Open Access
ARTICLE
Supervised Learning for Finite Element Analysis of Holes under Biaxial Load
School of Mechanical and Aerospace Engineering, Nanyang Technological University, 639798, Singapore
* Corresponding Author: Wai Tuck Chow. Email:
(This article belongs to the Special Issue: Advances in Methods of Computational Modeling in Engineering Sciences, a Special Issue in Memory of Professor Satya Atluri)
Digital Engineering and Digital Twin 2024, 2, 103-130. https://doi.org/10.32604/dedt.2024.044545
Received 02 August 2023; Accepted 07 March 2024; Issue published 06 May 2024
Abstract
This paper presents a novel approach to using supervised learning with a shallow neural network to increase the efficiency of the finite element analysis of holes under biaxial load. With this approach, the number of elements in the finite element analysis can be reduced while maintaining good accuracy. The neural network will be used to predict the maximum stress for holes of different configurations such as holes in a finite-width plate (2D), multiple holes (2D), staggered holes (2D), and holes in an infinite plate (3D). The predictions are based on their respective coarse mesh with only 2 elements along the whole quarter perimeter. The result shows the prediction errors are under 5% for all the listed hole configurations. In contrast, the conventional FEM with the respective coarse mesh has errors above 20%. To achieve similar accuracy, the conventional FEM would require finer mesh with at least 6 elements along the perimeter. Furthermore, this setup is also effective in predicting the maximum stress for the 3D problem. With the aid of supervised learning, the FEM analysis of a coarse mesh with only 2 elements along the quarter perimeter can attain prediction errors of less than 2%. For the same coarse mesh, the conventional FEM has errors above 35%. To achieve similar accuracy for the 3D problem, the conventional FEM would require finer mesh with more than 8 elements along the perimeter. This result shows that supervised learning has great potential to enhance the efficiency of finite element analysis with fewer elements while attaining satisfactory results.Keywords
Cite This Article
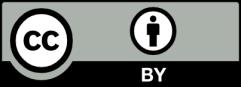
This work is licensed under a Creative Commons Attribution 4.0 International License , which permits unrestricted use, distribution, and reproduction in any medium, provided the original work is properly cited.