption of energy and material, such as for thermal upgrades, which consequently incurs additional economic costs. It is crucial to acknowledge that such improvements do not always lead to a decrease in total pollutant emissions, considering emissions across all stages of production and usage of energy and materials aimed at boosting energy efficiency and comfort in buildings. In addition, it explores the methods and mechanisms for modeling the operating modes of electric boilers used to collectively improve energy efficiency and indoor climatic conditions. Using the developed mathematical models, the study examines the dynamic states of building energy supply systems and provides recommendations for improving their efficiency. These dynamic models are executed in software environments such as MATLAB/Simscape and Python, where the component detailing schemes for various types of controllers are demonstrated. Additionally, controllers based on reinforcement learning (RL) displayed more adaptive load level management. These RL-based controllers can lower instantaneous power usage by up to 35%, reduce absolute deviations from a comfortable temperature nearly by half, and cut down energy consumption by approximately 1% while maintaining comfort. When the energy source produces a constant energy amount, the RL-based heat controller more effectively maintains the temperature within the set range, preventing overheating. In conclusion, the introduced energy-dynamic building model and its software implementation offer a versatile tool for researchers, enabling the simulation of various energy supply systems to achieve optimal energy efficiency and indoor climate control in buildings.Graphic Abstract
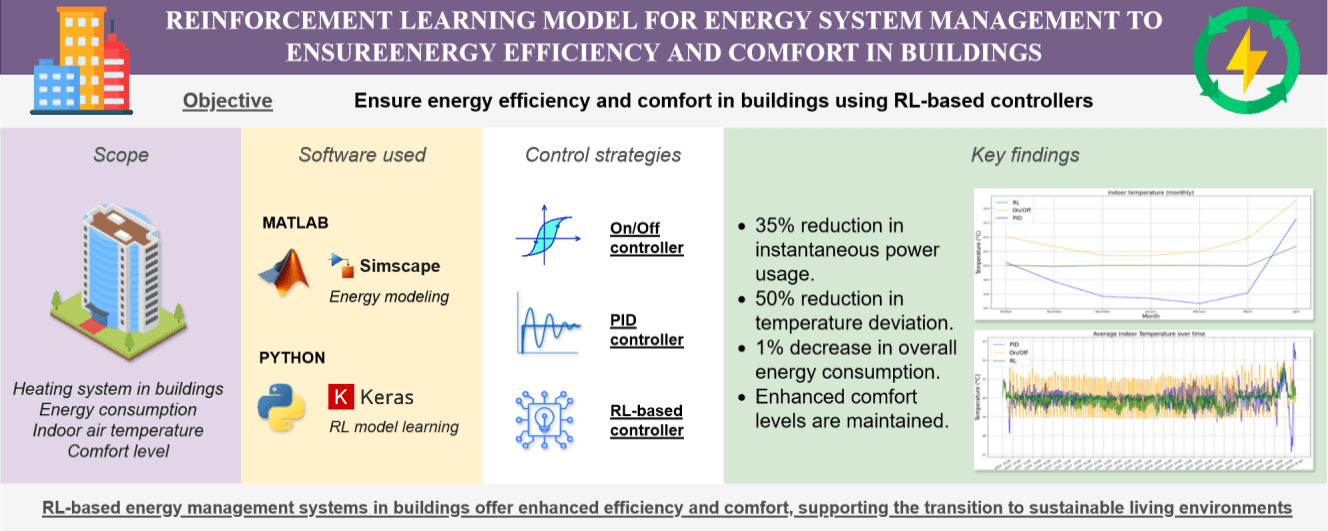
Keywords
Cite This Article
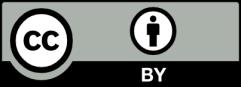
This work is licensed under a Creative Commons Attribution 4.0 International License , which permits unrestricted use, distribution, and reproduction in any medium, provided the original work is properly cited.