Open Access
ARTICLE
Production Capacity Prediction Method of Shale Oil Based on Machine Learning Combination Model
1 Petroleum Engineering Technology Research Institute of Shengli Oilfield, SINOPEC, Dongying, 257000, China
2 Postdoctoral Scientific Research Working Station of Shengli Oilfield, SINOPEC, Dongying, 257000, China
* Corresponding Author: Mingjing Lu. Email:
(This article belongs to the Special Issue: Integrated Geology-Engineering Simulation and Optimizationfor Unconventional Oil and Gas Reservoirs)
Energy Engineering 2024, 121(8), 2167-2190. https://doi.org/10.32604/ee.2024.049430
Received 07 January 2024; Accepted 22 March 2024; Issue published 19 July 2024
Abstract
The production capacity of shale oil reservoirs after hydraulic fracturing is influenced by a complex interplay involving geological characteristics, engineering quality, and well conditions. These relationships, nonlinear in nature, pose challenges for accurate description through physical models. While field data provides insights into real-world effects, its limited volume and quality restrict its utility. Complementing this, numerical simulation models offer effective support. To harness the strengths of both data-driven and model-driven approaches, this study established a shale oil production capacity prediction model based on a machine learning combination model. Leveraging fracturing development data from 236 wells in the field, a data-driven method employing the random forest algorithm is implemented to identify the main controlling factors for different types of shale oil reservoirs. Through the combination model integrating support vector machine (SVM) algorithm and back propagation neural network (BPNN), a model-driven shale oil production capacity prediction model is developed, capable of swiftly responding to shale oil development performance under varying geological, fluid, and well conditions. The results of numerical experiments show that the proposed method demonstrates a notable enhancement in R by 22.5% and 5.8% compared to singular machine learning models like SVM and BPNN, showcasing its superior precision in predicting shale oil production capacity across diverse datasets.Keywords
Cite This Article
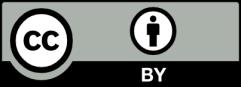